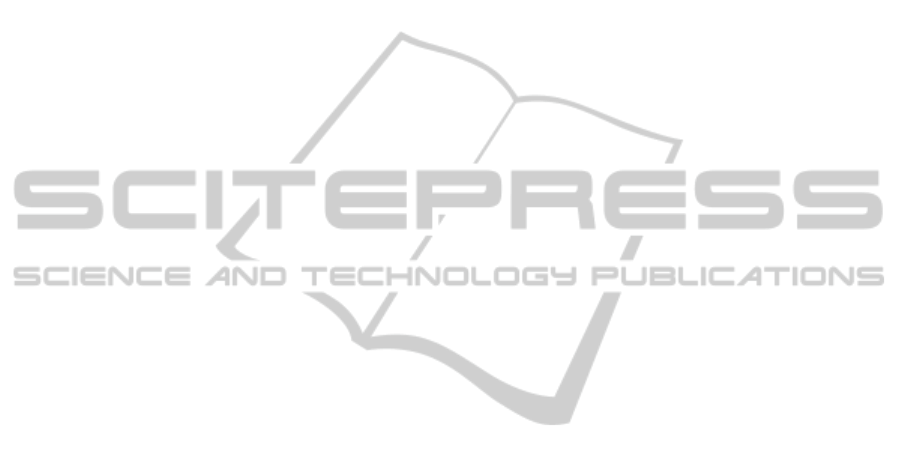
using the same baseline. Third, it is worth exploring
the compatibility of the global MACBETH matrix
with the general formulation of the CI operators for
more than two EC and with other mathematical
formulations, such as with the multilinear one.
Finally, it seems pertinent to exploit the CI
mathematical formulation to model non-monotonic
decision problems which involve not only cardinally
but also ordinally interdependent EC.
ACKNOWLEDGEMENTS
This work was funded by National Funds from the
Portuguese Public Budget through FCT –
Fundação
para a Ciência e a Tecnologia,
within the project
PTDC/EGE-GES/119230/2010.
REFERENCES
Aven, T., 2008. Risk analysis: assessing uncertainties
beyond expected values and probabilities, Wiley.
Bana e Costa C. A., Ensslin L. and Costa A.P., 1998.
Structuring the process of choosing rice varieties at the
south of Brazil. Multi-Criteria Evaluation in Land-Use
Management, Beinat E., Nijkamp P.(eds.), Kluwer
Academic Publishers, Environment & Management. 9:
33-45.
Bana e Costa, C. A. and Beinat, E., 2005. Model-
structuring in public decision-aiding. Working Paper
LSE OR 05.79 – London School of Economics.
Bana e Costa, C. A., Carnero, M. C. and Oliveira, M. D.,
2012. A multi-criteria model for auditing a Predictive
Maintenance Programme. European Journal of
Operational Research 217: 381–393.
Bana e Costa, C. A., Corrêa, E. C., de Corte, J.-M. and
Vansnick, J.-C., 2002. Facilitating bid evaluation in
public call for tenders: a socio-technical approach. The
Internation Journal of Management Science 30: 227-
242.
Bana e Costa, C. A., de Corte, J.-M. and Vansnick, J.-C.,
2012. MACBETH. International Journal of
Information Technology & Decision Making 11(2):
359-387.
Bana e Costa, C. A., Ensslin, L., Corrêa, E. C. and
Vansnick, J.-C., 1999. Decision Support Systems in
action: Integrated application in a multicriteria
decision aid process. European Journal of Operational
Research 113: 315-335.
Bana e Costa, C. A., Lourenço, J. C., Chagas, M. P. and
Bana e Costa, J. C., 2008. Development of reusable
bid evaluation models for the Portuguese Electric
Transmission Company. Decision Analysis 5(1): 22-
42.
Barin, A., Canha, L. N., Abaide, A. R., Orling, R. B. and
Martins, L. F. G., 2012. Selection of renewable energy
technologies in landfills: The M-MACBETH decision
support. Fuelling the Future: Advances in Science and
Technologies for Energy Generation, Transmission
and Storage. A. Mendez-Vilas, Universal-Publishers:
98-102.
Berrah, L. and Clivillé, V., 2007. Towards an aggregation
performance measurement system model in a supply
chain context. Computers in Industry 58: 709-719.
Bottero, M., Ferretti, V. and Mondini, G., 2013. A
Choquet integral-based approach for assessing the
sustainability of a new waste incinerator. International
Journal of Multicriteria Decision Making 3(2/3): 157.
Brosig, J., 2012. Multi-criteria evaluation of classical
swine fever control strategies using Choquet integral
(chapter three). Alternative Classical Swine Fever
control strategies - a simulation study. Agricultural
and Food Sciences. Christian-Albrechts, University of
Kiel. PhD thesis.
Cardin, M., Corazza, M., Funari, S. and Giove, S., 2013.
Building a global performance indicator to evaluate
academic activity using fuzzy measures. Neural Nets
and Surroundings. Apolloni B., Bassis S., Esposito A.
and Morabito F. C., Springer Berlin Heidelberg. 19:
217-225.
Carnero, M. C., 2006. Predictive maintenance programme
audit with MACBETH. Safety and Reliability for
Managing Risk. C. G. Soares and E. Zio, Taylor &
Francis Ltd. 1: 109-115.
Choquet, G., 1953. Theory of capacities. Annales de
l’Institut Fourier 5: 131-295.
Clivillé, V., Berrah, L. and Mauris, G., 2007. Quantitative
expression and aggregation of performance
measurements based on the MACBETH multi-criteria
method. International Journal of Production
Economics 105: 171-189.
Clivillé, V., Mauris, G. and Berrah, L., 2006. A quantified
industrial performance measurement system based on
a Choquet fuzzy integral. IEEE International
Conference on Fuzzy Systems, Vancouver, BC,
Canada.
Edwards, W. and Barron, F.H., 1994. SMARTS and
SMARTER: Improved simple methods for
multiattribute utility measurement. Organizational
Behavior and Human Decision Processes 60: 306-325.
Feyziog
̃
lu, O. and Büyüközkan, G., 2010. Evaluation of
Green Suppliers Considering Decision Criteria
Dependencies. Multiple Criteria Decision Making for
Sustainable Energy and Transportation Systems.
Ehrgott M., Naujoks B, Stewart T.J. and W. J.,
Springer Berlin Heidelberg. 634: 145-154.
Figueiredo, M. S. M. and Oliveira, M. D., 2009.
Prioritizing risks based on multicriteria decision aid
methodology: Development of methods applied to
ALSTOM power. IEEE International Conference on
Industrial Engineering and Engineering Management,
Hong Kong.
Grabisch, M., 1997. k-order additive discrete fuzzy
measures and their representation. Fuzzy Sets and
Systems 92: 167-189.
Grabisch, M., 2005. Une approach constructive de la
UsingMACBETHwiththeChoquetIntegralFundamentalstoModelInterdependenciesbetweenElementaryConcernsin
theContextofRiskManagement
125