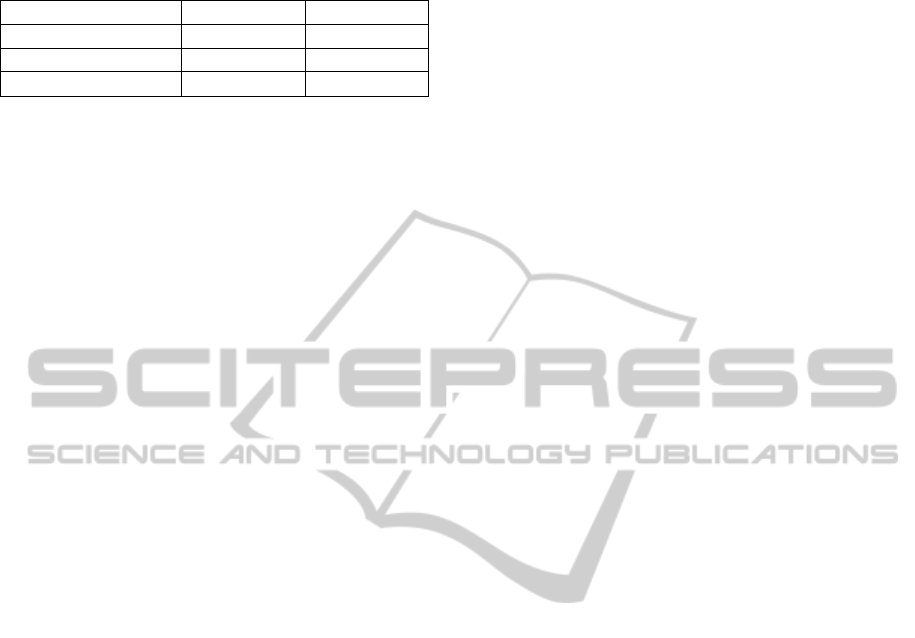
Table 1: Number of students, mean and estimated variance
of each group.
Group 1 Group 2
Number of students n
1
= 15 n
2
= 15
Sample mean
¯
X
1
= 0.5966
¯
X
2
= 0.2284
Estimated variance s
2
1
= 0.0158 s
2
1
= 0.0113
and n
2
=15, thus the degree of freedom is (15 −1) +
(15 −1) = 28. With alpha at 0.05, the two-tailed t
crit
is 2.0484 and we calculated t
obt
= +8.6690. Since
the t
obt
is far beyond the non-reject region defined by
t
crit
= 2.0484, we should reject H
0
and accept H
a
.
As listed in Table 1, the mean rejection rate in
Group 1 was 0.5966 and the mean rejection rate in
Group 2 was 0.2284, and the accepted alternative
hypothesis indicated the difference between the two
means was significant. The analysis suggested that
by using the optimized teaching strategy, the rejection
rate has been reduced from 0.5966 to 0.2284.
7 CONCLUSIONS
In teaching a student, an effective teacher should be
able to adapt a suitable teaching strategy based on
his/her knowledge about the student’s study state, and
should be able to improve his/her teaching when be-
coming more experienced. An effective ITS should
have the same abilities. In our research, we attempt to
build such an ITS. Our approch is POMDP.
Our research has novelty in state definition,
POMDP solving, and online strategy improvement.
The state definition allows important information to
be available locally for choosing the best responses,
and reduces an exponential space into a polynomial
one. Compared with the existing work for applying
RL and POMDP to build ITSs, which mainly depend
on off-line policy improvement, our online improve-
ment algorithm enables the system to continuously
optimize its teaching steategies while it teaches.
ACKNOWLEDGEMENTS
This research is supported by the Natural Sci-
ences and Engineering Research Council of Canada
(NSERC).
REFERENCES
Cassandra, A. (1998) A survey of pomdp applications. In
Working Notes of AAAI 1998 Fall Symposium on Plan-
ning with Partially Observable Markov Decision Pro-
cess, 17-24.
Chinaei, H. R., Chaib-draa, B., Luc Lamontagne, L. (2012).
Learning observation models for dialogue POMDPs.
Canadian AI’12 Proceedings of the 25th Canadian
conference on Advances in Artificial Intelligence ,
280-286, Springer-Verlag Berlin, Heidelberg.
Folsom-Kovarik, J. T., Sukthankar, G., Schatz, S., and
Nicholson, D. (2010) Scalable POMDPs for Diag-
nosis and Planning in Intelligent Tutoring Systems. In
Proceesings of AAAI Fall Symposium on Proactive As-
sistant Agents 2010.
Folsom-Kovarik, J. T., Sukthankar, G., and Schatz, S.
(2013). Tractable POMDP representations for intel-
ligent tutoring systems. ACM Transactions on In-
telligent Systems and Technology (TIST) - Special
section on agent communication, trust in multiagent
systems, intelligent tutoring and coaching systems
archive, 4(2), 29.
Heiman, G. W. (2011). Basic Statistics for the Behavioral
Sciences, Sixth Edition. Wadsworth Cengage Learn-
ing, Belmont, California, USA.
Jurcicek, F., Thomson, B., Keizer, S., Gasic, M., Mairesse,
F., Yu, K., and Young, S., (2010). Natural Belief-
Critic: a reinforcement algorithm for parameter esti-
mation in statistical spoken dialogue systems. Pro-
ceedings of Interspeech10, 90-93, Sept 26-30.
Kaelbling, L. P., Littman, M. L., and Cassandra, A. R.
(1998). Planning and acting in partially observable
stochastic domains. Elsevier Artificial Intelligence,
101: 99–134.
Litman, A. J. and Silliman, S. (2004). Itspoke: an intelligent
tutoring spoken dialogue system. In Proceedings of
Human Language Technology Conference 2004.
Rafferty, A. N., Brunskill, E., Thomas L. Griffiths, T. L.,
and Patrick Shafto, P., (2011). Faster Teaching by
POMDP Planning. In Proceesings of Artificial Intelli-
gence in Education (AIED) 2011), 280-287.
Sutton, R. S. and Barto, A. G. (2005). Reinforcement Learn-
ing: An Introduction. The MIT Press, Cambridge
Massachusetts.
Theocharous, G., Beckwith, R., Butko, N., and Philipose,
M. (2009). Tractable POMDP planning algorithms for
optimal teaching in SPAIS. In IJCAI PAIR Workshop
(2009).
Thomson, B., Jurcicek F., Gai, M., Keizer, S., Mairesse, F.,
Yu, K. and Young, S. (2010). Parameter learning for
POMDP spoken dialogue models. Spoken Language
Technology Workshop (SLT), 2010, 271-276, Berke-
ley, USA.
Williams, J. D., Poupart, P. and Young, S. (2005). Factored
Partially Observable Markov Decision Processes for
Dialogue Management. Proceedings of Knowledge
and Reasoning in Practical Dialogue Systems.
Williams, J. D. and Young, S. (2007). Partially observable
Markov decision processes for spoken dialog systems.
Elsevier Computer Speech and Language, 21, 393-
422.
CSEDU2014-6thInternationalConferenceonComputerSupportedEducation
240