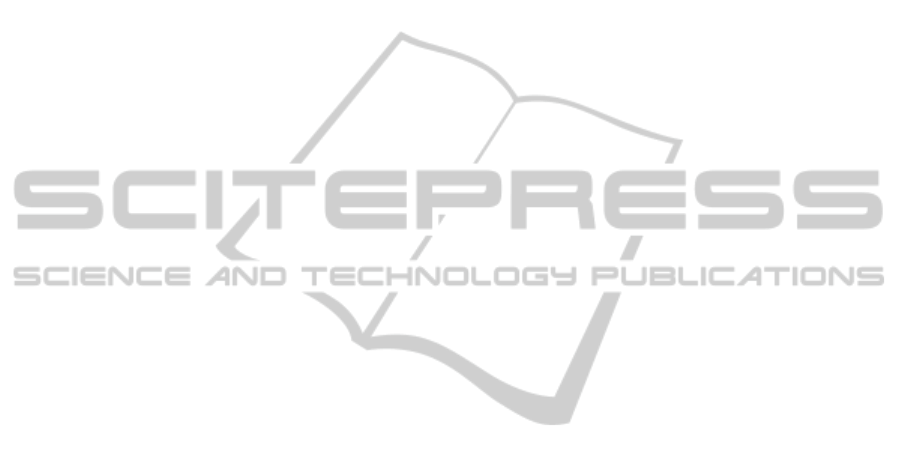
pendent and study of finding such classifiers on larger
datasets is left to future studies. Meanwhile, due to
the arguments in §3, a similar behaviour is expected
on any dataset if the replacement classifier is correctly
trained.
ACKNOWLEDGEMENTS
This work was supported by The Swedish Foundation
for Strategic Research in the project Wearable Visual
Information Systems”.
REFERENCES
Afkham, H. M., Carlsson, S., and Sullivan, J. (2012). Im-
proving Feature Level Likelihoods using Cloud Fea-
tures. In ICPRAM (2), pages 431–437.
Afkham, H. M., Ek, C. H., and Carlsson, S. (2013). Qual-
itative Vocabulary Based Descriptor. In International
Conference on Pattern Recognition Applications and
Methods, pages 1–6.
Csurka, G. and Perronnin, F. (2011). Fisher Vectors: Be-
yond Bag-of-Visual-Words Image Representations. In
Richard, P. and Braz, J., editors, Computer Vision,
Imaging and Computer Graphics. Theory and Appli-
cations, pages 28–42. Springer Berlin Heidelberg.
Dalal, N. and Triggs, B. (2005). Histograms of Oriented
Gradients for Human Detection. In CVPR (1), pages
886–893.
Fan, R.-E., Chang, K.-W., Hsieh, C.-J., Wang, X.-R., and
Lin, C.-J. (2008). LIBLINEAR: A Library for Large
Linear Classification. Journal of Machine Learning
Research, 9:1871–1874.
Felzenszwalb, P. F., Girshick, R. B., McAllester, D., and
Ramanan, D. (2010). Object Detection with Dis-
criminatively Trained Part-Based Models. PAMI,
32(9):1627–1645.
Kumar, M. P., Packer, B., and Koller, D. (2010). Self-Paced
Learning for Latent Variable Models. In Lafferty, J.,
Williams, C. K. I., Shawe-Taylor, J., Zemel, R. S., and
Culotta, A., editors, Advances in Neural Information
Processing Systems 23, pages 1189–1197.
Lazebnik, S., Schmid, C., and Ponce, J. (2006). Beyond
Bags of Features: Spatial Pyramid Matching for Rec-
ognizing Natural Scene Categories. In CVPR, pages
2169–2178.
Lowe, D. G. (2004). Distinctive Image Features from Scale-
Invariant Keypoints. International Journal of Com-
puter Vision, 60(2):91–110.
Madry, M., Afkham, H. M., Ek, C. H., Carlsson, S., and
Kragic, D. (2013). Extracting Essential Local Ob-
ject Characteristics for 3D Object Categorization. In
IEEE International Conference on Intelligent Robots
and Systems (IROS).
Morioka, N. and Satoh, S. (2010). Building Compact Local
Pairwise Codebook with Joint Feature Space Cluster-
ing. In ECCV (1), pages 692–705.
Savarese, S., Winn, J., and Criminisi, A. (2006). Discrimi-
native Object Class Models of Appearance and Shape
by Correlatons. In CVPR.
Schroff, F., Criminisi, A., and Zisserman, A. (2008). Object
Class Segmentation using Random Forests. In Pro-
ceedings of the British Machine Vision Conference,
pages 54.1–54.10. BMVA Press.
Vedaldi, A. and Fulkerson, B. (2008). VLFeat: An Open
and Portable Library of Computer Vision Algorithms.
Technical report.
Viola, P. and Jones, M. (2001). Robust real-time face detec-
tion. In Computer Vision, 2001. ICCV 2001. Proceed-
ings. Eighth IEEE International Conference on, page
747.
Winn, J., Criminisi, A., and Minka, T. (2005). Object Cate-
gorization by Learned Universal Visual Dictionary. In
ICCV.
Yang, W., Wang, Y., Vahdat, A., and Mori, G. (2012). Ker-
nel Latent SVM for Visual Recognition. In Bartlett,
P., Pereira, F. C. N., Burges, C. J. C., Bottou, L., and
Weinberger, K. Q., editors, Advances in Neural Infor-
mation Processing Systems 25, pages 818–826.
Zhang, Y. and Chen, T. (2009). Efficient Kernels for identi-
fying unbounded-order spatial features. In CVPR.
ICPRAM2014-InternationalConferenceonPatternRecognitionApplicationsandMethods
238