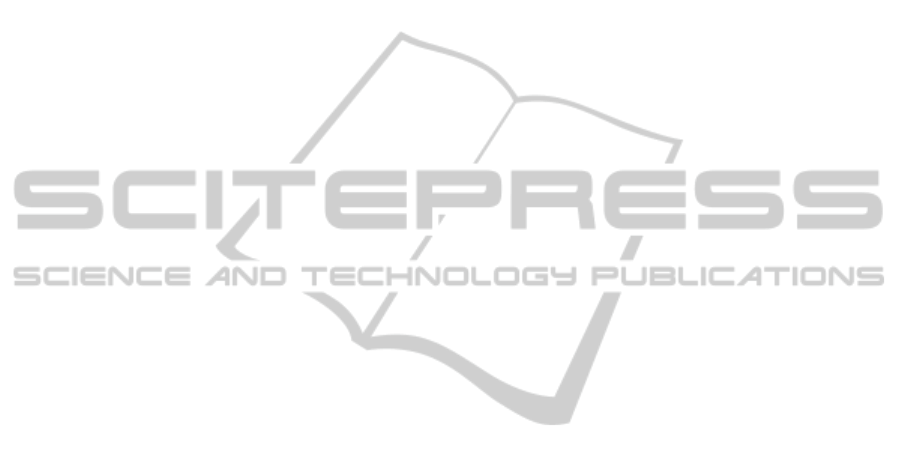
to study. Currently, the authors are working in
this direction.
ACKNOWLEDGEMENTS
This work is partially supported by Erasmus Mundus
Mobility with Asia (EMMA) grant 2012 from the Eu-
ropean Union at the Department of Informatics, Uni-
versity of Evora in Portugal and University with Po-
tential for Excellence (UPE)-Phase II project grant
from University Grants Commission (UGC) in India.
REFERENCES
Bhowmick, S. S., Saha, I., Rato, L., and Bhattacharjee, D.
(2013). RotaSVM: A new ensemble classifier. Ad-
vances in Intelligent Systems and Computing, 227:47–
57.
Breiman, L. (2001). Random forests. Machine Learning,
45(1):5–32.
Brusic, V., Rudy, G., Honeyman, G., Hammer, J., and L,
L. H. (1998). Prediction of MHC class II-binding
peptides using an evolutionary algorithm and artificial
neural network. Bioinformatics, 14:121–130.
Bui, H. H., Sidney, J., Peters, B., Sathiamurthy, M., Sinichi,
A., Purton, K. A., Moth, B. R., Chisari, F. V., Watkins,
D. I., and Sette, A. (2005). Automated generation and
evaluation of specific MHC binding predictive tools:
ARB matrix applications. Immunogenetics, 57:304–
314.
Chang, S. T., Ghosh, D., Kirschner, D. E., and Linder-
man, J. J. (2006). Peptide length-based prediction
of peptide-MHC class II binding. Bioinformatics,
22:2761–2767.
Cover, T. and Hart, P. (1967). Nearest neighbor pattern clas-
sification. IEEE Transactions on Information Theory,
13(1):21–27.
Friedman, M. (1937). The use of ranks to avoid the as-
sumption of normality implicit in the analysis of vari-
ance. Journal of the American Statistical Association,
32:675–701.
Friedman, M. (1940). A comparison of alternative tests of
significance for the problem of m rankings. Annals of
Mathematical Statistics, 11:86–92.
George, H. and Langley, J. P. (1995). Estimating continuous
distributions in bayesian classifiers. in Proceedings of
the Eleventh Conference on Uncertainty in Artificial
Intelligence, 69:338–345.
Greenbaum, J., Sidney, J., Chung, J., Brander, C., Peters,
B., and Sette, A. (2011). Functional classification of
class II human leukocyte antigen (HLA) molecules re-
veals seven different supertypes and a surprising de-
gree of repertoire sharing across supertypes. Immuno-
genetics, 63(6):325–335.
Haque, A. and Blum, J. S. (2005). New insights in anti-
gen processing and epitope selection: development
of novel immunotherapeutic strategies for cancer, au-
toimmunity and infectious diseases. Journal of Bi-
ological Regulators and Homeostatic Agents, 19:93–
104.
Karpenko, O., Shi, J., and Dai, Y. (2005). Prediction of
MHC class II binders using the ant colony search strat-
egy. Artificial Intelligence in Medicine, 35:147–156.
Lauemoller, S. L., Kesmir, C., Corbet, S. L., Fomsgaard, A.,
Holm, A., Claesson, M. H., Brunak, S., and Buus, S.
(2000). Identifying cytotoxic T cell epitopes from ge-
nomic and proteomic information. Rev Immunogenet,
2:447–491.
Maulik, U., Bandyopadhyay, S., and Saha, I. (2010). In-
tegrating clustering and supervised learning for cate-
gorical data analysis. IEEE Transactions on Systems,
Man and Cybernetics Part-A, 40(4):664–675.
Maulik, U. and Saha, I. (2010). Automatic fuzzy clustering
using modified differential evolution for image classi-
fication. IEEE Transactions on Geoscience and Re-
mote Sensing, 48(9):3503–3510.
Moutaftsi, M., Peters, B., Pasquetto, V., Tscharke, D. C.,
Sidney, J., Bui, H. H., Grey, H., and Sette, A. (2006).
A consensus epitope prediction approach identifies the
breadth of murine T(CD8+)-cell responses to vaccinia
virus. Nature Biotechnology, 24:817–819.
Murugan, N. and Dai, Y. (2005). Prediction of MHC class II
binding peptides based on an iterative learning model.
Immunome Research, 1:6.
Nielsen, M., Lundegaard, C., Blicher, T., Lamberth, K.,
Harndahl, M., and et al. (2007). NetMHCpan, a
method for quantitative predictions of peptide bind-
ing to any HLA-A and -B locus protein of known se-
quence. PLoS ONE, 2:e796.
Nielsen, M., Lundegaard, C., Worning, P., Hvid, C. S.,
Lamberth, K., Buus, S., Brunak, S., and Lund, O.
(2004). Improved prediction of MHC class I and class
II epitopes using a novel gibbs sampling approach.
Bioinformatics, 20:1388–1397.
Plewczynski, D., Basu, S., and Saha, I. (2012). AMS 4.0:
consensus prediction of post-translational modifica-
tions in protein sequences. Amino Acid, 43(2):573–
582.
Ramana, J. and Gupta, D. (2010). Machine learning meth-
ods for prediction of CDK-Inhibitors. PLoS ONE,
5(10).
Saha, I., Maulik, U., Bandyopadhyay, S., and Plewczynski,
D. (2011a). Fuzzy clustering of physicochemical and
biochemical properties of amino acids. Amino Acid,
43(2):583–594.
Saha, I., Maulik, U., Bandyopadhyay, S., and Plewczyn-
ski, D. (2011b). Improvement of new automatic dif-
ferential fuzzy clustering using SVM classifier for mi-
croarray analysis. Expert Systems with Applications,
38(12):15122–15133.
Saha, I., Maulik, U., Bandyopadhyay, S., and Plewczynski,
D. (2011c). SVMeFC: SVM ensemble fuzzy clus-
tering for satellite image segmentation. IEEE Geo-
science and Remote Sensing Letters, 9(1):52–55.
BIOINFORMATICS2014-InternationalConferenceonBioinformaticsModels,MethodsandAlgorithms
184