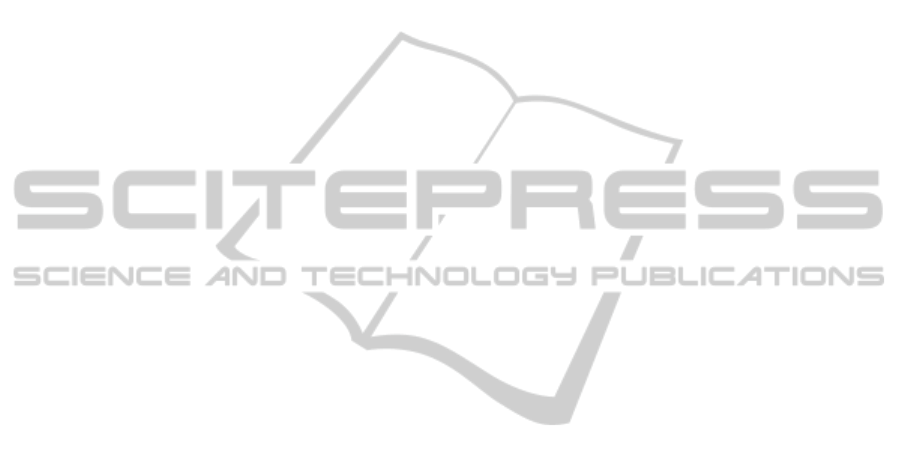
6 CONCLUSIONS
Beginning from the first half of 90’s, recommender
system research continues to grow in different appli-
cation domains. Nowadays it is not hard to see a rec-
ommender system working in the background of the
web site you have visited and recommends you music
or shopping items. Even though these useful appli-
cations of recommender systems exist, there are still
many challenges for a true personalized recommender
system. As the number of online news sources in-
creases it becomes harder for an end user to find what
she is looking for. Thus the need for a news recom-
mender system increases.
In this paper, the challenges and different meth-
ods of news recommendation domain are presented. It
is pointed out which approaches solve different chal-
lenges and how they do this. Our current frame-
work for comparing recommender systems in news
domain deals with content-based, collaborative and
hybrid recommendation approaches, though we in-
tend to expand it with recent results from semantically
based recommender systems. Including semantic rep-
resentations in the recommendation process helps us
to understand user needs and news content and can
be valuable when several of the challenges above are
addressed.
REFERENCES
Adomavicius, G. and Tuzhilin, A. (2005). Toward the
next generation of recommender systems: A survey of
the state-of-the-art and possible extensions. Knowl-
edge and Data Engineering, IEEE Transactions on,
17(6):734–749.
Borges, H. L. and Lorena, A. C. (2010). A survey on rec-
ommender systems for news data. In Smart Infor-
mation and Knowledge Management, pages 129–151.
Springer.
Burke, R. (2002). Hybrid recommender systems: Survey
and experiments. User modeling and user-adapted in-
teraction, 12(4):331–370.
Cantador, I., Bellog
´
ın, A., and Castells, P. (2008).
News@hand: A semantic web approach to recom-
mending news. In Proceedings of the 5th interna-
tional conference on Adaptive Hypermedia and Adap-
tive Web-Based Systems, AH ’08, pages 279–283,
Berlin, Heidelberg. Springer-Verlag.
Das, A., Datar, M., Garg, A., and Rajaram, S. (2007).
Google news personalization: Scalable online collab-
orative filtering. In WWW ’07 Proceedings of the 16th
international conference on World Wide Web, pages
271–280.
Fortuna, B., Fortuna, C., and Mladenic, D. (2010). Real-
time news recommender system. In ECML PKDD’10
Proceedings of the 2010 European conference on Ma-
chine learning and knowledge discovery in databases:
Part III, pages 583–586.
Garcin, F., Dimitrakakis, C., and Faltings, B. (2013). Per-
sonalized news recommendation with context trees.
CoRR, abs/1303.0665.
Iaquinta, L., Gemmis, M. D., Lops, P., Semeraro, G., Filan-
nino, M., and Molino, P. (2008). Introducing serendip-
ity in a content-based recommender system. In Hy-
brid Intelligent Systems, 2008. HIS’08. Eighth Inter-
national Conference on, pages 168–173. IEEE.
Lee, H. and Park, S. J. (2007). Moners: A news recom-
mender for the mobile web. Expert Systems with Ap-
plications, 32(1):143–150.
Li, L., Wang, D., Li, T., Knox, D., and Padmanabhan, B.
(2011). Scene : A scalable two-stage personalized
news recommendation system. In SIGIR ’11 Pro-
ceedings of the 34th international ACM SIGIR con-
ference on Research and development in Information
Retrieval, pages 125–134.
Lin, C., Xie, R., Li, L., Huang, Z., and Li, T. (2012).
Premise: personalized news recommendation via im-
plicit social experts. In Proceedings of the 21st ACM
international conference on Information and knowl-
edge management, pages 1607–1611. ACM.
Liu, J., Dolan, P., and Pedersen, E. R. (2010). Personalized
news recommendation based on click behavior. In IUI
’10 Proceedings of the 15th international conference
on Intelligent user interfaces, pages 31–40.
Resnick, P., Iacovou, N., Suchak, M., Bergstrom, P., and
Riedl, J. (1994). Grouplens: an open architecture for
collaborative filtering of netnews. In Proceedings of
the 1994 ACM conference on Computer supported co-
operative work, pages 175–186. ACM.
Saranya.K.G and Sadhasivam, G. (2012). A personalized
online news recommendation system. International
Journal of Computer Applications, 57(18):6–14.
Sarwar, B., Karypis, G., Konstan, J., and Riedl, J. (2001).
Item-based collaborative filtering recommendation al-
gorithms. In Proceedings of the 10th international
conference on World Wide Web, pages 285–295.
ACM.
Su, X. and Khoshgoftaar, T. M. (2009). A survey of col-
laborative filtering techniques. Advances in artificial
intelligence, 2009:4.
Tavakolifard, M., Gulla, J. A., Almeroth, K. C., Ingvaldesn,
J. E., Nygreen, G., and Berg, E. (2013). Tailored news
in the palm of your hand: a multi-perspective transpar-
ent approach to news recommendation. In Proceed-
ings of the 22nd international conference on World
Wide Web companion, pages 305–308. International
World Wide Web Conferences Steering Committee.
Wen, H., Fang, L., and Guan, L. (2012). A hybrid approach
for personalized recommendation of news on the web.
Expert Systems with Applications, 39(5):5806–5814.
Yeung, K. F. and Yang, Y. (2010). A proactive personal-
ized mobile news recommendation system. In De-
velopments in E-systems Engineering, pages 207–212.
IEEE.
ASurveyonChallengesandMethodsinNewsRecommendation
285