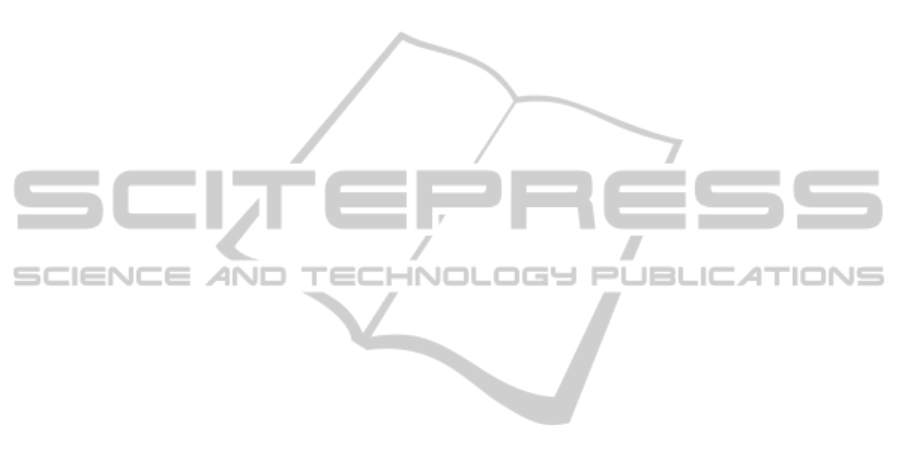
importance. We plan to extend our study to measure
the quality of extracted ‘relations’. It is difficult for
participants to judge relationships from lecture slides
since relations are not highly visible like concepts.
Therefore, we plan to provide extracted concept
maps using IHMC cmap tools to collect feedback on
the ‘strength of extracted relationships’. Lecturers
will also receive conceptual feedback regarding
deficiencies in knowledge organisation of their
courses. This includes disjoint concepts without any
relation to the central concept map and relations
without proper labelling. This process should
improve the legibility of the materials.
6 CONCLUSIONS
The primary challenge of concept map mining is the
lack of a suitable evaluation framework. The
existing approaches utilise human experts’
judgement or expert maps as the gold standard to
measure the quality and validity of machine-
extracted maps. However, these studies focus on
concept existence using IR metrics – precision and
recall, and not the concept ranking according to their
importance. Therefore, this paper proposes a
machine-based evaluation mechanism to assess
mined concept maps in an educational context. We
compared the machine-generated maps with human
judgment and obtained strong positive correlation (r
s
~1) for well-fitted courses.
This work has potential to be utilised as
conceptual feedback for lecturers to have an
overview of knowledge organisation of their
courses. Machine-extracted concept maps require
the assistance of domain experts to validate.
However, this effort is substantially smaller than that
required to construct a concept map manually. In
future work, we plan to provide task-adapted
concept maps instead of hints in intelligent tutoring
environment. This will help students to identify
knowledge gaps and to improve their organisation of
knowledge. We believe that this will help to improve
the depth of meaning that students can extract from
their learning.
REFERENCES
Atapattu, T, Falkner, K. and Falkner, N. 2012. Automated
extraction of semantic concepts from semi-structured
data: supporting computer-based education through
analysis of lecture notes. In proceedings of the 23
rd
International conference on Database and Expert
systems applications, Vienna, Austria.
Alves, A., Pereira, F and Cardoso, F. 2002. Automatic
reading and learning from text. In International
Symposium on Artificial Intelligence.
Ausubel, D., Novak, J. and Hanesian, H. 1978.
Educational psychology: A cognitive view, New York.
Chang, S. N. 2007. Externalising students’ mental models
through concept maps. Journal of Biological
Education.
Chen, N., Kinshuk, and Wei, C. 2008. Mining e-learning
domain concept map from academic articles,
Computer and Education.
Coffey, J., Carnot, M., Feltovich, P., Feltovich, J.,
Hoffman, R., Canas, A. and Novak, J. 2003. A
summary of literature pertaining to the use of concept
mapping techniques and technologies for education
and performance support, The Chief of Naval
Education and Training.
Dali, L., Rusu, D., Fortuna, B., Mladenic, D., Grobelnik,
M. 2009. Question answering based on Semantic
graphs. In Language and Technology Conference.
Poznan, Poland.
Frantzi, K., Ananiadou, S. and Mima, H. 2000. Automatic
recognition of multi-word terms: the C-value/NC-
value method. International Journal on Digital
Libraries.
Gantayat, N and Iyer, S. 2011. Automated building of
domain ontologies from lecture notes in courseware.
In Proceedings of the IEEE international conference
on Technology for education, India.
Gouli, E., Gogoulou, A., Papanikolaou, K. and
Grigoriadou, M. 2004. COMPASS: An adaptive web-
based concept map assessment tool. In Proceedings of
the first international conference on concept mapping.
Hearst, M. A., 2000. The debate on automated essay
grading. Intelligent systems and their Applications.
Kinchin, I. 2006. Developing PowerPoint handouts to
support meaningful learning. British Journal of
Education technology. 37 (4), 647-650.
Leake, D., Maguitman, A. and Reichherzer, T. 2004.
Understanding Knowledge Models: Modelling
Assessment of Concept Importance in Concept Maps.
In Proceedings of CogSc.
Maass, J., Pavlik, P. 2013. Utilising Concept mapping in
Intelligent Tutoring Systems. In Artificial Intelligence
in Education.
Novak, J. and Canas, A. 2006. The theory underlying
Concept maps and How to construct and use them.
Institute of Human and Machine Cognition.
Novak, J. and Gowin, D. 1984. Learning how to learn.
Cambridge University Press, New York and
Cambridge.
Olney, A. M., Graesser, A. and Person, N. 2012. Question
generation from Concept maps. Special issue on
Question generation, Dialogue and Discourse.
Ono, M., Harada, F. and Shimakawa, H. 2011. Semantic
network to formalise Learning items from Lecture
notes. International Journal of Advanced Computer
Science.
Starr, R. and Oliveira, J. 2013. Concept maps as the first
EvaluationofConceptImportanceinConceptMapsMinedfromLectureNotes-ComputerVsHuman
83