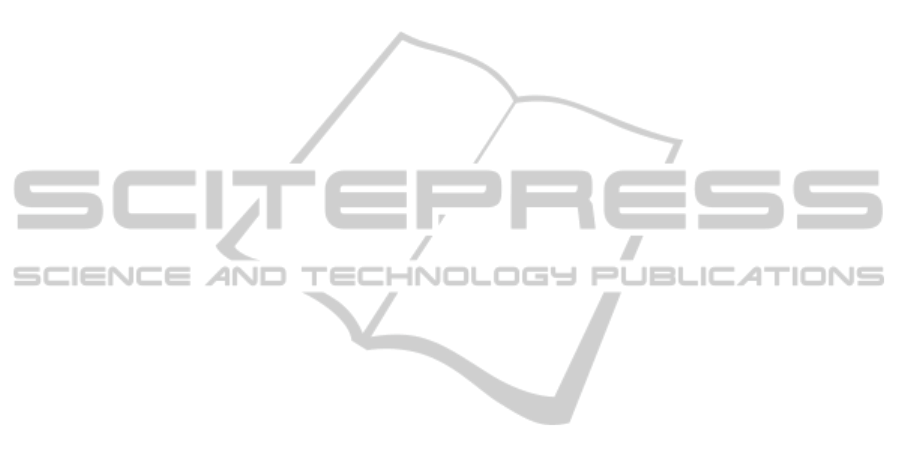
analysis will not be achieved using traditional image
analysis software.
The aim of our study is to develop a simple
analysis technique based on a parametric method
that captures the structural features from the strength
of scattering. Here only one histological subtype of
WDLS and DDLS is described, however they can
represent several patterns (Miettinen, 2003,
Miettinen, 2010) The comparison of the different
histological subtypes and the ability to differentiate
from Normal Fat and Lipoma, benign adipose tissue
is a future study.
5 CONCLUSIONS
Our objective was to study the response of tissue to
a near infrared laser excitation, and, specifically, to
characterize differences between healthy and
cancerous tissue. The morphology of the subsurface
is depicted based on the backscattered near infrared
light. Parametric models of these backscattered
signal characteristics are derived and linked
statistically to the optical properties of Normal Fat,
Well-Differentiated Liposarcoma and De-
Differentiated Liposarcoma.
The accurate diagnosis at early stage of cancer,
as well as the recognition of the tumor boundary in
tissue is highly important. However OCT has been
well-recognized as a powerful method for cancer
detection from tissue morphology, the diagnosis
from these images is subjective and not obvious. We
intend to fill the need for an objective means of data
analysis. The goal of the current study was to
develop a quantitative diagnostic method
differentiating between healthy and cancerous tissue.
The data analysis is developed on images
recorded on human Normal Fat Tissue vs. Well-
differentiated (WD) and De-differentiated
Liposarcoma (DDLS). Further refinement will allow
to detect tumor boundary, diagnose other type of
cancer (e.g. breast cancer) where structural analysis
is required for diagnosis, or to monitor quantitatively
tumor progression during cancer therapy.
As a demonstration of these methods, statistical
analysis was developed to evaluate OCT images of
human fat specimens. An accurate result was found
to quantify healthy vs. cancerous tissue. The analysis
can be applied in real-time for diagnosis, and it is
much simpler comparing to other quantifying
method. This practical advantage gives a good
possibility to use in surgical evaluation.
We describe first time a model-based tissue-
characterization method based on structural
properties of healthy vs. cancerous tissue. Further
statistical validation, sensitivity/specificity analysis
and classification methods have to be performed on
other measurements to prove the efficacy of the
developed method.
ACKNOWLEDGEMENTS
The authors wish to acknowledge the very important
contribution made by Shang Wang and Narendran
Sudheendran. This work was supported by the
Hungarian-American Fulbright Commission, the
French Ministry of Research and the University of
Houston. This work is the continuation of a poster
presentation held on November 16, 2012 at the
MEGA Research day of the University of Houston.
REFERENCES
Bajraszewski, T., Wojtkowski, M., Szkulmowski, M.,
Anna Szkulmowska, Huber, R., Kowalczyk, A., 2008.
Improved spectral optical coherence tomography using
optical frequency comb, Optics Express, 16(6).
Brezinski, M. E., 2006. Optical Coherence Tomography,
Principles and Applications, Academic Press Elsevier
Inc.
Drexler, W. and Fujimoto, J. G., 2008. Optical Coherence
Tomography: Technology and Applications, Springer,
Berlin, New York.
Carbajal, E. F., Baranov, S. A., Manne, V. G. R., Young,
E. D., Lazar, A. J., Lev, D. C., Pollock, R. E., Larin,
K.V., 2011. Revealing retroperitoneal liposarcoma
morphology using optical coherence tomography,
Journal of Biomedical Optics, 16(2).
Fletcher, C. D. M., Rydholm, A., Singer, S., Sundaram,
M., Coindre, J. M., 2006. Soft tissue tumours:
Epidemiology, clinical features, histopathological
typing and grading, WHO Classification of Soft Tissue
Tumors.
Goldberg, B. D., Iftimia, N. V., Bressner, J. E., Pitman, M.
B., Halpern, E., Bouma, B. E., Tearney, G. J., 2008.
Automated algorithm for differentiation of human
breast tissue using low coherence interferometry for
fine needle aspiration biopsy guidance, Journal of
Biomedical Optics, 13(1),
Gossage, K. W., Tkaczyk, T. T., Rodriguez, J. J., Barton,
J. K., 2003. Texture analysis of Optical Coherence
Tomography images: feasibility for tissue
classification, Journal of Biomedical Optics, 8(3).
Gossage, K. W., Smith, C. M., Kanter, E. M., Hariri, L. P.,
Stone, A. L., Rodriguez, J. J., Williams, S. K., Barton,
J. K., 2006. Texture analysis of speckle in Optical
Coherence Tomography images of tissue phantoms,
Physics in Medicine and Biology. 51.
BIOIMAGING2014-InternationalConferenceonBioimaging
26