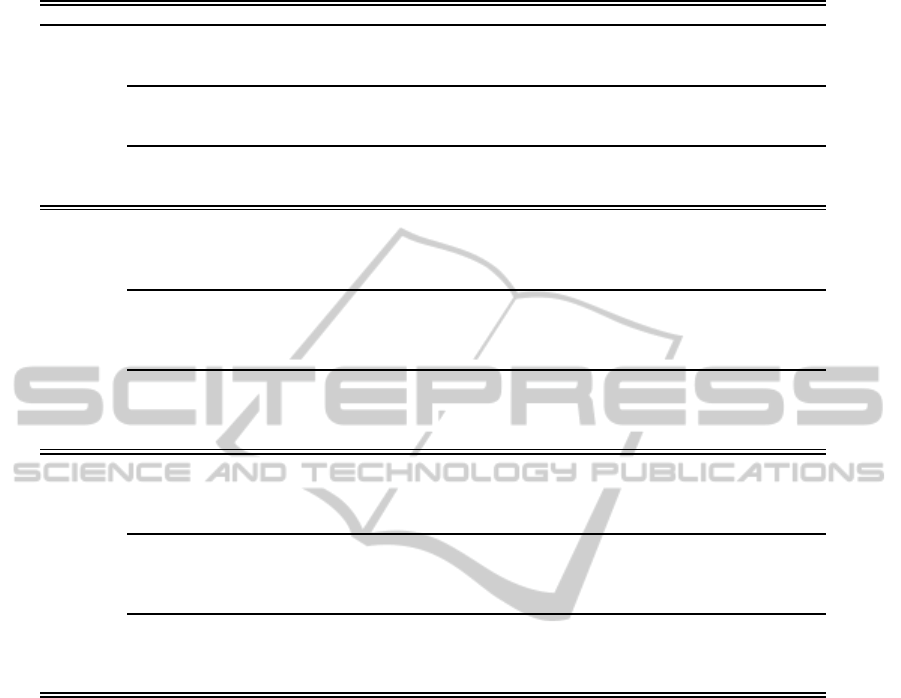
Table 3: Sensitivity of proposed methods to the parameter γ on Benchmark data sets.
Data sets Methods Precision Recall F-measure Rand Index
EachMovie Robust Parametrized-OKM(α = 0,γ = 1) 0.632± 0.02 0.886± 0.05 0.737±0.03 0.635± 0.04
Robust Parametrized-OKM(α = 0,γ = 0.8) 0.627± 0.01 0.857± 0.02 0.724± 0.02 0.621± 0.03
Robust Parametrized-OKM(α = 0,γ = 0.7) 0.623± 0.01 0.868± 0.04 0.725± 0.01 0.619± 0.02
Robust Parametrized R-OKM(α = 1,γ = 1) 0.691± 0.05 0.635± 0.03 0.659±0.03 0.619± 0.05
Robust Parametrized R-OKM(α = 1,γ = 0.7) 0.691±0.06 0.621± 0.06 0.652± 0.04 0.611± 0.06
Robust Parametrized R-OKM(α = 1,γ = 0.5) 0.661± 0.03 0.605± 0.07 0.631± 0.05 0.583± 0.04
Robust Parametrized R-OKM(α = 1.5,γ = 1) 0.719±0.10 0.632± 0.05 0.668±0.05 0.631± 0.06
Robust Parametrized R-OKM(α = 1.5,γ = 0.7) 0.711± 0.09 0.611± 0.05 0.653± 0.01 0.617± 0.18
Robust Parametrized R-OKM(α = 1.5,γ = 0.5) 0.663± 0.03 0.603± 0.07 0.630± 0.05 0.583± 0.04
Emotion Robust Parametrized-OKM(α = 0,γ = 0.3) 0.659± 0.005 0.519± 0.038 0.580± 0.022 0.521± 0.007
Robust Parametrized-OKM(α = 0,γ = 0.4) 0.657± 0.005 0.491± 0.013 0.562± 0.006 0.510± 0.000
Robust Parametrized-OKM(α = 0,γ = 0.8) 0.654± 0.003 0.492± 0.039 0.561± 0.024 0.507± 0.009
Robust Parametrized-OKM(α = 0,γ = 5.0) 0.661± 0.006 0.487± 0.02 0.560± 0.011 0.510± 0.002
Robust Parametrized R-OKM(α = 5,γ = 0,1) 0.698± 0.000 0.222± 0.021 0.337± 0.024 0.440± 0.004
Robust Parametrized R-OKM(α = 5,γ = 0,5) 0.677± 0.00 0.203± 0.00 0.313± 0.00 0.428± 0.000
Robust Parametrized R-OKM(α = 5,γ = 0.7) 0.679± 0.002 0.207± 0.00 0.318± 0.00 0.429± 0.00
Robust Parametrized R-OKM(α = 5,γ = 1.0) 0.672± 0.000 0.200± 0.004 0.308± 0.004 0.424± 0.001
Robust Parametrized R-OKM(α = 0.1,γ = 0,1) 0.700± 0.002 0.285± 0.006 0.405± 0.006 0.462± 0.003
Robust Parametrized R-OKM(α = 0.1,γ = 0.3) 0.681± 0.001 0.244± 0.015 0.388± 0.011 0.454± 0.004
Robust Parametrized R-OKM(α = 0.1,γ = 0.5) 0.676± 0.002 0.262± 0.034 0.377± 0.036 0.447± 0.011
Robust Parametrized R-OKM(α = 0.1,γ = 1.0) 0.676± 0.001 0.256± 0.037 0.370± 0.039 0.445± 0.013
Scene Robust Parametrized-OKM(α = 2.0,γ = 0.2) 0.514± 0.055 0.960± 0.000 0.672± 0.040 0.509± 0.051
Robust Parametrized-OKM(α = 2.0,γ = 0.5) 0.480± 0.050 0.557± 0.048 0.511± 0.009 0.578± 0.025
Robust Parametrized-OKM(α = 2.0,γ = 0.8) 0.488± 0.030 0.652± 0.119 0.548± 0.023 0.632± 0.019
Robust Parametrized-OKM(α = 2.0,γ = 5.0) 0.514± 0.000 0.682± 0.005 0.586± 0.001 0.688± 0.000
Robust Parametrized R-OKM(α = 0.8,γ = 0.2) 0.514± 0.053 0.960± 0.000 0.668± 0.045 0.509± 0.051
Robust Parametrized R-OKM(α = 0.8,γ = 0.5) 0.492± 0.041 0.585± 0.064 0.529± 0.002 0.593± 0.013
Robust Parametrized R-OKM(α = 0.8,γ = 1.0) 0.471± 0.020 0.672± 0.110 0.548± 0.023 0.631± 0.018
Robust Parametrized R-OKM(α = 0.8,γ = 5.0) 0.514± 0.000 0.726± 0.039 0.586± 0.002 0.688± 0.000
Robust Parametrized R-OKM(α = 0.4,γ = 0.2) 0.514± 0.053 0.960± 0.000 0.668± 0.045 0.509± 0.051
Robust Parametrized R-OKM(α = 0.4,γ = 0.8) 0.473± 0.018 0.639± 0.135 0.536± 0.038 0.623± 0.009
Robust Parametrized R-OKM(α = 0.4,γ = 1.0) 0.525± 0.002 0.672± 0.003 0.590± 0.003 0.686± 0.000
Robust Parametrized R-OKM(α = 0.4,γ = 5.0) 0.516± 0.002 0.684± 0.008 0.588± 0.004 0.689± 0.001
Table 3 evaluates the sensitivity of proposed
method to the parameter γ respectively on Emotion,
EachMovie and Scene data sets. Using EachMovie
and Scene data sets, F-measure and Rand Index de-
crease when γ decrease. However F-measure and
Rand Index decrease when γ increase using Emotion
data set.
6 CONCLUSIONS
Overlapping clustering is a necessary requirement for
many applications of clustering where data need to
be assigned to more than one cluster. Existing over-
lapping clustering methods can produce non disjoint
clusters, but its is not well adapted for clustering noisy
data. The performance of these methods are reduced
when data contain noisy observations. The proposed
method, Robust Parametrized R-OKM solves this is-
sue and identifies more relevant clusters which fit the
true structures in data. Experiments performed in arti-
ficial and real data sets showed the robustness of pro-
posed method when data contain noise.
As future work, we plan to confirm preliminary
obtained results on other real overlapping data sets.
Instead, one could add an auto adjusted value of γ to
automatically control the outliers boundaries in real
life applications of overlapping clustering.
REFERENCES
Battle, A., Segal, E., and Koller, D. (2005). Probabilis-
tic discovery of overlapping cellular processes and
their regulation. Journal of computational biology
: a journal of computational molecular cell biology,
12(7):909–927.
Ben N’Cir, C., Cleuziou, G., and Essoussi, N. (2013). Iden-
tification of non-disjoint clusters with small and pa-
rameterizable overlaps. In Computer Applications
Technology (ICCAT), 2013 International Conference
on, pages 1–6.
Bezdek, J. C. (1981). Pattern recognition with fuzzy objec-
tive function algoritms. Plenum Press, 4(2):67–76.
OverlappingClusteringwithOutliersDetection
285