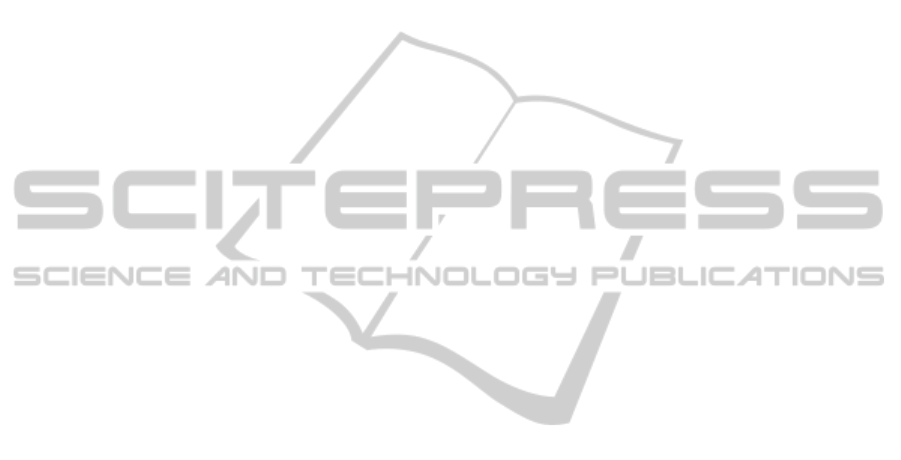
5 CONCLUSIONS
In this paper we discussed the characteristics and
behaviour of three methods frequently used to assess
semantics in various NLP applications: LSA, LDA
and lexical chaining. These methods have been
tested on two different corpora containg different
types of written discouse: a corpus consisting of 4
articles from Wikipedia and another one consisting
of 35 chat conversations with multiple participants
debating four pre-imposed topics: forum, chat, blog
and wikis.
In contrast with the previous studies, we have
compared the outcomes of the three methods using
quantitative scores computed based on the outputs of
each method. These scores included correlations
between similarity scores and the number of
common words from topics and chains. Thus, the
obtained results are task and discourse-independent.
The most important result is that LSA and LDA
have shown the strongest correlation on both
corpora. This is consistent with the theoretical
underpinnings, as LDA is similar to Probabilistic
Latent Semantic Analysis (pLSA), except that the
LDA distribution of topics is assumed to have a
prior Dirichlet distribution. Moreover, LSA scores
might be used to compute the coherence of a LDA
topic as shown in the paper.
Another important contribution is that WordNet-
based lexical chains are not very correlated with
neither LSA nor LDA, therefore they might be seen
as complementary to the LSA or LDA results.
ACKNOWLEDGEMENTS
This research was supported by project No.264207,
ERRIC-Empowering Romanian Research on
Intelligent Information Technologies/FP7-REGPOT-
2010-1.
REFERENCES
Barzilay, R. and Elhadad. M., 1997. Using lexical chains
for text summarization. In: Proceedings of the
Intelligent Scalable Text Summarization Workshop,
pp. 10–17.
Budanitsky, A. and Hirst, G., 2006. Evaluating wordnet-
based measures of semantic relatedness. In:
Computational Linguistics 32 (1), pp. 13–47.
Blei, D. M., Ng, A. Y. and Jordan, M. I., 2003. Latent
Dirichlet allocation. In: Journal of Machine Learning
Research 3, pp. 993-1022.
Boyd-Graber, J., Fellbaum, C., Osherson, D. and Schapire,
R., 2006. Adding dense, weighted, connections to
WordNet. In: Proceedings of the 3rd GlobalWordNet
Meeting, pp. 29–35.
Carthy, J., 2004. Lexical chains versus keywords for topic
tracking. In: Computational Linguistics and Intelligent
Text Processing, LNCS, pp. 507–510. Springer.
Chiru, C., Janca, A., Rebedea, T., 2010. Disambiguation
and Lexical Chains Construction Using WordNet. In
S. Trăuşan-Matu, P.Dessus (Eds.) Natural Language
Processing in Support of Learning: Metrics, Feedback
and Connectivity, MatrixRom, pp 65-71.
Cramer, I., 2008. How well do semantic relatedness
measures perform? a meta-study. In: Proceedings of
the Symposium on Semantics in Systems for Text
Processing.
Griffiths, T. L., Steyvers, M. and Tenenbaum, J. B., 2007.
Topics in semantic representation. In: Psychological
Review, vol. 114, no. 2, pp. 211–244.
Gong, Y. and Liu, X., 2001. Generic Text Summarization
Using Relevance Measure and Latent Semantic
Analysis. In: Proceedings of the 24th ACM SIGIR
conference, pp. 19-25.
Haghighi, A. and Vanderwende, L., 2009. Exploring
content models for multi-document summarization. In:
Proceedings of HLT-NAACL, pp. 362–370.
Halliday, M. A.K. and Hasan, R., 1976. Cohesion In
English, Longman.
Jiang, J. J. and Conrath, D. W, 1997. Semantic similarity
based on corpus statistics and lexical taxonomy. In:
Proceedings of ROCLING X, pp. 19-33.
Kakkonen, T., Myller, N., Sutinen, E.and Timonen, J.,
2008. Comparison of Dimension Reduction Methods
for Automated Essay Grading. In: Educational
Technology & Society, 11(3), pp. 275–288.
Landauer, T. K. and Dumais, S. T., 1997. A solution to
Plato's problem: the Latent Semantic Analysis theory
of acquisition, induction and representation of
knowledge. Psychological Review, 104(2), 211-240.
Misra, H., Yvon, F., Jose, J. and Cappé, O., 2009. Text
Segmentation via Topic Modeling: An Analytical
Study. In: 18th ACM Conference on Information and
Knowledge Management, pp. 1553–1556.
Morris, J. and Hirst, G., 1991. Lexical Cohesion, the
Thesaurus, and the Structure of Text. In:
Computational Linguistics, Vol 17(1), pp. 211-232.
Novischi, A. and Moldovan, D., 2006. Question answering
with lexical chains propagating verb arguments. In:
Proceedings of the 21st International Conference on
CL and 44th Annual Meeting of ACL, pp. 897–904.
Tsatsaronis, G., Varlamis, I. and Vazirgiannis, M., 2010.
Text relatedness based on a word thesaurus. In:
Artificial Intelligence Research, 37, pp. 1–39.
WEBIST2014-InternationalConferenceonWebInformationSystemsandTechnologies
262