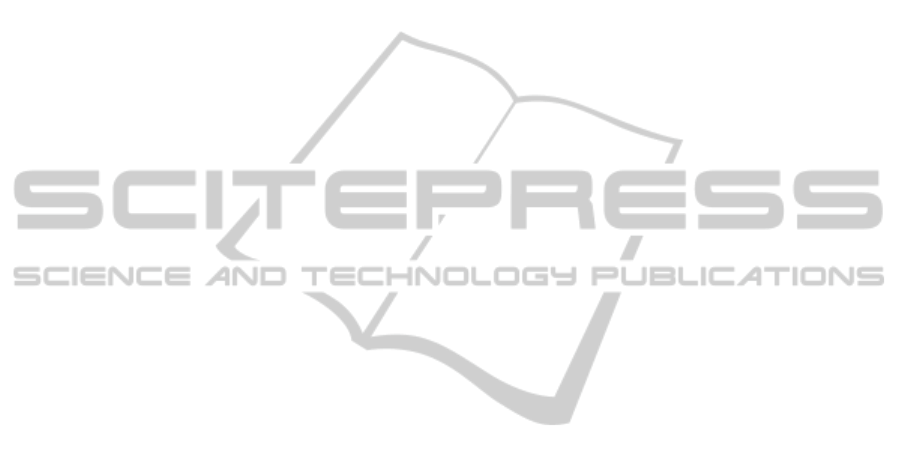
Our approach is similar to spontaneous EEG
modeling efforts that recently emerged and incorpo-
rated head-brain active models in an attempt to ex-
plain spontaneous EEG rhythms. Still, such mod-
els were either hand-tuned and did not incorporate
a joint modeling-estimation modules as proposed
herein, or are computationally intensive. The cur-
rent manuscript advocates simple population-level
model that (a) allow emergent ECT-induced seizures
from normal operation conditions, (b) are biologically
plausible since they derive from neurophysiological
evidence, and (c) are computationally favorable since
they employ generalized cortical population models
and have few fixed parameters (to significantly mod-
ify behavior from normal to epileptic EEG), thereby
making them amenable to estimation.
It also demonstrated the utility of a general nonlin-
ear estimation framework in estimating the connec-
tion strengths based on real ECT seizure data. This
is an integral component to simulate and understand
how seizures propagate among cortical areas under
stimulation. The development of such biologically
plausible models carries the long-term potential of de-
veloping and designing improved ECT-stimulus de-
livery configurations that will (a) retain ECT thera-
peutic efficacy and (b) reduce cognitive side-effects
of associated epilepsies. The preliminary results in-
troduced here focused on the potential role of corti-
cal inhibition particularly, inhibition of inhibition, in
promoting seizures. It is suggested that commonly re-
ported fast activity at seizure onsets can actively con-
tribute to seizure generation (before depolarization
block) by inhibiting dendritic-targeting interneurons
thus disinhibiting local pyramidal cells. Other com-
monly reported global and local players in induced
epileptogenesis are to be addressed in more elabo-
rate models. These include thalamocortical interac-
tions (Demont-Guignard et al., 2012), slow synaptic
mechanisms such as presynaptic GABA-B receptors
activation (Dugladze et al., 2013), accumulation of
Cl
−
within the intracellular pyramidal cell membrane
under excessive stimulation, and possibly astrocyte-
neuron cell interactions.
The estimation framework, an augmented state cu-
bature Kalman filter, was shown to behave reasonably
well on a set of simulated EEG traces intended to ap-
proximate induced seizures. While the augmented
state vector here included only one parameter, our
ongoing work intends to estimate multiple connec-
tivity parameters from real EEG recordings obtained
from ECT-induced seizures and subsequently verify
the connection topology with known propagation pat-
terns of seizures in experimental setup. As mentioned
earlier, the employment of cubature Kalman filtering
for parameter estimation could suffer from lack of ac-
curacy particularly for those parameters that are not
observable at the output. Since our choice of model
starts with an initial parameter set that allows pro-
duces normal EEG behavior, only between-area con-
nectivity was estimated, as output-observable param-
eters, to fit the EEG transition into induced seizures.
ACKNOWLEDGEMENTS
Project supported by the National Council for Scien-
tific Research, Lebanon (LNCSR) and by the AUB
Farouk Jabre Interfaculty Award.
REFERENCES
Arasaratnam, I. and Haykin, S. (2009). Cubature kalman
filters. Automatic Control, IEEE Transactions on,
54(6):1254–1269.
Avermann, M., Tomm, C., Mateo, C., Gerstner, W., and Pe-
terson, C. (2012). Microcircuits of excitatory and in-
hibitory neurons in layer2/3 of mouse barrel cortex.
Journal of Physiology, 107(11):175–191.
Bai, S., Loo, C., Al Abed, A., and Dokos, S. (2012). A com-
putational model of direct brain excitation induced by
electroconvulsive therapy: comparison among three
conventional electrode placements. Brain Stimulation,
5(3):408–421.
Beierlein, M., Gibson, J. R., and Connors, B. W. (2003).
Two dynamically distinct inhibitory networks in layer
4 of the neocortex. Journal of neurophysiology,
90(5):2987–3000.
Cammarota, M., Losi, G., Chiavegato, A., Zonta, M., and
Carmignoto, G. (2013). Fast spiking interneuron con-
trol of seizure propagation in a cortical slice model of
focal epilepsy.
Cruishank, S., Ahmed, O., Stevens, T., Patrick, S., Gonza-
lez, A., Elmaleh, E., and Connors, B. (2012). Thala-
mic control of layer 1 circuits in prefrontal cortex.
De Curtis, M. and Gnatkovsky, V. (2009). Reevaluating
the mechanisms of focal ictogenesis: The role of low-
voltage fast activity. Epilepsia, 50(12):2514–2525.
Demont-Guignard, S., Benquet, P., Gerber, U., Biraben, A.,
Martin, B., and Wendling, F. (2012). Distinct hyperex-
citability mechanisms underlie fast ripples and epilep-
tic spikes. Annals of Neurology, 71:342–352.
Deng, Z.-D., Lisanby, S. H., and Peterchev, A. V. (2011).
Electric field strength and focality in electroconvul-
sive therapy and magnetic seizure therapy: a finite ele-
ment simulation study. Journal of neural engineering,
8(1):016007.
Dugladze, T., Maziashvili, N., B
¨
orgers, C., Gurgenidze, S.,
H
¨
aussler, U., Winkelmann, A., Haas, C. A., Meier,
J. C., Vida, I., Kopell, N. J., et al. (2013). Gabab
autoreceptor-mediated cell type-specific reduction of
ModelingofNeuronalPopulationActivationunderElectroconvulsiveTherapy
237