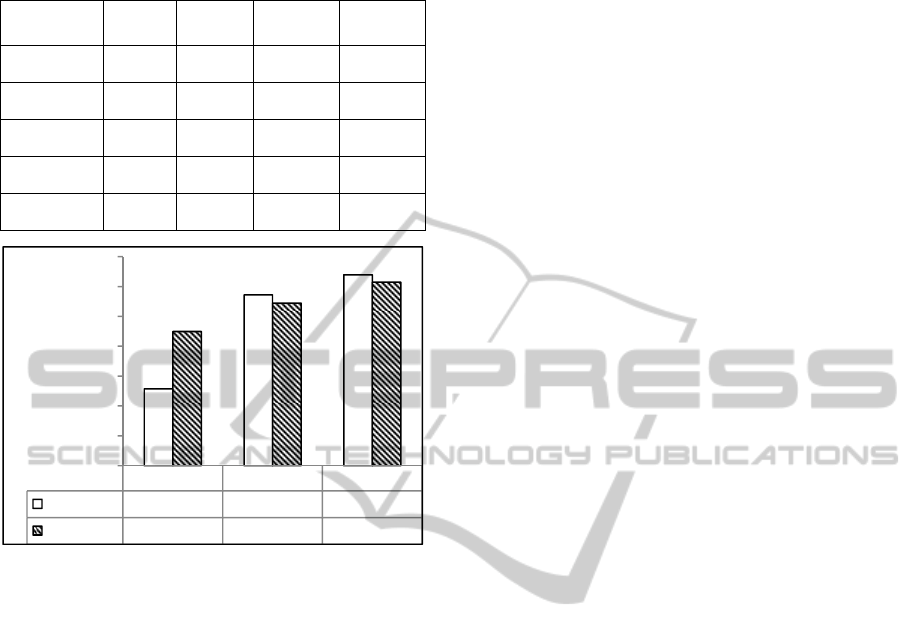
Table 4: Results for a 7-input ANFIS model (using PSNR,
UQI, MSSIM, WSNR, VIF, NQM, and IFC).
Distortion
s
MAE RMSE
Blur 0.9937 0.9902 2.2626 3.2177
JPEG 2000 0.9944 0.9902 2.4395 3.3292
JPEG 0.9814 0.9758 5.7428 7.1629
APGN 0.7035 0.7338 96.1683 99.8975
AWGN 0.9548 0.9590 16.8990 22.7204
Figure 6: Comparing the average correlation results for
JPEG/JPEG 2000 distortion for three methods: ANFIS
with 7 inputs (proposed), neural network (NN)
(Bouzerdoum et al., 2004) and MSSIM (Wang et al.,
2004).
6 CONCLUSIONS
In this paper, we explored the application of an
adaptive neuro-fuzzy inference system (ANFIS) for
full-reference assessing the quality of images with
references. The experimental results showed that
ANFIS network can be trained using image quality
assessment metrics to predict the differential mean
opinion score (DMOS) with high correlation
coefficients and low errors. The ANFIS results
compare favourably with two other methods in the
literature. As a future work, the proposed method
can be evaluated using k-fold cross validation and
other databases. More quality assessment metrics
can be considered and in this case the selection of
the most relevant features for building the predictive
models will be of interest.
ACKNOWLEDGEMENTS
The authors would like to acknowledge the support
provided by the Deanship of Scientific Research at
King Fahd University of Petroleum & Minerals
(KFUPM). The authors would also like to thank the
anonymous reviewers for providing helpful and
constructive comments that helped enhancing the
paper presentation and content.
REFERENCES
Balamurugan, P., Rajesh, R., 2007. Greenery image and
non-greenery image classification using adaptive
neuro-fuzzy inference system. In International
Conference on Computational Intelligence and
Multimedia Applications.
Bouzerdoum, A., Havstad, A., Beghdadi. A., 2004. Image
quality assessment using a neural network approach.
In Fourth IEEE International Symposium on Signal
Processing and Information Technology.
Chetouani, A., Beghdadi, A., Deriche, M., 2010. Image
distortion analysis and classification scheme using a
neural approach. In 2nd European Workshop on
Visual Information Processing (EUVIP).
Damera-Venkata, N., Kite, T., Geisler, W., Evans, B.,
Bovik, A., 2000. Image quality assessment based on a
degradation model. IEEE Transactions on Image
Processing, 9(4): 636-650.
De, I., Sil, J., 2009. No-reference quality prediction of
distorted/decompressed images using ANFIS. In
International Conference on Computer Technology
and Development.
He, L., Gao, F., Hou W., Hao, L., 2013. Objective image
quality assessment: a survey. International Journal of
Computer Mathematics.
Kaya, S., Milanova, M., Talburt, J., Tsou, B., Altynova,
M. 2011. Subjective image quality prediction based
on neural network. In Proceedings of the 16th
International Conference on Information Quality.
Kudelka Jr., M., 2012. Image quality assessment. WDS'12
Proceedings of Contributed Papers, Part I, 94–99.
Kung, C.-H., Yang, W.-S., Huang, C.-Y., Kung, C.-M.,
2010. Investigation of the image quality assessment
using neural networks and structure similarity. In
Proceedings of the 3rd International Symposium
Computer Science and Computational Technology.
Jang, J. S. R., 1993. ANFIS: Adaptive-network-based
fuzzy inference system. IEEE Transactions on
Systems, Man, Cybernetics, 23(5/6):665–685.
Khuntia, S. R., Panda, S. 2011. ANFIS approach for SSSC
controller design for the improvement of transient
stability performance. Mathematical and Computer
Modelling, 57(1–2): 289–300.
Larson, E. C., Chandler, D. M. 2010. Most apparent
distortion: full-reference image quality assessment and
MSSIM NN ANFIS
Pearson
0,9114 0,9744 0,9879
Spearman
0,9499 0,969 0,983
0,86
0,88
0,9
0,92
0,94
0,96
0,98
1
ICAART2014-InternationalConferenceonAgentsandArtificialIntelligence
176