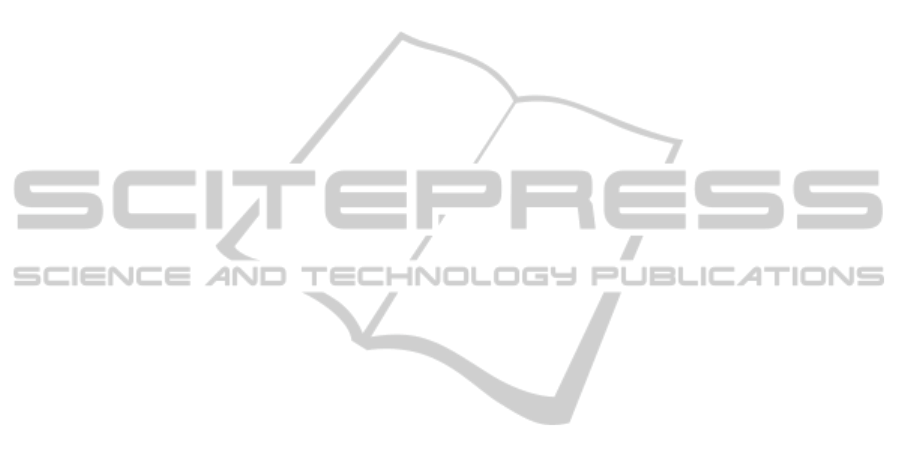
method in pattern recognition learning. Automation
and remote control, 25:821–837.
Barbar´a, D. and Jajodia, S. (2002). Applications of data
mining in computer security, volume 6. Springer.
Bezdek, J. C., Ehrlich, R., and Full, W. (1984). Fcm: The
fuzzy c-means clustering algorithm. Computers &
Geosciences, 10(2):191–203.
Cali´nski, T. and Harabasz, J. (1974). A dendrite method for
cluster analysis. Communications in Statistics-theory
and Methods, 3(1):1–27.
Cortes, C. and Vapnik, V. (1995). Support-vector networks.
Machine learning, 20(3):273–297.
Driver, H. E. and Kroeber, A. L. (1932). Quantitative ex-
pression of cultural relationships. University of Cali-
fornia Press.
Filippone, M., Camastra, F., Masulli, F., and Rovetta, S.
(2008). A survey of kernel and spectral methods for
clustering. Pattern recognition, 41(1):176–190.
Fraley, C. and Raftery, A. E. (1998). How many clusters?
which clustering method? answers via model-based
cluster analysis. The computer journal, 41(8):578–
588.
Girolami, M. (2002). Mercer kernel-based clustering in fea-
ture space. Neural Networks, IEEE Transactions on,
13(3):780–784.
Gordon, A. D. (1996). Null models in cluster validation. In
From data to knowledge, pages 32–44. Springer.
Harman, H. H. (1960). Modern factor analysis.
Hartigan, J. A. (1975). Clustering Algorithms. John Wiley
& Sons, Inc., New York, NY, USA, 99th edition.
Kaufman, L. and Rousseeuw, P. J. (2009). Finding groups
in data: an introduction to cluster analysis, volume
344. Wiley-Interscience.
Kim, D.-W., Lee, K. Y., Lee, D., and Lee, K. H. (2005).
Evaluation of the performance of clustering algo-
rithms in kernel-induced feature space. Pattern
Recognition, 38(4):607–611.
Krzanowski, W. J. and Lai, Y. (1988). A criterion for deter-
mining the number of groups in a data set using sum-
of-squares clustering. Biometrics, pages 23–34.
Mac Queen, J. et al. (1967). Some methods for classifi-
cation and analysis of multivariate observations. In
Proceedings of the fifth Berkeley symposium on math-
ematical statistics and probability, volume 1, page 14.
California, USA.
Mercer, J. (1909). Functions of positive and negative type,
and their connection with the theory of integral equa-
tions. Philosophical transactions of the royal society
of London. Series A, containing papers of a mathe-
matical or physical character, 209:415–446.
Milligan, G. W. and Cooper, M. C. (1985). An examination
of procedures for determining the number of clusters
in a data set. Psychometrika, 50(2):159–179.
Muller, K.-R., Mika, S., Ratsch, G., Tsuda, K., and
Scholkopf, B. (2001). An introduction to kernel-based
learning algorithms. Neural Networks, IEEE Transac-
tions on, 12(2):181–201.
Murtagh, F. (1983). A survey of recent advances in hierar-
chical clustering algorithms. The Computer Journal,
26(4):354–359.
Olson, C. F. (1995). Parallel algorithms for hierarchical
clustering. Parallel computing, 21(8):1313–1325.
Pr´ıncipe, J. C., Liu, W., and Haykin, S. (2011). Kernel
Adaptive Filtering: A Comprehensive Introduction,
volume 57. John Wiley & Sons.
Qin, J., Lewis, D. P., and Noble, W. S. (2003). Kernel hi-
erarchical gene clustering from microarray expression
data. Bioinformatics, 19(16):2097–2104.
Sch¨olkopf, B., Smola, A., and M¨uller, K.-R. (1998). Non-
linear component analysis as a kernel eigenvalue prob-
lem. Neural computation, 10(5):1299–1319.
Shawe-Taylor, N. and Kandola, A. (2002). On kernel target
alignment. Advances in neural information processing
systems, 14:367.
Sneath, P. H., Sokal, R. R., et al. (1973). Numerical taxon-
omy. The principles and practice of numerical classi-
fication.
Sugar, C. A. and James, G. M. (2003). Finding the num-
ber of clusters in a dataset. Journal of the American
Statistical Association, 98(463).
Tibshirani, R., Walther, G., and Hastie, T. (2001). Estimat-
ing the number of clusters in a data set via the gap
statistic. Journal of the Royal Statistical Society: Se-
ries B (Statistical Methodology), 63(2):411–423.
Vapnik, V. (2000). The nature of statistical learning theory.
springer.
ICPRAM2014-InternationalConferenceonPatternRecognitionApplicationsandMethods
262