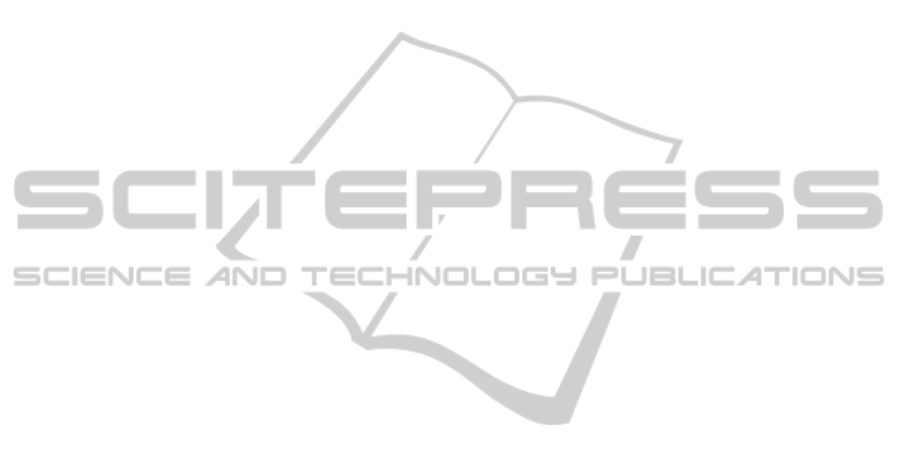
on time, it cannot be satisfied at a later time period,
and therefore we need to pay penalty for each unit.
Also, if the port is forced to hold some inventory, then
a holding cost is charged. In addition to all these cost
factors, we also need to consider the fact that each
vessel is available for a certain amount of time within
a period, and therefore even if a vessel has enough
capacity, it may not have enough time to visit all the
ports we desire.
Optimally solving distribution operations schedul-
ing problem is not an easy task. Previous work related
to industrial shipping varies a lot. Here, we focus
on the existing results that are closely related to our
work. A large summary of works related to various
types of vessel scheduling and routing problem can be
found in the literature survey by (Christiansen et al.,
2004). Two more recent surveys can be found more
specifically in the area of combined inventory man-
agement and routing ((Andersson et al., 2010)) and
on fleet composition and routing ((Hoff et al., 2010)).
The most recent literature survey is by (Christiansen
et al., 2012), which takes a look at the publications in
the last decade, and list possible research areas that
could be pursued in this area.
(Xinlian et al., 2000) presents an algorithm which
combines the linear programming technique with that
of dynamic programming to improve the solution to
linear model for fleet planning. Even though their ap-
proach is similar, the problem they are dealing with
requires demand satisfaction and initial fleet is al-
ready given, and the decision is to whether add new
vessels to the existing fleet or not.
(Cho and Perakis, 2001) presented a better formu-
lation to the original fleet deployment problem pro-
posed by (Ronen, 1986). In this formulation, just like
we do, there is a single loading port, finite number of
customer ports, and a finite planning horizon. How-
ever, they require the demand to be met, and the fleet
size is constant. The costs incurred are due to routes
chosen, shipping cargoes, and unloading time. They
show that this formulation is better for computational
efficiency.
(Cho and Perakis, 1996) present a study regarding
fleet size and design of optimal liner routes for a con-
tainer shipping company. The problem is solved by
generating a number of candidate routes for the dif-
ferent ships first, and then, the problem is formulated
and solved as a linear programming model, where the
columns represent the candidate routes. They extend
this model to a mixed integer programming model
that also considers investment alternatives to expand-
ing fleet capacity. (Bendall and Stent, 2001) also
present a model for determining the optimal number
of ships and fleet deployment plan.
On the other hand, (Nicholson and Pullen, 1971)
were the first ones to propose dynamic programming
application to ship fleet management. The problem
they dealt with was to determine the sequence in
which the currently owned ships should be sold and
the extent to which charter ships should be taken on.
They tackle the problem in two stages. The first stage
determines a good priority ordering for selling the
ships regardless of the rate at which charter ships are
taken on. The second stage uses dynamic program-
ming to determine an optimal level of chartering given
the priority replacement order. This first stage pri-
ority ordering essentially reduces the dynamic pro-
gramming calculation from a problem with as many
as states as number of ships in fleet to a 1 state vari-
able problem which is computationally manageable
by dynamic programming methods. Several authors
use benchmark instances to compare the results of
different strategies and heuristics. (Gheysens et al.,
1984) define 20 test instances with 12100 nodes for
the standard fleet size and mix vehicle routing prob-
lem. (Wu et al., 2005) deals with trucks that vary in
capacity and age are utilized over space and time to
meet customer demand. Operational decisions (in-
cluding demand allocation and empty truck reposi-
tioning) and tactical decisions (including asset pro-
curements and sales) are explicitly examined in a lin-
ear programming model to determine the optimal fleet
size and mix. The method uses a time-space network,
common to fleet-management problems, but also in-
cludes capital cost decisions, wherein assets of dif-
ferent ages carry different costs, as is common to re-
placement analysis problems. A two-phase solution
approach is developed to solve large-scale instances
of the problem. Phase I allocates customer demand
among assets through Benders decomposition with a
demand-shifting algorithm assuring feasibility in each
subproblem. Phase II uses the initial bounds and dual
variables from Phase I and further improves the so-
lution convergence through the use of Lagrangian re-
laxation.
A network optimization approach has been
proposed by (Bookbinder and Reece, 1988), where
they formulate a multi-commodity capacitated
distribution-planning problem as a non-linear mixed
integer programming model, and solve it as a gener-
alized assignment problem within an algorithm for
the overall distribution/routing problem based on a
Bender’s type decomposition.
(Lei et al., 2009) proposes an approach to a bi-
directional flow problem where each iteration starts
with a given planning horizon, which is then parti-
tioned into three planning intervals, where each inter-
val consists of consecutive time periods in the given
ICORES2014-InternationalConferenceonOperationsResearchandEnterpriseSystems
64