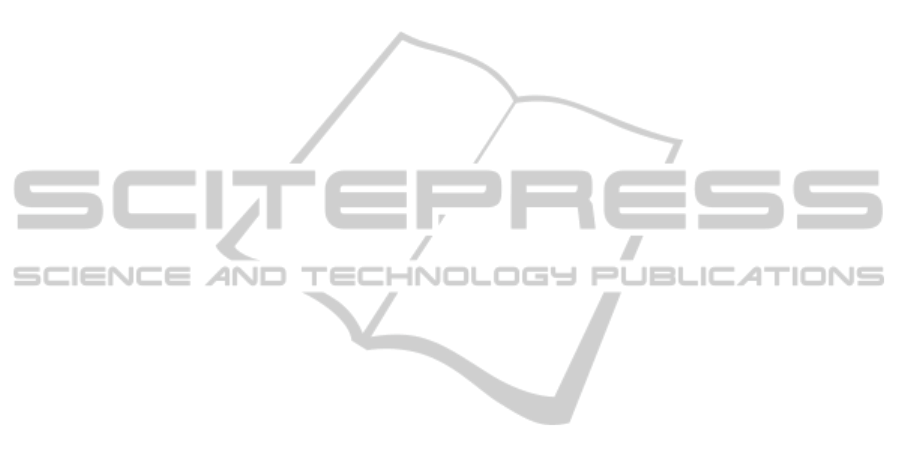
spectral signal representation such as power spec-
tral density techniques, atomic decompositions, time-
frequency energy distributions, continuous and dis-
crete wavelet approaches, and also various classifiers
aiming at distinguishing between right and left hand
motor imageries. They used EEG data from 2 dif-
ferent datasets, from a total of eleven subjects. The
EEG signal was acquired during 8 seconds trials, with
bipolar electrodes over C3 and C4 locations based on
international standard 10/20 system, and band-pass
filtered in the range of 0.5 - 30 Hz. The statistical
analysis was based on five-fold cross validation for
comparisons amongst classifiers. Classification ac-
curacy with Tukeys Honestly Significant Difference
criterion defined the best methods to extract spectral
features, and ANOVA was used to evaluate the vari-
ability among subjects. Using these criterions, the
power spectral density approaches demonstrated the
most consistent robustness and effectiveness in ex-
tracting the discriminant characteristics. With regard
to classification methods, the study has shown the su-
periority of Support Vector Machines with Gaussian
kernel. Furthermore, it was concluded by Herman
et al. (2008) that the combination of different EEG
datasets undertaken by subjects, with varying levels
of prior experience and in motor imagery, enables a
higher inter-subject variance.
Hema et al. (2010) investigated the effect of the
power spectrum feature of each of ten sub-bands of
10 Hz width using motor imagery signals of 10 sec-
onds (s) period recorded from C3 and C4 channels
and a neural classifier aiming at distinguishing among
four tasks. The data signal was segmented into 0.5
s segments with an overlap of 0.25 s, and each seg-
ment was filtered using a Chebyshev band pass filter
with a bandwidth of 10 Hz. The sum of the power
spectrum values was calculated and then a logarithmic
transform was applied. For each subject, ten neural
network models, for each of the ten sub bands of 10
Hz bandwidth, were developed. Classification rates
between 89.23% and 94.47% were achieved for sub-
band frequencies from 21 - 40 Hz.
Fitzgibbon et al. (2004) demonstrated that gamma
band power spectrum corresponding to complex men-
tal tasks had great increase relative to a Control condi-
tion, and the spatial distribution of such increase was
task-related. These studies suggested that higher fre-
quency bands (30 - 100 Hz) may contain useful in-
formation for classification between different mental
states and encouraged Liu et al. (2005) to also investi-
gate the effect of 10 Hz width sub-bands into the clas-
sification of different mental tasks. Differently, Liu
et al. (2005) used EEG signals from C3, C4, P3, P4,
O1 and O2. Seven subjects participated in the exper-
iment and five mental tasks were analyzed, including
no specific mental task, mental multiplication, mental
letter composing, geometric figure rotation and visual
counting. Again, a period of 10 s constituted a trial,
and the sum of weighted power spectrum was used
as feature extracted from ten 10 Hz wide sub-bands,
segmented into 1 s segments with an overlap of 0.9 s.
A Fisher’s Linear Discriminant based classifier was
used to distinguish between the mental tasks. In this
case, frequencies ranging from 30 to 100 Hz resulted
in greater classification accuracy, over 85%.
2 METHODOLOGY
Based on the previously discussed studies, and in
order to validate the proposed approach, EEG sig-
nals were systematically recorded at 1000 Hz using a
Bioamplifier plus PowerLab 16/30 configuration from
AdInstruments, according to the approved protocol
(COEP - USJT - No.088/2011). Three able body sub-
jects were requested to sit comfortably during the ex-
periment in which they had to perform 4 motor im-
ageries (right or left hand close, right or left arm flex).
EEG signals were acquired transversally from F, C, P
and O areas (from Fz, Cz, Pz and, Oz to F3, F4, C3,
C4, P3, P4, O1 and O2 respectively). The commands
were randomly given through the computer monitor
summing 45 repetitions for each movement imagina-
tion. After eliminating those corrupted with unusu-
ally excessive noise, or with simultaneous movement,
only 39 samples were used for each motor imagery
for each subject.
A segment of 2.5 s of each trial was selected and
the Power Spectral Density Averages in ten different
frequency band configurations were computed as fea-
tures. The common part among those arrangements
was: (A) Alpha (8 - 12 Hz), (B1) Beta1 (12 - 16 Hz),
(B2) Beta2 (16 - 20 Hz), (B3) Beta3 (20 - 28 Hz). The
investigation was based on the influence of consider-
ing or not the (D) Delta ( 0 - 4 Hz) and (T) Theta ( 4 -
8 Hz) bands, and five configurations of Gamma band
: (G1) 28 - 32 Hz; (G2) 28 - 64 Hz; (G3) 28 - 100 Hz;
(G4) Gamma1 (28 - 32 Hz), and Gamma2 (32 - 64
Hz); (G5) Gamma1 (28 - 32 Hz), Gamma2 (32 - 64
Hz), and Gamma3 (64 - 100 Hz). The ten treatments
are defined in Table 1. A spatial feature selection,
applying different electrode combinations and aims
to finding the most useful and discriminatory infor-
mation, was also carried out to improve classification
performance.
Fisher’s Linear Discriminant Analysis (Thomaz
and Gillies, 2005) was used as classifier, implement-
ing a few experiments aiming at discriminating be-
ADifferentStatisticalApproachAimingatEEGParameterInvestigationforBrainMachineInterfaceUse
245