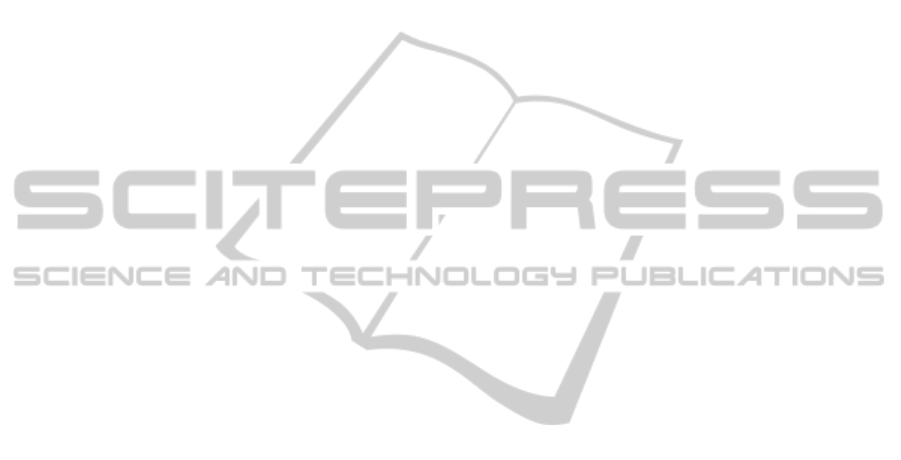
fields materialized view maintenance, integrity check-
ing and view updating, we can hardly evaluate the ac-
curacy of finding other research fields. For this we
would need to evaluate a broader collection of pa-
pers. The detection of research schools, however, was
highly accurate for our collection but an evaluation of
our rules for distinguishing weak and strong reference
types is still to be done. In particular, the trade-off be-
tween the detection of as many different schools as
possible while avoiding the generation of false pos-
itives has still to be investigated. Nevertheless, the
results we have achieved so far already indicate that
the automatic detection of research schools and/or re-
search fields is possible in a feasible way.
8 CONCLUSION
We have presented a system for bibliographical anal-
ysis which is desired by many researchers. Our anal-
ysis results indicate that such a system is able to an-
swer the bibliographical questions which researchers
might encounter while searching for specific papers in
a particular area. Our tool can become a powerful and
influential information system supporting researchers
in other scientific communities to collect, manage, ac-
cess the bibliographical and citation analysis of doc-
uments. The specificity of our system proposal is
the consequent application of a database system with
a full-fledged query language at hand. In this way,
a flexible analysis tool could be programmed which
can be easily extended by new paper related measure-
ments. While the analysis part as well as data acqui-
sition is constantly extended, we additionally plan to
develop a web-based interface for supporting a flexi-
ble querying in the future.
REFERENCES
Aksnes, W. (2005). Citation and Their Use as Indicators in
Science Policy. Study of Validity and Applicability Is-
sues with a Particular Focus on Highly Cited Papers.
PhD thesis, University of Twente.
Bakkalbasi, N., Bauer, K., Glover, J., and Wang, L. (2006).
Three options for citation tracking: Google scholar,
scopus and web of science. BDL, 3(1).
Behrend (2011). A uniform fixpoint approach to the
implementation of inference methods for deductive
databases. In LNAI, pages 1–16.
Chen, C. (2006). Citespace ii: Detecting and visualizing
emerging trends and transient patterns in scientific lit-
erature. JASIST, 57(3):359–377.
Dong and et al. (2005). Reference reconciliation in complex
information spaces. SIGMOD Rec., pages 85–96.
Falagas and et al. (2008). Comparison of pubmed, sco-
pus, web of science, and google scholar: strengths and
weaknesses. FASEB., 22(2):338–342.
Han, H. and et al. (2004). Two supervised learning ap-
proaches for name disambiguation in author citations.
In JCDL, pages 296–305.
Harzing (2013). A preliminary test of google scholar as a
source for citation data: a longitudinal study of nobel
prize winners. Scientometrics., 94(3):1057–1075.
Jacso (2005). As we may search-comparison of major fea-
tures of the web of science, scopus, and google scholar
citation-based and citation-enhanced databases. CUR-
RENT SCIENCE-BANGALORE., 89(9):1537–1547.
Khazaei, H. (2012). Metadata visualization of scholarly
search results: supporting exploration and discovery.
In i-KNOW, pages 1–8.
Klampfl, K. (2013). An unsupervised machine learning ap-
proach to body text and table of contents extraction
from digital scientific articles. In TPDL, pages 144–
155.
Lacasta and et al. (2013). Design and evaluation of a seman-
tic enrichment process for bibliographic databases.
DKE, 88(1):94–107.
Lister, R. and Box, I. (2008). A citation analysis of the
sigcse 2007 proceedings. In SIGCSE, pages 476–480.
Mayol, E. and Teniente, E. (1999). A survey of current
methods for integrity constraint maintenance and view
updating. In ER Workshops, pages 62–73.
Nascimento, M. A., Sander, J., and Pound, J. (2003). Anal-
ysis of sigmod’s co-authorship graph. SIGMOD Rec.,
32(3):8–10.
Newman (2001). The structure of scientific collaboration
networks. In PNAS, pages 401–409.
Rahm, T. (2005). Citation analysis of database publications.
SIGMOD Rec., pages 48–53.
Smeaton, A. F. and et al. (2002). Analysis of papers from
twenty-five years of sigir conferences: What have we
been doing for the last quarter of a century. SIGIR
Forum, 36:39–43.
Tejada and et al. (2002). Learning domain-independent
string transformation weights for high accuracy object
identification. SIGMOD Rec., pages 350–359.
Vicknair and et al. (2010). A comparison of a graph
database and a relational database: a data provenance
perspective. ACMSE., pages 1–6.
Wu and et al. (2008). Interpreting tf-idf term weights as
making relevance decisions. 26(3):1–37.
AFlexibleSystemforaComprehensiveAnalysisofBibliographicalData
151