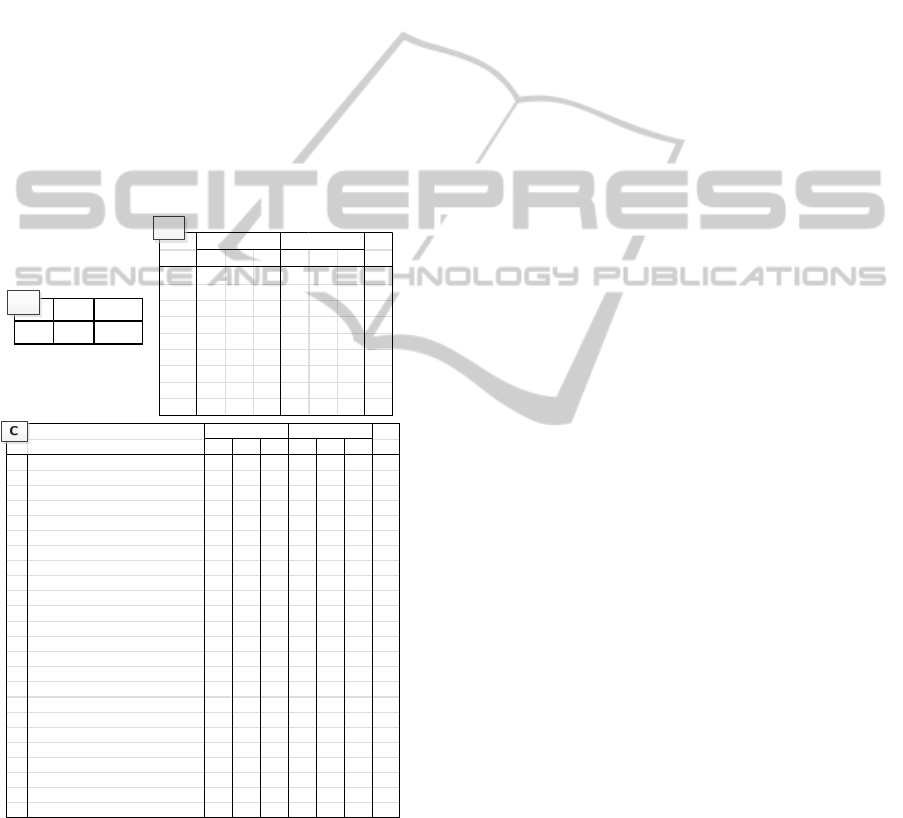
Progress Ratio (OCPRI), at the end of those two
projects, there are three members, O1, E2, and E4
that had a significant increase in terms of acquiring
new competences that might be used in the future,
and consequently, they have more opportunities to
participate in collaborative processes than those who
have a low ratio.
Table 3C illustrates some examples of indicators
to evaluate, for instance, the level of exclusivity of
each competence and the circulation of competences
among members. Based on these data, it is possible
to verify, for example, that according to
Competences Exclusivity Progress Ratio (CEPR),
the highest value belongs to competence C3
(infrared illumination) that had a great proliferation
among members of the network.
Table 3: Indicators for Knowledge production and
circulation analysis.
Start Finish
C 18 24
A
Entity
TOC AOCI OCI TOC AOCI OCI OCPR
O1 4 0,22 0,17 9 0,38 0,27 1,64
E1 2 0,11 0,08 2 0,08 0,06 0,73
E2 1 0,06 0,04 3 0,13 0,09 2,18
E3 1 0,06 0,04 1 0,04 0,03 0,73
E4 3 0,17 0,13 5 0,21 0,15 1,21
E5 1 0,06 0,04 1 0,04 0,03 0,73
E6 5 0,28 0,21 5 0,21 0,15 0,73
E7 3 0,17 0,13 3 0,13 0,09 0,73
O2 4 0,22 0,17 5 0,21 0,15 0,91
Start Finish
B
Competences CA ACEI CEI CA ACEI CEI CEPR
C1 Computervision 2 0,22 0,11 3 0,33 0,09 0,79
C2 SoftwareEngi nee ring 3 0,33 0,17 3 0, 33 0,09 0,53
C3 Infraredillumination 1 0,11 0,06 3 0,33 0,09 1,59
C4 Automaticpatternrecogniti on 3 0,33 0,17 4 0, 44 0,12 0,71
C5 Tollsystems 1 0,11 0,06 1 0, 11 0,03 0,53
C6 InformationSystemsArchi te cture , 1 0,11 0,06 1 0, 11 0,03 0,53
C7 IndustrialDesign 1 0,11 0,06 1 0,11 0,03 0,53
C8 Modellingofproducts 1 0,11 0,06 1 0,11 0,03 0,53
C9 Rapidprototyping 1 0,11 0,06 1 0, 11 0,03 0,53
C10 Developmentofmolds 1 0,11 0,06 1 0, 11 0,03 0,53
C11 Plasticinje ction 1 0,11 0,06 1 0, 11 0,03 0,53
C12 FunctionalTe sts 1 0,11 0,06 1 0, 11 0,03 0,53
C13 SoftwareDeve lopment 1 0,11 0,06 1 0, 11 0,03 0,53
C14 SoftwareArchitecture 1 0,11 0,06 1 0,11 0,03 0,53
C15 ProjectManagement 2 0,22 0,11 2 0,22 0,06 0,53
C16 FunctionalAnalysis 1 0,11 0,06 1 0,11 0,03 0,53
C17 Remotemonitoring 1 0,11 0,06 1 0,11 0,03 0,53
C18 Supplierofequipmentforimagecapture 1 0,11 0,06 1 0, 11 0,03 0,53
C19 ElectronicTollCollection(ETC)systems 0 0,00 0,00 1 0, 11 0,03 ‐‐‐‐
C20 InformationSystemsopentomulti‐vendor 0 0,00 0,00 1 0,11 0,03 ‐‐‐‐
c21 Automati cvehicleidentificationsyste ms 0 0,00 0, 00 1 0,11 0,03 ‐‐‐
C22 Communicationsystemsbetweenvehicles 0 0,00 0,00 1 0,11 0,03 ‐‐‐
C23 Classificationsystemsofvehicles 0 0,00 0,00 1 0,11 0,03 ‐‐‐‐
C24 Shortrunproduction 0 0,00 0,00 1 0,11 0,03 ‐‐‐
FinishStart
5 CONCLUSIONS
Reaching a better characterization and understanding
of the mechanisms of production and circulation of
knowledge in collaborative networks is an important
element for a better understanding of the behavioral
aspects, and also to improve the sustainability of this
organizational form.
The development of a set of indicators to capture
and measure the circulation and production of
knowledge can be a useful instrument to the
manager of this network, as a way to support the
promotion of collaborative behaviors, and for a
member as a way to extract the advantages of
belonging to a network. Using simple calculations as
illustrated above, it is possible to extract some
indicators. Some preliminary steps in this direction
were presented. However, the development of
indicators to measure the potential impacts and
worth related to production and circulation of
knowledge, for instance, at a member level, in terms
of capacity of generating new ideas, development of
new processes, new products or services,
organizational improvement through the
combination of the existent resources and diversity
of cultures and experiences of other enterprises is
not yet well understood and requires further research
and development.
ACKNOWLEDGEMENTS
This work was partially supported by BRISA Innovation
and Technology (BIT) through a research and
development project.
REFERENCES
Abreu, A. and L. M. Camarinha-Matos, 2010.
Understanding Social Capital in Collaborative
Networks, in
Balanced Automation Systems for Future
Manufacturing Networks
, Á.O. Bas., R.D. Franco.,
and P.G. Gasquet, (Eds.), Boston, Springer. pp. 109 -
118.
Abreu, A., P. Macedo, et al., 2008. Towards a
methodology to measure the alignment of value
systems in collaborative networks.
Innovation in
Manufacturing Network
. A. Azevedo (Eds.) Boston,
Springer, pp. 37- 46.
Argote, L., et al., 2000. Knowledge Transfer in
Organizations: Learning from the Experience of
Others.
Organizational Behavior and Human Decision
Processes,
82(1).
Bell, D., 1974.
The coming of postindustrial society,
Harmondsworth, Penguin.
Camarinha-Matos, L. M., P. Macedo, et al., 2008.
Analysis of core-values alignment in collaborative
networks.
Pervasive Collaborative Networks. L. M.
Camarinha-Matos and W. Picard (Eds.), Boston,
Springer, pp.53 - 64.
Castells, M., 2005. A era da informação: economia,
ICORES2014-InternationalConferenceonOperationsResearchandEnterpriseSystems
188