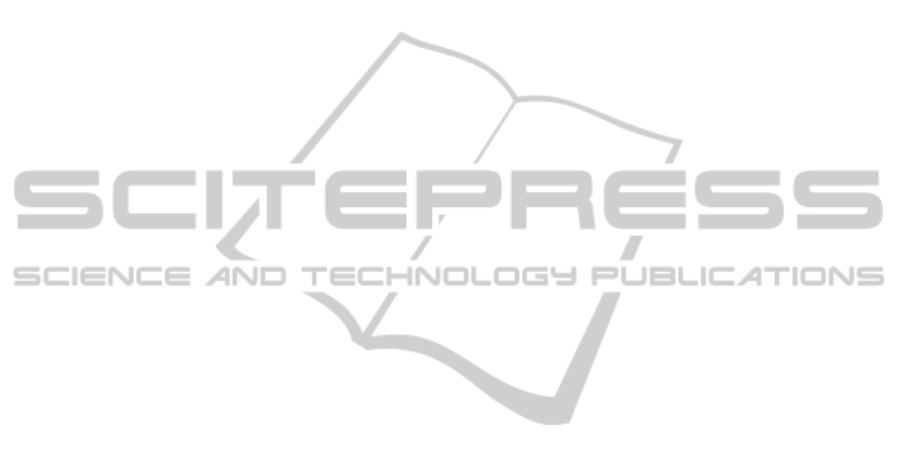
4 CONCLUSION AND FUTURE
WORK
The results of the previous sections show, in a for-
mal way with data mining techniques, that there is
a relationship between the evaluation of the courses
from students and the results they obtained in the cor-
responding examinations. In particular, the analysis
performed on data related to the Computer Science
degree program under examination illustrates that the
courses which received a positive evaluation corre-
spond to exams in which students obtained a good
average mark and that they took with a small delay.
Conversely, the worst evaluations were given to those
courses which do not match good achievements by
students.
The analysis based on clustering with Manhattan
distance allows us to classify courses according to
the assessment received by students and can highlight
some regularities that emerge over the years or points
out some trend reversals due to changes of teachers.
In the Computer Science degree program just consid-
ered, for example, we observe the trend to give not
so good evaluation to Mathematics courses. Results
of this type point out a critical issue in the involved
courses and can be used to implement improvement
strategies.
We wish to emphasize that our analysis refers to
the courses evaluation that students make before tak-
ing the exams and knowing their grades. In fact, as
already observed, the evaluation module is given to
students before the end of the course. Surely, there
is the risk that their judgment is influenced by the
inherent difficulty of the course or by the comments
made by students of the previous years. To this pur-
pose, it is important that during the module compi-
lation the teacher explains that a serious assessment
of the course can increase the quality level of the in-
volved services. Students represent the end-users as
well as the principal actors of the formative services
offered by the University and the measure of their per-
ceived quality is essential for planning changes. How-
ever, the results of courses evaluation should always
be considered in a critical way and should not have the
goal of simplifying the contents to get best ratings.
In general, many other factors should be consid-
ered for evaluating courses and student success, as
addressed in (Romero and Ventura, 2010). The ap-
proach used in this work could be refined and deep-
ened if it was possible to identify the students in-
volved in the courses evaluation in order to connect
properly the results of the evaluation with those of ex-
ams. Moreover, it would be interesting to connect the
assessment of students with other information such
as the gender of students and teachers or the kind of
high school attended by students. Starting from the
academic year 2011/2012, the University of Florence
began to manage on line the evaluation module de-
scribed in Section 2. Therefore, in a next future, it
might be possible to proceed in this direction, tak-
ing into account appropriate strategies to maintain pri-
vacy.
An interesting additional source of information
could be given by social media sites, such as
Face-
book
or
Twitter
, used by students to post comments
about courses and teachers. It would be useful to link
this information with the results of students and their
official evaluations about teachings, in order to take
into account more feedbacks. In such a context, it
might be interesting to use text mining techniques to
classify the student comments and enrich the database
for an analysis similar to that illustrated in this work.
REFERENCES
Progetto SISValDidat. https://valmon.disia.unifi.it/
sisvaldidat/unifi/index.php.
Campagni, R., Merlini, D., and Sprugnoli, R. (2012a).
Analyzing paths in a student database. In The 5th
International Conference on Educational Data
Mining, Chania, Greece, pages 208–209.
Campagni, R., Merlini, D., and Sprugnoli, R.
(2012b). Data mining for a student database. In
ICTCS 2012, 13th Italian Conference on Theo-
retical Computer Science, Varese, Italy.
Campagni, R., Merlini, D., and Sprugnoli, R.
(2012c). Sequential patterns analysis in a student
database. In ECML-PKDD Workshop: Mining
and exploiting interpretable local patterns (I-Pat
2012), Bristol.
Campagni, R., Merlini, D., Sprugnoli, R., and Verri,
M. C. (2013). Comparing examination results
and courses evaluation: a data mining approach.
In Didamatica 2013, Pisa, Area della Ricerca
CNR, AICA, pages 893–902.
Dwork, C. (2008). Differential privacy: a survey of
results. In Theory and Applications of Models
of Computation, 5th International Conference,
TAMC 2008, pages 1–19.
Liao, T. W. (2005). Clustering of time series data: a
survey. Pattern Recognition, 38(11):1857–1874.
MacQueen, J. (1967). Some methods for classifica-
tions and analysis of multivariate observations.
In Proc. of the 5th Berkeley Symp. on Mathemat-
ical Statistics and Probability. University of Cal-
ifornia Press., pages 281–297.
CSEDU2014-6thInternationalConferenceonComputerSupportedEducation
32