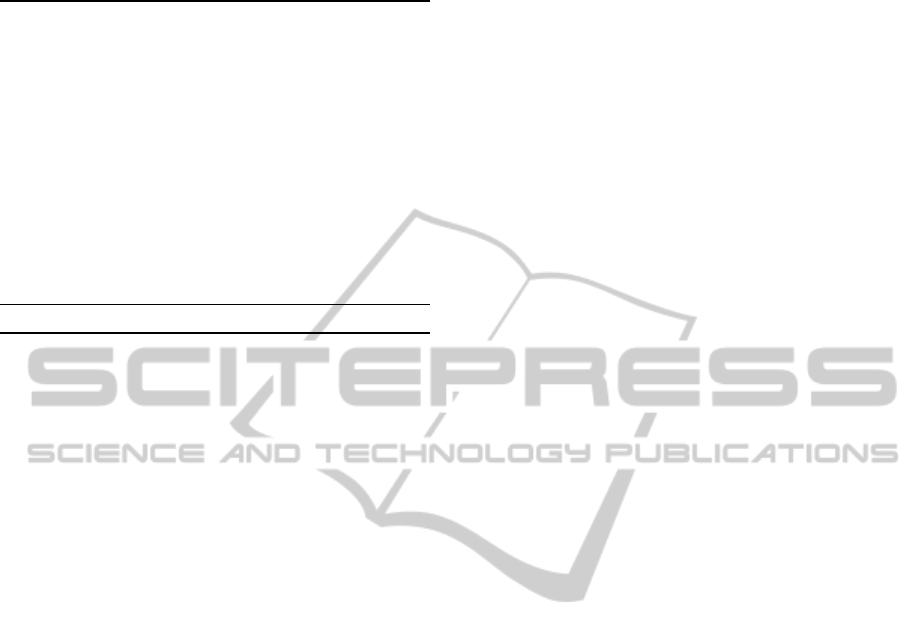
Table 2: Classification accuracy (%).
simple full-connected proposed
road 93.10 93.80 94.92
building 75.90 72.96 78.70
sky 90.52 82.21 90.25
tree 70.49 77.59 79.95
sidewalk 77.06 78.43 81.36
car 53.84 58.64 65.16
column pole 9.53 16.15 12.85
sign symbol 1.73 1.62 1.70
fence 5.23 11.09 13.48
pedestrian 17.26 30.69 31.52
bicyclist 17.09 18.49 24.88
avg. 46.52 49.24 52.25
4 CONCLUSIONS
We have proposed a method to discriminatively learn
the prior biases in the classification. In the proposed
method, for improving the classification performance,
all samples are utilized to train the classifier and the
input sample is adequately classified based on the
prior information via the learnt biases. The proposed
method is formulated in the maximum-margin frame-
work, resulting in the optimization problem of the QP
form similarly to SVM. We also presented the compu-
tationally efficient approach to optimize the resultant
QP along the line of SMO. The experimental results
on the patch labeling in the on-board camera images
demonstrated that the proposed method is superior in
terms of classification accuracy and the computation
cost. In particular, the proposed classifier operates
as fast as the standard (linear) classifier, and besides
the computation time for training the classifier is even
faster than the SVM of the same size.
REFERENCES
Bishop, C. M. (1995). Neural Networks for Pattern Recog-
nition. Oxford University Press, New York, NY.
Bishop, C. M. (2006). Pattern Recognition and Machine
Learning. Springer, Berlin, Germany.
Brostow, G. J., Shotton, J., Fauqueur, J., and Cipolla, R.
(2008). Segmentation and recognition using structure
from motion point clouds. In ECCV’08, the 10th Eu-
ropean Conference on Computer Vision, pages 44–57.
Chang, C.-C. and Lin, C.-J. (2001). LIBSVM: a library
for support vector machines. Software available at
http://www.csie.ntu.edu.tw/ cjlin/libsvm.
Cremers, D. and Grady, L. (2006). Statistical priors for ef-
ficient combinatorial optimization via graph cuts. In
ECCV’06, the 9th European Conference on Computer
Vision, pages 263–274.
El-Baz, A. and Gimel’farb, G. (2009). Robust image seg-
mentation using learned priors. In ICCV’09, the 12nd
International Conference on Computer Vision, pages
857–864.
Fan, R.-E., Chen, P.-H., and Lin, C.-J. (2005). Working set
selection using second order information for training
support vector machines. Journal of Machine Learn-
ing Research, 6:1889–1918.
Gao, T., Stark, M., and Koller, D. (2012). What makes a
good detector? - structured priors for learning from
few examples. In ECCV’12, the 12th International
Conference on Computer Vision, pages 354–367.
Ghosh, J. and Ramamoorthi, R. (2003). Bayesian Nonpara-
metrics. Springer, Berlin, Germany.
Jiang, T., Jurie, F., and Schmid, C. (2009). Learning shape
prior models for object matching. In CVPR’09, the
22nd IEEE Conference on Computer Vision and Pat-
tern Recognition, pages 848–855.
Jie, L., Tommasi, T., and Caputo, B. (2011). Multi-
class transfer learning from unconstrained priors. In
ICCV’11, the 13th International Conference on Com-
puter Vision, pages 1863–1870.
Joachims, T. (1999). Making large-scale svm learning prac-
tical. In Sch¨olkopf, B., Burges, C., and Smola, A.,
editors, Advances in Kernel Methods - Support Vec-
tor Learning, pages 169–184. MIT Press, Cambridge,
MA, USA.
Kan, M., Shan, S., Zhang, H., Lao, S., and Chen, X. (2012).
Multi-view discriminant analysis. In ECCV’12, the
12th International Conference on Computer Vision,
pages 808–821.
Kapoor, A., Hua, G., Akbarzadeh, A., and Baker, S. (2009).
Which faces to tag: Adding prior constraints into ac-
tive learning. In ICCV’09, the 12nd International
Conference on Computer Vision, pages 1058–1065.
Kobayashi, T. and Otsu, N. (2008). Image feature extraction
using gradient local auto-correlations. In ECCV’08,
the 10th European Conference on Computer Vision,
pages 346–358.
Platt, J. (1999). Fast training of support vector machines
using sequential minimal optimization. In Sch¨olkopf,
B., Burges, C., and Smola, A., editors, Advances
in Kernel Methods - Support Vector Learning, pages
185–208. MIT Press, Cambridge, MA, USA.
Poggio, T., Mukherjee, S., Rifkin, R., Rakhlin, A., and
Verri, A. (2001). b. Technical Report CBCL Paper
#198/AI Memo #2001-011, Massachusetts Institute of
Technology, Cambridge, MA, USA.
Sharma, A. and Jacobs, D. (2011). Bypassing synthesis:
Pls for face recognition with pose, low-resolution and
sketch. In CVPR’11, the 24th IEEE Conference on
Computer Vision and Pattern Recognition (CVPR),
pages 593–600.
Smola, A. J., Bartlett, P., Sch¨olkopf, B., and Schuurmans,
D. (2000). Advances in Large-Margin Classifiers.
MIT Press, Cambridge, MA, USA.
ICPRAM2014-InternationalConferenceonPatternRecognitionApplicationsandMethods
74