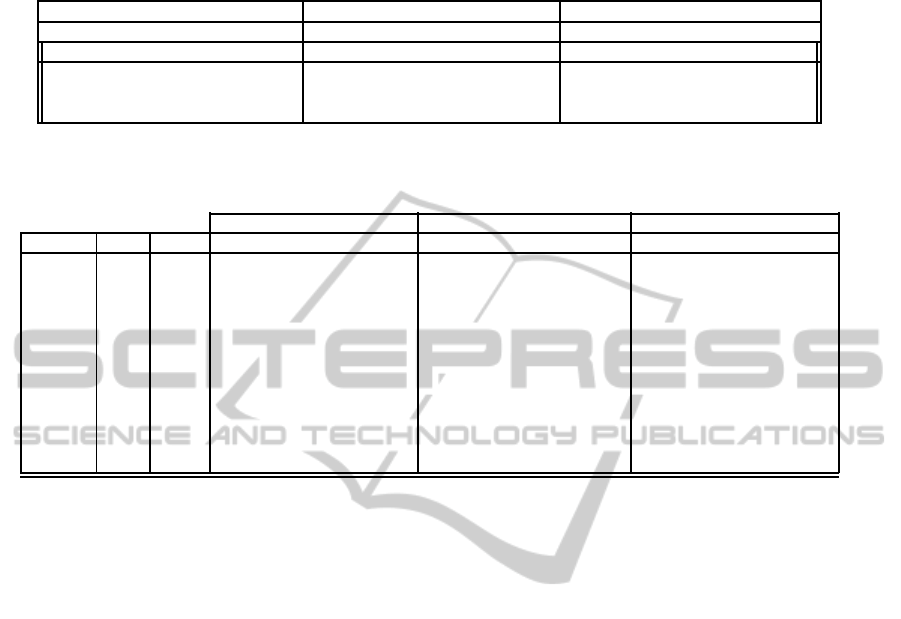
Table 2: Ocean image and its copy distorted by respectively Gaussian blurring (top), fastfading (middle) and white noise
(bottom). Mean and standard deviation (in the brackets) of the relative error (%) for the estimation of the true value of SSIM
and SNR using just 100 blocks with size equal to: 8× 8, 16× 16 and 32× 32.
Block size = 8 /No. Blocks = 100 Block size = 16 /No. Blocks = 100 Block size = 32 /No. Blocks = 100
Total blocks: 6144 Total blocks: 1536 Total blocks: 384
SSIM error SNR error SSIM error SNR error SSIM error SNR error
1.84% (1.41%) 2.46%(2.14%) 1.25%(1.01%) 2.43%(1.63)% 1.40%(1.20%) 2.2% (1.72%)
1.33%(1.42%) 2.75%(2.00%) 1.39%(0.80%) 2.50%(1.90%) 0.79%(0.55%) 2.25%(1.71%)
1.14%(0.87%) 1.31%(1.03%) 1.09%(0.20%) 1.07%(0.70%) 0.71%(0.54%) 0.81%(0.57%)
Table 3: Images in Fig. 3 with different kinds of distortions. Mean value and standard deviation (in brackets) of the relative
error (%) as in eq. (3) for the estimation of the true value of SSIM and SNR considering just 50, 100 and 200 non overlapping
blocks with size equal to 16× 16.
Blk size:16 / Sel. Blks:50 Blk size:16 / Sel. Blks:100 Blk size:16 / Sel. Blks:200
Image Dist Blks SSIM error SNR error SSIM error SNR error SSIM error SNR error
Ocean Gb 1536 2.53% (2.49) 4.15% (2.70) 1.25% (1.01) 2.43% (1.63) 1.16%(1.03) 1.82% (1.18)
Stream Gb 1536 3.50%(3.00) 2.65%(2.25) 3.45%(3.01) 2.29% (1.80) 2.43%(2.01) 1.66%(1.02)
Lighth. Gb 1350 2.45%(2.20) 3.72%(2.01) 2.30%(1.80) 2.39%(2.22) 1.56%(1.21) 1.75%(1.15)
Sail4 Gb 1536 2.13%(2.00) 3.53%(2.99) 1.47%(0.80) 3.26%(2.22) 1.02%(0.45) 1.47%(0.89)
Ocean Ff 1536 1.53% (1.20) 3.18%(2.05) 1.39%(0.80) 2.50%(1.90) 0.83%(0.52) 1.86%(1.01)
House Ff 1536 1.08%(0.99) 2.68%(1.90) 0.75%(0.57) 1.73%(1.60) 0.55%(0.21) 1.36%(0.94)
Stream Jp 1536 2.93%(2.90) 2.78%(2.09) 1.95%(1.50) 1.84%(1.30) 1.56%(0.98) 1.11%(0.80)
Flower Jp 1280 0.58%(0.50) 4.01%(3.03) 0.30%(0.15) 2.62%(2.15) 0.23%(0.09) 1.89%(1.01)
Ocean Wn 1536 1.19%(0.70) 1.29%(0.90) 1.09%(0.80) 1.07%(0.70) 0.61%(0.30) 0.72%(0.31)
House Wn 1536 5.46%(2.09) 2.04%(1.77) 4.40%(2.87%) 1.93%(1.35) 2.72%(2.51) 0.93%(0.80)
Flower Wn 1280 2.82%(2.77) 1.45% (1.35) 1.86%(1.52%) 0.90%(0.50) 1.09%(1.08) 0.56% (0.53)
REFERENCES
Benabdelkader, S. and Boulemden, M. (2005). Recursive
algorithm based on fuzzy 2-partition entropy for 2-
level image thresholding. In Pattern Recognition, 38.
Elsevier.
Bruni, V., Rossi, E., and Vitulano, D. (2013a). Jensen-
shannon divergence for visual quality assessment. In
Signal Image and Video Prcessing 7(3). Springer.
Bruni, V., Vitulano, D., and Ramponi, G. (2011). Image
quality assessment through a subset of the image data.
In Proc. of ISPA 2011. IEEE.
Bruni, V., Vitulano, D., and Wang, Z. (2013b). Special issue
on human vision and information theory. In Signal
Image and Video Prcessing 7(3). Springer.
Cover, T. M. and Thomas, J. A. (1991). Elements of Infor-
mation Theory. John Wiley & sons.
Frazor, R. and Geisler, W. (2006). Local luminance and
contrast in natural in natural images, 46. In Vision
Research.
Gonzalez, R. C. and Woods, R. E. (2002). Digital Image
Processing. Prentice Hall, 2nd edition.
Grunwald, P. D. (2004). A tutorial introduction to the min-
imum description length principle. In Advances in
Minimum Description Length: Theory and Applica-
tions. Myung Grunwald, Pitt,.
Monte, V., Frazor, R., Bonin, V., Geisler, W., and Corandin,
M. (2005). Independence of luminance and contrast
in natural scenes and in the early visual system 8(12).
In Nature Neuroscience.
Rivera, M., Ocegueda, O., and Marroquin, J. L. (2007).
Entropy-controlled quadratic markov measure field
models for efficient image segmentation. In IEEE
Trans. on Image Proc. 16(12).
Sheikh, H. R. and Bovik, A. C. (2006). Image information
and visual quality. In IEEE Trans. on Image Proc.,
15(2).
Sheikh, H. R., Bovik, A. C., and Veciana, G. D. (2005). An
information fidelity criterion for image quality assess-
ment using natural scene statistics. In IEEE Trans. on
Image Proc., 14(12).
Sheikh, H. R., Wang, Z., Cormack, L., and
Bovik, A. C. Live image quality assess-
ment database release 2. [Online]. Avail-
able:http://live.ece.utexas.edu/research/quality.
Wang, W., Wang, Y., Huang, Q., and Gao, W. (2010). Mea-
suring visual saliency by site entropy rate. In Proc. of
CVPR10. IEEE.
Wang, Z., Bovik, A. C., Sheikh, H. R., and Simoncelli, E. P.
(2004a). Image quality assessment: From error visi-
bility to structural similarity. In IEEE Trans. on Image
Proc., 13.
Wang, Z. and E.P.Simoncelli (2005). Reduced-reference
image quality assessment using a wavelet-domain nat-
ural image statistic model. In Proc. of Human Vision
and Electronic Imaging X, vol. 5666. SPIE.
Wang, Z. and Li, Q. (2011). Information content weight-
ing for perceptual image quality assessment. In IEEE
Trans. on Image Proc. 20(5).
Wang, Z., Lu, L., and Bovik, A. (2004b). Video quality as-
AFastComputationMethodforIQAMetricsBasedontheirTypicalSet
205