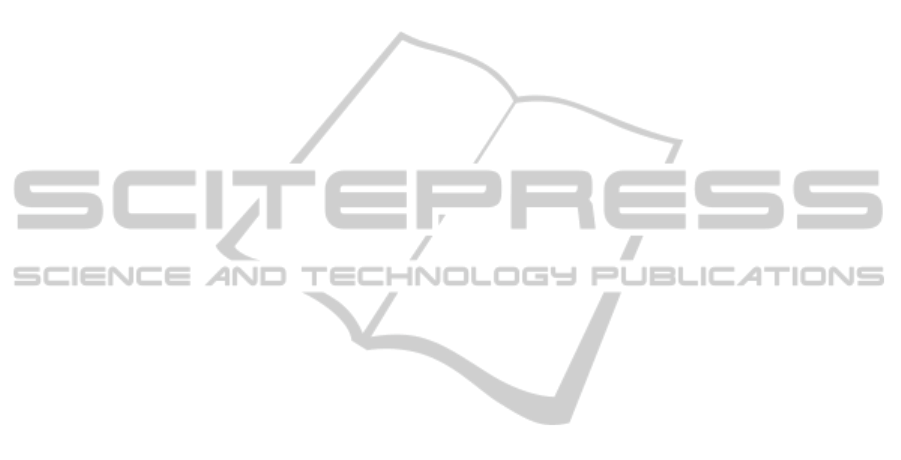
Gentle, J. (2007). Matrix algebra: theory, computations,
and applications in statistics. Springer texts in statis-
tics. Springer, New York, NY.
Gupta, A., Joshi, N., Zitnick, L., Cohen, M., and Curless, B.
(2010). Single image deblurring using motion density
functions. In ECCV ’10: Proc. of the 10th Europ.
Conf. on Comp. Vision.
Huang, J. (2000). Statistics of natural images and models.
PhD thesis, Brown Univ., Providence.
Ito, A., Sankaranarayanan, A. C., Veeraraghavan, A., and
Baraniuk, R. G. (2013). Blurburst: Removing blur due
to camera shake using multiple images. ACM Trans.
Graph. Submitted.
Ji, H. and Wang, K. (2012). A two-stage approach to blind
spatially-varying motion deblurring. In IEEE Conf.
on Comp. Vision and Pattern Recogn. (CVPR), pages
73–80. IEEE Comp. Soc.
Joshi, N., Zitnick, C., Szeliski, R., and Kriegman, D.
(2009). Image deblurring and denoising using color
priors. In IEEE Conf. on Comp. Vision and Pattern
Recognition (CVPR), pages 1550–1557. IEEE Comp.
Soc.
Krishnan, D. and Fergus, R. (2009). Fast image deconvo-
lution using hyper-laplacian priors. In Neural Inform.
Proc. Sys.
Levin, A., Fergus, R., Durand, F., and Freeman, W. T.
(2007a). Deconvolution using natural image priors.
Technical report, MIT.
Levin, A., Fergus, R., Durand, F., and Freeman, W. T.
(2007b). Image and depth from a conventional camera
with a coded aperture. ACM Trans. Graph., 26.
Levin, A. and Weiss, Y. (2007). User assisted separation
of reflections from a single image using a sparsity
prior. IEEE Trans. Pattern Anal. and Mach. Intell.,
29(9):1647–1654.
Li, X., Pan, J., Lin, Y., and Su, Z. (2013). Fast blind deblur-
ring via normalized sparsity prior. Journal of Inform.
& Comp. Sc., 10(16):5083–5091.
Lin, H. T., Tai, Y.-W., and Brown, M. S. (2011). Motion reg-
ularization for matting motion blurred objects. IEEE
Trans. Pattern Anal. and Mach. Intell., 33(11):2329–
2336.
Liu, R., Li, Z., and Jia, J. (2008). Image partial blur de-
tection and classification. In IEEE Conf. on Comp.
Vision and Pattern Recognition (CVPR), pages 1–8.
IEEE Comp. Soc.
Marichal, X., Ma, W.-Y., and Zhang, H.-J. (1999). Blur
determination in the compressed domain using DCT
information. In Intern. Conf. on Image Proc. (ICIP),
pages 386–390.
Meer, P. (2004). Robust techniques for computer vision.
In Medioni, G. and Kang, S. B., editors, Emerging
Topics in Computer Vision, chapter 4. Prentice Hall
PTR, Upper Saddle River, NJ.
Scales, J. A. and Gersztenkorn, A. (1988). Robust methods
in inverse theory. Inverse Problems, 4(4):1071.
Scales, J. A., Gersztenkorn, A., and Treitel, S. (1988). Fast
l
p
solution of large, sparse, linear systems: Applica-
tion to seismic travel time tomography. Journal of
Computational Physics, 75:314–333.
Schmidt, U., Rother, C., Nowozin, S., Jancsary, J., and
Roth, S. (2013). Discriminative non-blind deblurring.
In IEEE Conf. on Comp. Vision and Pattern Recogni-
tion (CVPR), pages 604–611. IEEE Comp. Soc.
Schmidt, U., Schelten, K., and Roth, S. (2011). Bayesian
deblurring with integrated noise estimation. In
IEEE Conf. on Comp. Vision and Pattern Recognition
(CVPR), pages 2625–2632. IEEE Comp. Soc.
Shi, M.-z., Xu, T.-f., Feng, L., Liang, J., and Zhang, K.
(2013). Single image deblurring using novel image
prior constraints. Optik - International Journal for
Light and Electron Optics, 124(20):4429–4434.
Simoncelli, E. (1997). Statistical models for images: Com-
pression, restoration and synthesis. In In 31st Asilo-
mar Conf on Signals, Systems and Computers, pages
673–678. IEEE Comp. Soc.
Sorel, M. and Sroubek, F. (2012). Restoration in the pres-
ence of unknown spatially varying blur. In Gunturk,
B. and Li, X., editors, Image Restoration: Fundamen-
tals and Advances, pages 63–88. CRC Press.
Srivastava, A., Lee, A. B., Simoncelli, E. P., and c. Zhu, S.
(2003). On advances in statistical modeling of natural
images. Journal of Math. Imaging and Vision, 18:17–
33.
Wang, S., Hou, T., Border, J., Qin, H., and Miller, R.
(2012). High-quality image deblurring with panchro-
matic pixels. ACM Trans. Graph., 31(5).
Wang, Z., Simoncelli, E. P., and Bovik, A. C. (2003). Multi-
scale structural similarity for image quality assess-
ment. In Proc. of the 37th IEEE Asilomar Conf. on
Signals, Systems and Computers.
Weiss, Y. and Freeman, W. (2007). What makes a good
model of natural images? In IEEE Conf. on Comp.
Vision and Pattern Recognition (CVPR), pages 1–8.
IEEE Comp. Soc.
Whyte, O., Sivic, J., Zisserman, A., and Ponce, J. (2012).
Non-uniform deblurring for shaken images. Intern.
Journal of Comp. Vision, 98(2):168–186.
Yuan, L., Sun, J., Quan, L., and Shum, H.-Y. (2007).
Blurred/non-blurred image alignment using sparse-
ness prior. In Proc. of the 11th Intern. Conf. on Comp.
Vision (ICCV), pages 1–8.
Yun-Fang, Z. (2010). Blur detection for surveillance video
based on heavy-tailed distribution. In Asia Pacific
Conf. on Postgrad. Research in Microelectronics and
Electronics, pages 101–105.
Zhong, L., Cho, S., Metaxas, D., Paris, S., and Wang, J.
(2013). Handling noise in single image deblurring us-
ing directional filters. In IEEE Conf. on Comp. Vision
and Pattern Recogn. (CVPR), pages 612–619. IEEE
Comp. Soc.
Zhou, X., Zhou, F., Bai, X., and Xue, B. (2014). A bound-
ary condition based deconvolution framework for im-
age deblurring. Journal of Computational and Applied
Mathematics, 261:14–19.
ICPRAM2014-InternationalConferenceonPatternRecognitionApplicationsandMethods
136