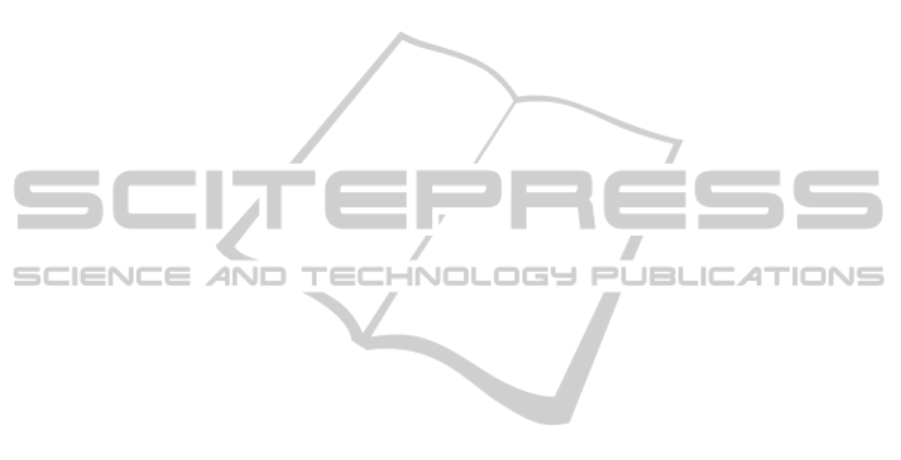
ACKNOWLEDGEMENTS
This work has been made within the research project
SPACE, which is an interdisciplinary research project
between the University Luxembourg, Department of
Computer Science and SES Engineering. We thank
all the SPACE members as well as all the SES en-
gineers for their kind support. The views expressed
herein represent the authors’ views only and do not in
any way bind or commit SES Engineering itself.
REFERENCES
Azevedo, D. N. R. and Ambr
´
osio, A. M. Dependability in
satellite systems: An architecture for satellite teleme-
try analysis.
Beringer, J. and H
¨
ullermeier, E. (2006). Online clustering
of parallel data streams. Data & Knowledge Engineer-
ing, 58(2):180–204.
Bouleau, F. and Schommer, C. (2013). Outlier identifica-
tion in spacecraft monitoring data using curve fitting
information. In European Conference on Data Analy-
sis 2013, page 158.
Das, K., Bhaduri, K., and Votava, P. (2011). Distributed
anomaly detection using 1-class svm for vertically
partitioned data. Statistical Analysis and Data Min-
ing, 4(4):393–406.
Deng, K., Moore, A., and Nechyba, M. (1997). Learning to
recognize time series: Combining arma models with
memory-based learning. In IEEE Int. Symp. on Com-
putational Intelligence in Robotics and Automation,
volume 1, pages 246 – 250.
Fujimaki, R., Yairi, T., and Machida, K. (2005). An
anomaly detection method for spacecraft using rele-
vance vector learning. In Ho, T., Cheung, D., and
Liu, H., editors, Advances in Knowledge Discovery
and Data Mining, volume 3518 of Lecture Notes in
Computer Science, pages 785–790.
Fukushima, Y. Telemetry data mining with svm for satellite
monitoring.
Isaksson, C. and Dunham, M. H. (2009). A comparative
study of outlier detection algorithms. In Machine
Learning and Data Mining in Pattern Recognition,
pages 440–453. Springer.
Keogh, E. (2004). T5: Data mining and machine learning
in time series databases.
Keogh, E., Chakrabarti, K., Pazzani, M., and Mehrotra, S.
(2001). Locally adaptive dimensionality reduction for
indexing large time series databases. In Proceedings
of the 2001 ACM SIGMOD international conference
on Management of data, SIGMOD ’01, pages 151–
162, New York, NY, USA. ACM.
Keogh, E., Lonardi, S., and chi’ Chiu, B. Y. (2002). Finding
surprising patterns in a time series database in linear
time and space. In In In proc. of the 8th ACM SIGKDD
International Conference on Knowledge Discovery
and Data Mining, pages 550–556. ACM Press.
Last, M., Kandel, A., and Bunke, H. (2004). Data mining
in time series databases. World scientific Singapore.
L
´
etourneau, S., Famili, F., and Matwin, S. (1999). Data
mining for prediction of aircraft component replace-
ment. Special Issue on Data Mining.
Li, Q., Zhou, X., Lin, P., and Li, S. (2010). Anomaly de-
tection and fault diagnosis technology of spacecraft
based on telemetry-mining. In Systems and Control
in Aeronautics and Astronautics (ISSCAA), 2010 3rd
International Symposium on, pages 233–236.
Lin, J., Keogh, E., Lonardi, S., and Chiu, B. (2003). A sym-
bolic representation of time series, with implications
for streaming algorithms. In Proceedings of the 8th
ACM SIGMOD workshop on Research issues in data
mining and knowledge discovery, DMKD ’03, pages
2–11, New York, NY, USA. ACM.
Mart
´
ınez-Heras, J.-A., Donati, A., Sousa, B., and Fischer, J.
(2012). Drmust–a data mining approach for anomaly
investigation.
Rebbapragada, U., Protopapas, P., Brodley, C. E., and Al-
cock, C. (2009). Finding anomalous periodic time se-
ries. Machine learning, 74(3):281–313.
Saleh, J. and Castet, J. (2011). Spacecraft Reliability and
Multi-State Failures: A Statistical Approach. John
Wiley & Sons.
Yairi, T., Kawahara, Y., Fujimaki, R., Sato, Y., and
Machida, K. (2006). Telemetry-mining: A machine
learning approach to anomaly detection and fault di-
agnosis for space systems. In Proceedings of the
2nd IEEE International Conference on Space Mission
Challenges for Information Technology, SMC-IT ’06,
pages 466–476, Washington, DC, USA. IEEE Com-
puter Society.
ICAART2014-InternationalConferenceonAgentsandArtificialIntelligence
120