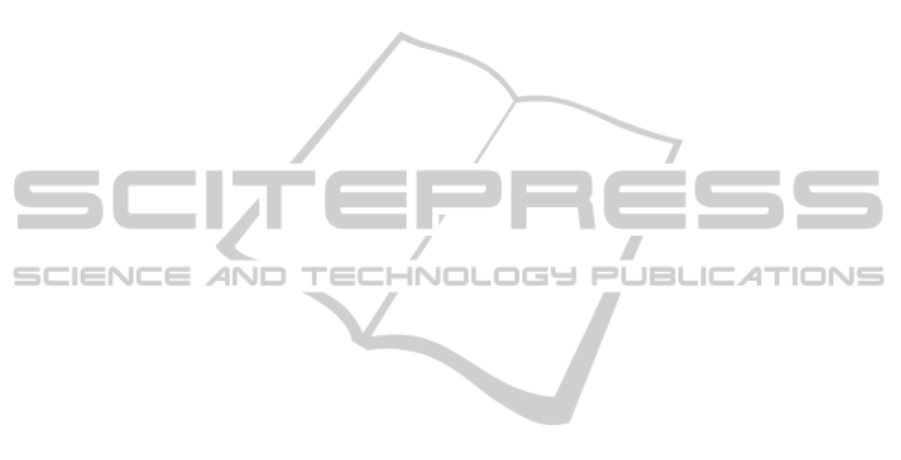
heterogeneous modeling paradigm and to implement
and compare different driver models in highway traf-
fic. We have evaluated our framework from two per-
spectives: (i) Its ability to meet this goal, (ii) the scala-
bility of the framework. Our evaluation demonstrates
that the our framework is quite flexible in modeling
complex heterogeneous driving behavior. The main
advantage of our framework is its ability to mix mul-
tiple driver models rather than using a single model
for an entire population of drivers as is done in cur-
rent traffic simulation frameworks. A second advan-
tage of our proposed framework is that in compari-
son with solutions like micro-simulation with reactive
agents, the framework’s use of BDI agents makes it
better suited for modeling the cognitive complexities
of driving behavior. While less scalable than reac-
tive agents, our BDI approach easily scales to 100s
of agents which makes them more scalable than other
cognitive frameworks such as SOAR and ACT-R. In
terms of scalability the framework shows promising
results towards our requirements. The scalability of
agents and their improvement remains an important
avenue of research.
REFERENCES
Barcel
´
o, J., editor (2010). Fundamentals of Traffic Simula-
tion, volume 145 of Operations Research & Manage-
ment Science. Springer.
Bartish, A. and Thevathayan, C. (2002). BDI agents for
game development. In Proc. AAMAS ’02, part 2, page
668. ACM.
Bazzan, A. L. C., Wahle, J., and Kl
¨
ugl, F. (1999). Agents in
traffic modelling - from reactive to social behaviour.
KI-99: Advances in Artificial Intelligence, pages 303–
306.
Behrens, T. M., Hindriks, K. V., and Dix, J. (2010). To-
wards an environment interface standard for agent
platforms. Annals of Mathematics and Artificial In-
telligence, 61(4):261–295.
Cheng, H. H. (2010). A Review of the Applications of
Agent Technology in Traffic and Transportation Sys-
tems. IEEE Transactions on Intelligent Transporta-
tion Systems, 11(2):485–497.
Dekker, M., Hameete, P., and Hegemans, M. (2012). Hac-
tarV2: an agent team strategy based on implicit coor-
dination. Programming Multi-Agent Systems , LNCS,
7217:173–184.
Ehlert, P. and Rothkrantz, L. (2001). Microscopic traffic
simulation with reactive driving agents. In ITSC. 2001
IEEE Intelligent Transportation Systems., pages 860–
865. IEEE.
Hidas, P. (2002). Modelling lane changing and merging
in microscopic traffic simulation. Transportation Re-
search Part C: Emerging Technologies, 10(5-6):351–
371.
Hindriks, K. V., de Boer, F. S., van der Hoek, W., and
Meyer, J.-J. C. (2001). Agent programming with
declarative goals. Intelligent Agents VII Agent The-
ories Architectures and Languages, LNCS, 1986:228–
243.
Hindriks, K. V., van Riemsdijk, B., Behrens, T., Korstanje,
R., Kraayenbrink, N., Pasman, W., and de Rijk, L.
(2011). Unreal goal bots. Agents for Games and Sim-
ulations II, LNCS, 6525:1–18.
Hoogendoorn, R., Hoogendoorn, S. P., Brookhuis, K., and
Daamen, W. (2010). Mental Workload, Longitudi-
nal Driving Behavior, and Adequacy of Car-Following
Models ... Transportation Research Record, 2188:64–
73.
Kesting, A., Treiber, M., and Helbing, D. (2007).
General Lane-Changing Model MOBIL for Car-
Following Models. Transportation Research Record,
1999(1):86–94.
Krajzewicz, D. and Hertkorn, G. (2002). Sumo (simulation
of urban mobility). Proc. of the 4th Middle East Sym-
posium on Simulation and Modelling, pages 183—-
187.
Langley, P., Laird, J. E., and Rogers, S. (2009). Cognitive
architectures: Research issues and challenges. Cogni-
tive Systems Research, 10(2):141–160.
Lee, L., Nwana, H., Ndumu, D., and Wilde, P. D. (1998).
The stability, scalability and performance of multi-
agent systems. BT Technology Journal, 16(3):94–103.
Nagel, K. and Rickert, M. (2001). Parallel implementation
of the TRANSIMS micro-simulation. Parallel Com-
puting, 27(12):1611–1639.
Navarro, L., Flacher, F., and Corruble, V. (2011). Dynamic
level of detail for large scale agent-based urban simu-
lations. Proc. AAMAS, 2:701–708.
Ossen, S. and Hoogendoorn, S. P. (2011). Heterogeneity in
car-following behavior: Theory and empirics. Trans-
portation Research Part C: Emerging Technologies,
19(2):182–195.
Pokahr, A., Braubach, L., and Lamersdorf, W. (2005).
Jadex: A BDI reasoning engine. Multi-Agent Pro-
gramming, pages 149–174.
Rindsf
¨
user, G. (2005). Multi Agent System Simulation for
the Generation of Individual Activity Programs. Ap-
plications of Agent Technology in Traffic and Trans-
portation, pages 165–180.
Rossetti, R. J., Bordini, R. H., Bazzan, A. L., Bampi, S.,
Liu, R., and Vliet, D. V. (2002). Using BDI agents
to improve driver modelling in a commuter scenario.
Transportation Research Part C: Emerging Technolo-
gies, 10(5-6):373–398.
Salvucci, D. D. (2006). Modeling Driver Behavior in a Cog-
nitive Architecture. Human Factors and Ergonomics
Society, 48(2):362–380.
Schakel, W. J., Knoop, V. L., and van Arem, B. (2012).
Integrated Lane Change Model with Relaxation and
Synchronization. Transportation Research Record,
2316:47–57.
Smith, L., Beckman, R., and Baggerly, K. (1995). TRAN-
SIMS: Transportation analysis and simulation system.
Technical report, Los Alamos National Lab (United
States).
ICAART2014-InternationalConferenceonAgentsandArtificialIntelligence
154