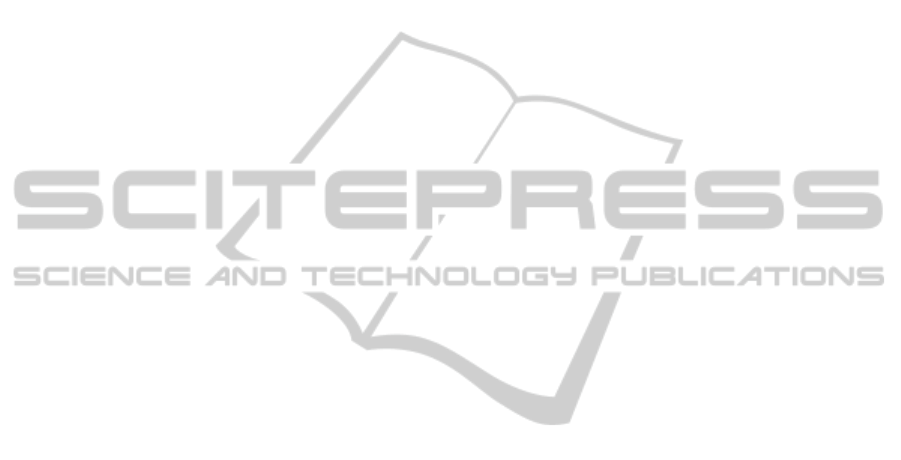
way. Shrinkage estimators shrink the sample covari-
ance matrix towards the identity matrix or diagonal
matrix in the case of the paper. In cases, where the
fitness function requires highly different eigenvalues
and a rotation other than the cartesian coordinate sys-
tem this may be problematic. Therefore, a switch to-
wards the eigenspace of the covariance matrix was
proposed in this paper and investigated in experiments
on the BBOB test suite.
REFERENCES
Audet, C. (2013). A survey on direct search methods
for blackbox optimization and their applications. In
Mathematics without boundaries: Surveys in inter-
disciplinary research. Springer. To appear. Also Les
Cahiers du GERAD G-2012-53, 2012.
Bai, Z. D. and Silverstein, J. W. (1998). No eigenvalues
outside the support of the limiting spectral distribution
of large-dimensional sample covariance matrices. The
Annals of Probability, 26(1):316–345.
Beyer, H.-G. (2001). The Theory of Evolution Strategies.
Natural Computing Series. Springer, Heidelberg.
Beyer, H.-G. and Meyer-Nieberg, S. (2006). Self-adaptation
of evolution strategies under noisy fitness evalua-
tions. Genetic Programming and Evolvable Machines,
7(4):295–328.
Beyer, H.-G. and Schwefel, H.-P. (2002). Evolution strate-
gies: A comprehensive introduction. Natural Com-
puting, 1(1):3–52.
Beyer, H.-G. and Sendhoff, B. (2008). Covariance matrix
adaptation revisited - the CMSA evolution strategy -.
In Rudolph, G. et al., editors, PPSN, volume 5199 of
Lecture Notes in Computer Science, pages 123–132.
Springer.
Chen, X., Wang, Z., and McKeown, M. (2012). Shrinkage-
to-tapering estimation of large covariance matri-
ces. Signal Processing, IEEE Transactions on,
60(11):5640–5656.
Chen, Y., Wiesel, A., Eldar, Y. C., and Hero, A. O.
(2010). Shrinkage algorithms for MMSE covariance
estimation. IEEE Transactions on Signal Processing,
58(10):5016–5029.
Conn, A. R., Scheinberg, K., and Vicente, L. N. (2009).
Introduction to Derivative-Free Optimization. MOS-
SIAM Series on Optimization. SIAM.
Dong, W. and Yao, X. (2007). Covariance matrix repair-
ing in gaussian based EDAs. In Evolutionary Com-
putation, 2007. CEC 2007. IEEE Congress on, pages
415–422.
Eiben, A. E. and Smith, J. E. (2003). Introduction to
Evolutionary Computing. Natural Computing Series.
Springer, Berlin.
Finck, S., Hansen, N., Ros, R., and Auger, A. (2010).
Real-parameter black-box optimization benchmark-
ing 2010: Presentation of the noiseless functions.
Technical report, Institute National de Recherche en
Informatique et Automatique. 2009/22.
Fisher, T. J. and Sun, X. (2011). Improved Stein-type
shrinkage estimators for the high-dimensional mul-
tivariate normal covariance matrix. Computational
Statistics & Data Analysis, 55(5):1909 – 1918.
Hansen, N. (2006). The CMA evolution strategy: A com-
paring review. In Lozano, J. et al., editors, To-
wards a new evolutionary computation. Advances in
estimation of distribution algorithms, pages 75–102.
Springer.
Hansen, N., Auger, A., Finck, S., and Ros, R. (2012).
Real-parameter black-box optimization benchmark-
ing 2012: Experimental setup. Technical report, IN-
RIA.
Hansen, N., Auger, A., Ros, R., Finck, S., and Po
ˇ
s
´
ık, P.
(2010). Comparing results of 31 algorithms from the
black-box optimization benchmarking BBOB-2009.
In Proceedings of the 12th annual conference com-
panion on Genetic and evolutionary computation,
GECCO ’10, pages 1689–1696, New York, NY, USA.
ACM.
Hansen, N. and Ostermeier, A. (2001). Completely deran-
domized self-adaptation in evolution strategies. Evo-
lutionary Computation, 9(2):159–195.
Huber, P. J. (1981). Robust Statistics. Wiley, New York.
Ledoit, O. and Wolf, M. (2004). A well-conditioned estima-
tor for large dimensional covariance matrices. Journal
of Multivariate Analysis Archive, 88(2):265–411.
Ledoit, O. and Wolf, M. (2012). Non-linear shrinkage esti-
mation of large dimensional covariance matrices. The
Annals of Statistics, 40(2):1024–1060.
Meyer-Nieberg, S. and Beyer, H.-G. (2007). Self-adaptation
in evolutionary algorithms. In Lobo, F., Lima, C., and
Michalewicz, Z., editors, Parameter Setting in Evo-
lutionary Algorithms, pages 47–76. Springer Verlag,
Heidelberg.
Rechenberg, I. (1973). Evolutionsstrategie: Optimierung
technischer Systeme nach Prinzipien der biologischen
Evolution. Frommann-Holzboog Verlag, Stuttgart.
Sch
¨
affer, J. and Strimmer, K. (2005). A shrinkage approach
to large-scale covariance matrix estimation and impli-
cations for functional genomics,. Statistical Applica-
tions in Genetics and Molecular Biology, 4(1):Article
32.
Schwefel, H.-P. (1981). Numerical Optimization of Com-
puter Models. Wiley, Chichester.
Stein, C. (1956). Inadmissibility of the usual estimator for
the mean of a multivariate distribution. In Proc. 3rd
Berkeley Symp. Math. Statist. Prob. 1, pages 197–206.
Berkeley, CA.
Stein, C. (1975). Estimation of a covariance matrix. In Rietz
Lecture, 39th Annual Meeting. IMS, Atlanta, GA.
Thomaz, C. E., Gillies, D., and Feitosa, R. (2004). A new
covariance estimate for bayesian classifiers in biomet-
ric recognition. Circuits and Systems for Video Tech-
nology, IEEE Transactions on, 14(2):214–223.
AdaptingtheCovarianceMatrixinEvolutionStrategies
99