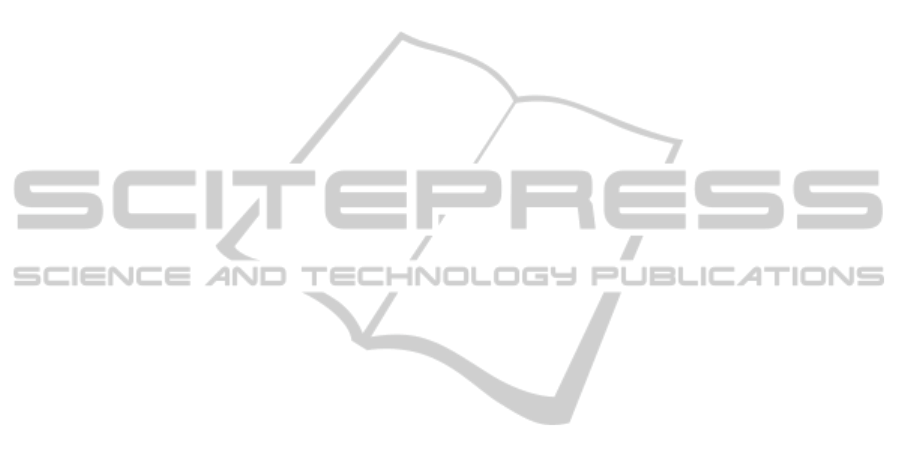
narrow (M1, M4), low (M2, M3, M4), high (M1),
all describing salient features of the four dimensional
FCM data. These 4D modes are marked in one 2D
marginal biplot in Fig. 3. To a varying degree, these
modes are visible also in other biplots but the voluplot
representation is much clearer.
A more detailed view of the modes and their posi-
tions is provided by the four baryplots in Figs. 4d–
g. As in the voluplot, the highest level set M1 is
easily identified. We may conclude that this nearly
symmetric and narrow mode actually corresponds to
the monodisperse 3 µm calibration particle popula-
tion. Symmetry and relative narrowness indicate that
the optomechanical measurement settings in the FCM
unit functioned well. Likewise, the narrow, smaller
mode M4 can be identified as the 1 µm calibration
particle population.
The wide mode M3 (see Fig. 4c) clearly represents
fines, and the slightly narrower M2 is caused by pitch.
The skewness of M2 and M3 can be observed both
from the scatterplot matrix and from the baryplots,
but the baryplots quantify the degree and direction of
skewness better.
Finally, the marginal densities added to the bary-
plots in Figs. 4d–g complement the LST plots in use-
ful way, facilitating the interpretation of baryplots.
4.2.3 Density based Parallel Coordinate Plot
To compare the LST method with a more familiar
multivariate visualization, we also created a density-
based parallel coordinate plot of the FCM data
(Fig. 5) as defined in Miller and Wegman (1991).
The kernel density estimator was used with the stan-
dard Gaussian kernel and smoothing parameter h =
0.0012. We calculated 3000 univariate estimates, and
evaluated each estimate on an equispaced grid of 1000
points. A subsample of 100000 observations was
used in the density plot.
In Fig. 5 the narrow modes M1, M2, and M4 can
be distinguished and associated with the correspond-
ing modes in Fig. 4. However, the wide mode M3,
which is clearly apparent in the LST plots, is not eas-
ily observed in the PC plot. M3 appears as a smoothly
varying background in the density-based PCP.
On the other hand, PCP suggests a mode Mx not
reported in the LST analysis. Still, Mx can be visually
observed in the enhanced baryplots as a shoulder of
the marginal near M1, although not as a mode. This
borderline case is due to the limited spatial resolution
in the 4D density and level set calculations. At the
same time it brings out issues in the LST visualization
scheme that need to be addressed in future research,
namely the need for efficient partitioning of the space
as dimension increases.
Density based PCP nicely shows the connections
of the modes in different dimensions. However, the
structure of the modes, volume, shape, skewness, kur-
tosis, etc., cannot be observed as clearly as in the LST
plots. In addition, the order of variables in the PCP
may bias the inference to some extent as opposed to
the equal treatment of the variables in the LST plots.
5 DISCUSSION
We have applied level set tree based methods to 4D
flow cytometry data. In the four dimensional case
it is possible to estimate density functions quite ac-
curately, while the more traditional methods using
marginal densities and slices are already difficult to
use in this setting, and the difficulties would rapidly
multiply if higher dimensional data were considered.
5.1 Observations – 2D or 4D
For our data, the modes can be detected both with the
scatter plot matrix (2D) and with the LST plots (4D).
However, the information provided by these methods
is different. The scatter plot matrix is based on the
histogram estimates of the two dimensional marginal
densities, whereas the LST plots are based on the ker-
nel estimates of the four dimensional density. Thus,
the LST plots visualize the concentration of the prob-
ability mass in the 4D space instead of visualizing the
concentration of the probability mass through projec-
tions to the 2D space, as is done in the scatter plot
matrix. The existence and the location of the modes
can be seen in the scatter plot matrix but the LST plots
show estimates of the full mode structure of the un-
derlying density. This means that we see estimates
of the size of the modes and estimates on the spread
of the probability mass associated with the modes.
The scatter plots give indications of these properties,
but since they show data projected to two dimensions
(estimates of 2D marginal densities), we cannot in-
fer the size of the modes and the spread of the prob-
ability mass in the 4D space. In FCM data analy-
sis, the LST methods can simultaneously identify and
quantify features of multidimensional particle clusters
from large and dominating modes down to small and
easily missed concentrations. This feature may prove
to be highly valuable for example in medical FCM
data exploration.
There are examples where the modes can be de-
tected with the LST plots but not with the scatter
plot matrices. This can happen when the modes are
so close to each other that they mask each other in
projections, see Klemel¨a (2004). On the other hand,
IVAPP2014-InternationalConferenceonInformationVisualizationTheoryandApplications
216