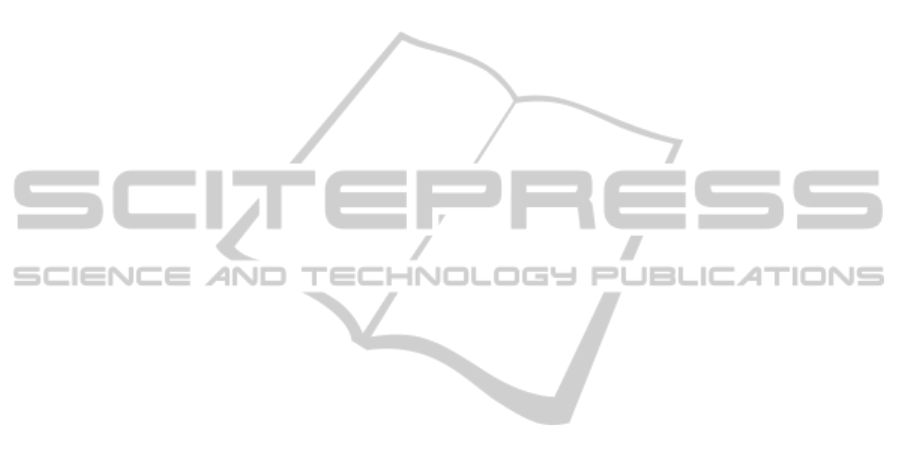
for an agent to reason counterfactually in worlds that
haveplausibility starting from the ordinal ω. Consider
a statement of the form “The present king of France
is bald.” We can reasonably expect an agent to revise
their beliefs about the present king of France, even if
they do not believe in his existence. For example, one
might be told “All french monarchs just had a hair
transplant.” In this case, it could be counterfactually
concluded that the present king of France is no longer
bald. This kind of reasoning can be modelled by iden-
tifying each limit ordinal (such as ω) with a hypothet-
ical world configuration. In this manner, ordinals of
the form ω + i can then be used as plausibility val-
ues to represent events of varying degrees of implau-
sibility within these hypothetical worlds. The order-
ing on limit ordinals indicates which hypetheticals are
the most outlandish, but we are able to perform revi-
sion across each in a uniform manner. We leave this
application of plausibility functions for future work.
6 CONCLUSIONS
In this paper, we have discussed the use of plausiblity
functions for reasoning about belief change, with a
particular focus on action domains. We have demon-
strated that Spohn-style ranking functions can be used
to define an algebra of belief change, which can then
be extended to reason about belief progression due
to actions. We then used the same kind of rank-
ing functions to represent uncertainty over the actions
that have been executed. Through commonsense ex-
amples we demonstrated that ranking functions are a
flexible tool that can capture many different kinds of
uncertainty. At a formal level, it has long been known
that AGM revision can be defined in terms of rank-
ing functions or total pre-orders; but there is a sense
in which pre-orders are simpler, and they have tended
to be more popular in the literature. Our examples
give many situations that are easier to represent with
ranking functions, because the gap between different
levels of plausibility is important. In many cases, our
uniform treatment of belief states and observations
simplifies the algebra of belief change.
REFERENCES
Alchourr´on, C., G¨ardenfors, P., and Makinson, D. (1985).
On the logic of theory change: Partial meet func-
tions for contraction and revision. Journal of Symbolic
Logic, 50(2):510–530.
Baltag, A., Moss, L., and Solecki, S. (1998). The logic of
public announcements, common knowledge and pri-
vate suspicions. In Proceedings of the 7th Conference
on Theoretical Aspects of Rationality and Knowledge
(TARK-98), pages 43–56.
Boutilier, C. (1995). Generalized update: Belief change in
dynamic settings. In Proceedings of the Fourteenth In-
ternational Joint Conference on Artificial Intelligence
(IJCAI 1995), pages 1550–1556.
Delgrande, J., Dubois, D., and Lang, J.(2006). Iterated revi-
sion as prioritized merging. In Proceedings of the 10th
International Conference on Principles of Knowledge
Representation and Reasoning (KR2006).
Delgrande, J. and Levesque, H. (2012). Belief revision with
sensing and fallible actions. In Proceedings of the In-
ternational Conference on Knowledge Representation
and Reasoning (KR2012).
Dubois, D. and Prade, H. (2004). Possibilistic logic: a ret-
rospective and prospective view. Fuzzy Sets and Sys-
tems, 144(1):3–23.
Hunter, A. and Delgrande, J. (2006). Belief change in the
context of fallible actions and observations. In Pro-
ceedings of the National Conference on Artificial In-
telligence(AAAI06).
Hunter, A. and Delgrande, J. (2011). Iterated belief change
due to actions and observations. Journal of Artificial
Intelligence Research, 40:269–304.
Katsuno, H. and Mendelzon, A. (1991). On the difference
between updating a knowledge base and revising it. In
Proceedings of the Second International Conference
on Principles of Knowledge Representation and Rea-
soning (KR 1991), pages 387–394.
Katsuno, H. and Mendelzon, A. (1992). Propositional
knowledge base revision and minimal change. Arti-
ficial Intelligence, 52(2):263–294.
Lang, J. (2007). Belief update revisited. In Proceedings of
the International Joint Conference on Artificial Intel-
ligence (IJCAI07), pages 2517–2522.
Levesque, H., Pirri, F., and Reiter, R. (1998). Foundations
for the situation calculus. Link¨oping Electronic Arti-
cles in Computer and Information Science, 3(18):1–
18.
Lorini, E. (2011). A dynamic logic of knowledge, graded
beliefs and graded goals and its application to emotion
modelling. In LORI, pages 165–178.
Shapiro, S., Pagnucco, M., Lesperance, Y., and Levesque,
H. (2011). Iterated belief change in the situation cal-
culus. Artificial Intelligence, 175(1):165–192.
Spohn, W. (1988). Ordinal conditional functions. A dy-
namic theory of epistemic states. In Harper, W. and
Skyrms, B., editors, Causation in Decision, Belief
Change, and Statistics, vol. II, pages 105–134. Kluwer
Academic Publishers.
Stalnaker, R. (2009). Iterated belief revision. Erkenntnis,
70(1-2):189–209.
van Ditmarsch, H., van der Hoek, W., and Kooi, B. (2007).
Dynamic Epistemic Logic. Springer.
RankingFunctionsforBeliefChange-AUniformApproachtoBeliefRevisionandBeliefProgression
419