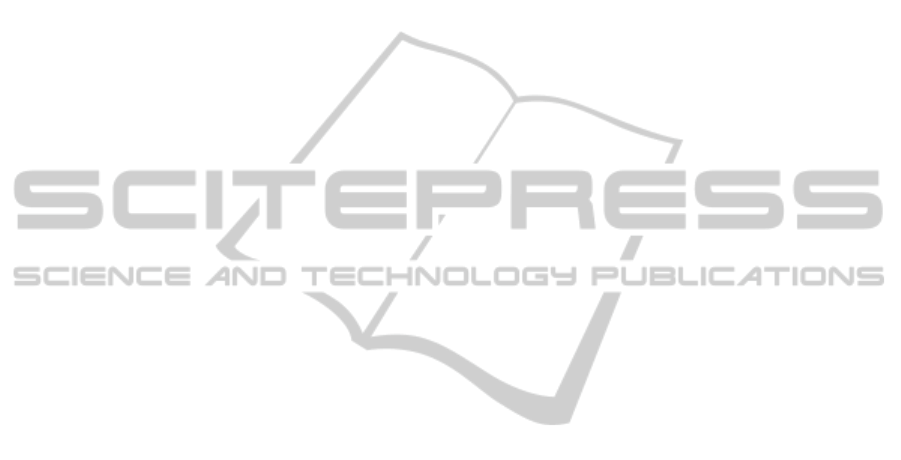
However, this approach does require the videos to
be already well segmented and aligned, which means
that in each video, there should be only one full per-
formance of an action, i.e. actions of the same type
start from the same pose and end at the same pose. In
future work, we shall explore automatic subsequence
segmentation methods, in order to obtain meaningful
subsequences corresponding to action atoms. There-
fore, the performance could be improved further on
different kinds of datasets. Also, in this work, the
weight of each histogram is set by experience, which
can be learned automatically in future.
ACKNOWLEDGEMENTS
We would like to thank Dr. Piotr Doll
´
ar for gener-
ously sharing the feature extraction source code and
toolbox.
REFERENCES
Blank, M., Gorelick, L., Shechtman, E., Irani, M., and
Basri, R. (2005). Actions as space-time shapes. In
ICCV 2005, volume 2, pages 1395–1402 Vol. 2.
Bobick, A. and Davis, J. (2001). The recognition of human
movement using temporal templates. Pattern Analy-
sis and Machine Intelligence, IEEE Transactions on,
23(3):257–267.
Bosch, A., Zisserman, A., and Munoz, X. (2007). Repre-
senting shape with a spatial pyramid kernel. In Pro-
ceedings of the 6th ACM international conference on
Image and video retrieval, pages 401–408. ACM.
Choi, J., Jeon, W. J., and Lee, S.-C. (2008). Spatio-temporal
pyramid matching for sports videos. In Proceedings
of the 1st ACM International Conference on Multime-
dia Information Retrieval, MIR ’08, pages 291–297.
ACM.
Doll
´
ar, P., Rabaud, V., Cottrell, G., and Belongie, S. (2005).
Behavior recognition via sparse spatio-temporal fea-
tures. In Visual Surveillance and Performance Eval-
uation of Tracking and Surveillance, 2005. 2nd Joint
IEEE International Workshop on, pages 65–72.
Efros, A., Berg, A., Mori, G., and Malik, J. (2003). Rec-
ognizing action at a distance. In Computer Vision,
2003. Proceedings. Ninth IEEE International Confer-
ence on, pages 726–733 vol.2.
Gilbert, A., Illingworth, J., and Bowden, R. (2009). Fast
realistic multi-action recognition using mined dense
spatio-temporal features. In Computer Vision, 2009
IEEE 12th International Conference on, pages 925–
931.
Laptev, I. and Lindeberg, T. (2003). Space-time inter-
est points. In Computer Vision, 2003. Proceedings.
Ninth IEEE International Conference on, pages 432–
439 vol.1.
Lazebnik, S., Schmid, C., and Ponce, J. (2006). Beyond
bags of features: Spatial pyramid matching for recog-
nizing natural scene categories. In CVPR2006, vol-
ume 2, pages 2169–2178.
Marszalek, M., Laptev, I., and Schmid, C. (2009). Actions
in context. In CVPR 2009, pages 2929–2936.
Niebles, J. C., Wang, H., and Fei-fei, L. (2006). Unsu-
pervised learning of human action categories using
spatial-temporal words. In In Proc. BMVC.
Oikonomopoulos, A., Patras, I., and Pantic, M. (2005). Spa-
tiotemporal salient points for visual recognition of hu-
man actions. Systems, Man, and Cybernetics, Part B:
Cybernetics, IEEE Transactions on, 36(3):710–719.
Poppe, R. (2010). A survey on vision-based human action
recognition. Image Vision Comput., 28(6):976–990.
Schuldt, C., Laptev, I., and Caputo, B. (2004). Recognizing
human actions: a local svm approach. In ICPR 2004,
volume 3, pages 32–36 Vol.3.
Scovanner, P., Ali, S., and Shah, M. (2007). A 3-
dimensional sift descriptor and its application to ac-
tion recognition. In Proceedings of the 15th Inter-
national Conference on Multimedia, pages 357–360.
ACM.
Shen, Y. and Foroosh, H. (2008). View-invariant recogni-
tion of body pose from space-time templates. In CVPR
2008, pages 1–6.
Sun, J., Wu, X., Yan, S., Cheong, L.-F., Chua, T.-S., and Li,
J. (2009). Hierarchical spatio-temporal context mod-
eling for action recognition. In CVPR 2009, pages
2004–2011.
Wang, H., Ullah, M. M., Klser, A., Laptev, I., and Schmid,
C. (2009). Evaluation of local spatio-temporal fea-
tures for action recognition. In University of Central
Florida, U.S.A.
Wang, Y., Huang, K., and Tan, T. (2007). Human activ-
ity recognition based on r transform. In CVPR2007,
pages 1–8.
Willems, G., Tuytelaars, T., and Gool, L. (2008). An effi-
cient dense and scale-invariant spatio-temporal inter-
est point detector. In Proceedings of the 10th Euro-
pean Conference on Computer Vision: Part II, pages
650–663. Springer-Verlag.
ICPRAM2014-InternationalConferenceonPatternRecognitionApplicationsandMethods
636