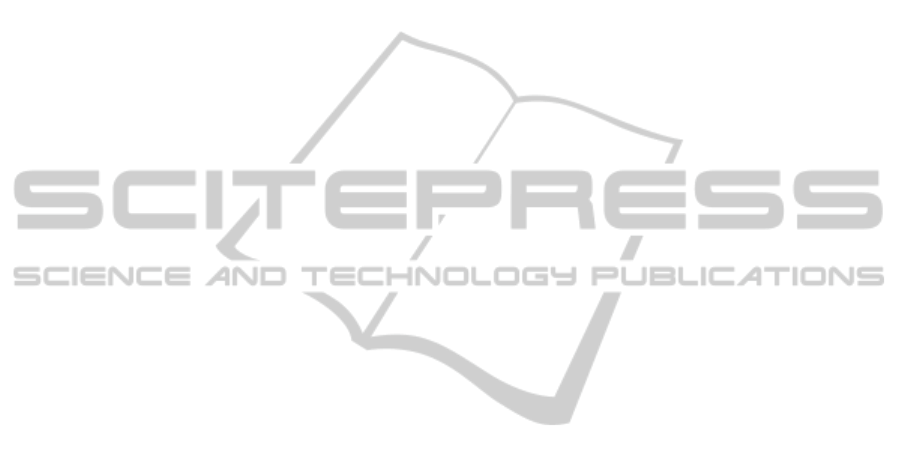
5 CONCLUSIONS AND NEXT
STEPS
The extension of the conventinal CEP architecture by
decoupled dynamic partitions overcomes the problem
of limited flexibility due to pre-partitioning. It thereby
allows for applying the proposed DCEP approach to
highly dynamic and high volume DLM scenarios. By
seperating simple CEP pattern matching tasks from
dynamically changing complex analysis tasks, we in-
crease the computing efficiency of DLM processing.
In a next step, the prototypical realization of the
DCEP architecture will be evaluated using several
data sets of AMI data from a smart grid test field
in northwestern Switzerland. The provided PV mea-
surement data will be combined with simulated cloud
movement data, based on empirically tested assump-
tions about the signal behaviour of the PV devices
under the effect of cloud shading. Existing studies
show that PV arrays under partially shaded conditions
exhibit characteristic signal patterns, cf., e.g., (Ve-
muru et al., 2012). These signal characteristics can be
used for cloud recognition when combined with back-
ground domain knowledge on typical cloud shapes,
shape change, movement patterns and the geographic
charactersitics of PV locations that influence the shad-
ing patterns. Other challenges that must be addressed
in the generalized DCEP framework design such as
the fuzzy matching of time lags between situations
of interest will be addressed in future work, cf. also
(Schaaf, 2013).
ACKNOWLEDGEMENTS
Parts of the work presented here were done under Eu-
rostars Project E!7377.
REFERENCES
Dam, Q., Mohagheghi, S., and Stoupis, J. (2008). Intelli-
gent demand response scheme for customer side load
management. In Energy 2030 Conference, 2008. EN-
ERGY 2008. IEEE, pages 1–7.
Huang, Z. and Nieplocha, J. (2008). Transforming power
grid operations via high performance computing. In
Power and Energy Society General Meeting - Con-
version and Delivery of Electrical Energy in the 21st
Century, 2008 IEEE, pages 1–8.
Koch, S., Barcenas, F. S., and Andersson, G. (2010). Using
controllable thermal household appliances for wind
forecast error reduction. In Ferrera, P. M., editor, IFAC
Conference on Control Methodologies and Technol-
ogy for Energy Efficiency, volume 1, pages 261–266.
IFAC.
Koch, S., Meier, D., Zima, M., Wiederkehr, M., and Ander-
sson, G. (2009). An active coordination approach for
thermal household appliances - local communication
and calculation tasks in the household. In PowerTech,
2009 IEEE Bucharest, pages 1–8.
Lu, N. and Hammerstrom, D. (2006). Design considerations
for frequency responsive grid friendlytm appliances.
In Transmission and Distribution Conference and Ex-
hibition, 2005/2006 IEEE PES, pages 647–652.
Molderink, A., Bakker, V., Bosman, M. G. C., Hurink, J.,
and Smit, G. J. M. (2010). Management and control
of domestic smart grid technology. Smart Grid, IEEE
Transactions on, 1(2):109–119.
Ringwood, J. V., Bofelli, D., and Murray, F. T. (2001). Fore-
casting electricity demand on short, medium and long
time scales using neural networks. J. Intell. Robotics
Syst., 31(1-3):129–147.
Schaaf, M. (2013). Event processing with dynamically
changing focus. To be published in the Proceedings
of the Seventh IEEE International Conference on Re-
searcg Challenges in Information Science 2013 (RCIS
2013).
Vemuru, S., Singh, P., and Niamat, M. (2012). Anal-
ysis of photovoltaic array with reconfigurable mod-
ules under partial shading. In Photovoltaic Specialists
Conference (PVSC), 2012 38th IEEE, pages 001437–
001441.
Vovos, P., Kiprakis, A., Wallace, A., and Harrison, G.
(2007). Centralized and distributed voltage control:
Impact on distributed generation penetration. Power
Systems, IEEE Transactions on, 22(1):476–483.
Yin, J., Kulkarni, A., Purohit, S., Gorton, I., and Akyol, B.
(2011). Scalable real time data management for smart
grid. In Proceedings of the Middleware 2011 Industry
Track Workshop, Middleware ’11, pages 1:1–1:6, New
York, NY, USA. ACM.
IntelligentDynamicLoadManagementBasedonSolarPanelMonitoring
81