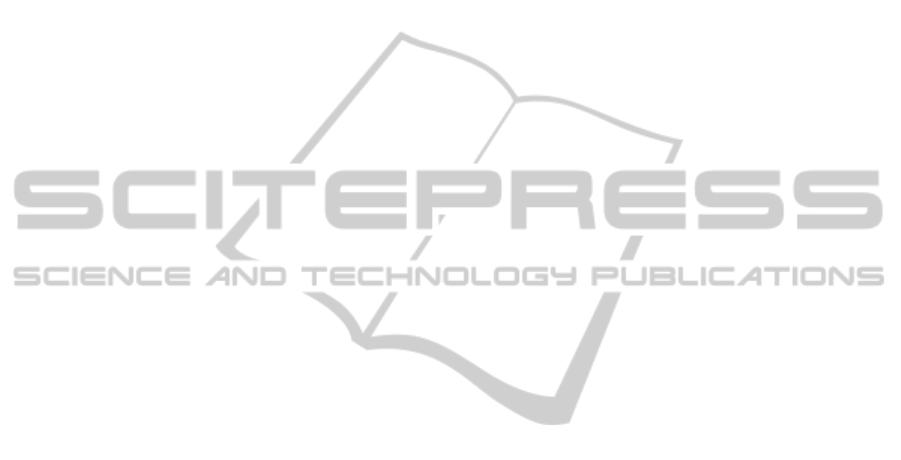
“Projet Voltaire”, a web-based environment to learn
French spelling. For the next step, we will plug the
gamification system on other learning environments,
in order to evaluate its genericity.
An experiment will allow us to evaluate the
system described in this paper. For instance, we plan
to compare the automatic adaptation with the
“home made” adaptation: what happens if the user
can choose the new functionalities by himself? In
order to evaluate the gamification adaptation to
users’ profiles, we plan to compare three cases:
Case 1: Selecting game elements according to
user’s profile.
Case 2: Selecting game elements randomly.
Case 3: Selecting game elements at the
opposite of user’s profile.
In this way, we will be able to evaluate the
relevance of the proposed adaptation (case 1).
Furthermore, some improvements will concern
the flexibility of the player model. Sometimes, the
player type is not enough to model the user’s needs,
as they can change during the day. As an example,
two motivational factors of Lazzaro (2004) are
detailed bellow:
Hard fun (Players look for challenge, strategy
and problem solving).
Easy fun (Players enjoy intrigue and
curiosity).
Whether we expect to relax (easy fun) or to be
challenged (hard fun) depends more on our mood
than our personality and player type. Some
contextual information can help to know about this
mood, like the hour and the day. For example, a user
may expect a more relaxing activity after lunch.
Furthermore, expert systems are limited as they are
static. Another improvement we plan to do is the use
of machine learning techniques to automatically
adapt the adaptation rules themselves, based on the
experience with the previous users.
REFERENCES
Andrade G., Ramalho G., Gomes A. S., Corruble V.,
2006. Dynamic Game Balancing: an Evaluation of
User Satisfaction, in: Proceedings of the 2nd Artificial
Intelligence and Interactive Digital Entertainment
Conference (AIIDE06), AAAI Press.
Bartle, R., 1996. Hearts, Clubs, Diamonds, Spades: Players
Who Suit MUDs. Journal of MUD Research 1, 1.
Beck, J., 2005. Engagement tracing: using response times
to model student disengagement. Artificial intelligence
in education: Supporting learning through intelligent
and socially informed technology 125, 88.
Bernardini, A., Conati, C., 2010. Discovering and
recognizing student interaction patterns in exploratory
learning environments, in: Intelligent Tutoring
Systems. pp. 125–134.
Bouvier, P., Lavoué, E., Sehaba, K., George, S., 2013.
Identifying Learner’s Engagement in Learning Games:
a Qualitative Approach based on Learner’s Traces of
Interaction, in: 5th International Conference on
Computer Supported Education (CSEDU 2013),
Aachen, Germany. pp. 6–8.Brusilovsky 2001.
Brusilovsky, P., 2001. Adaptive hypermedia. User
modeling and user-adapted interaction 11 (1-2): 87-
110.
Charlier, N., Michela, O., Remmele, B., Whitton, N.,
2012. Not Just for Children: Game-Based Learning for
Older Adults, in: 6th European Conference on Games
Based Learning, Cork, Ireland. pp. 102–108.
Cheng, T. I. C., n.d. Cheng, L.-T., Shami, S., Casey, D.,
Muller, M., DiMicco, J., Patterson, J., others, 2011.
Finding moments of play at work, in: CHI 2011
Workshop (2–5). Vancouver: Canada.
Cocea, M., 2006. Assessment of motivation in online
learning environments, in: Adaptive Hypermedia and
Adaptive Web-Based Systems. pp. 414–418.
Conati, C., Manske, M., 2009. Evaluating adaptive
feedback in an educational computer game, in:
Intelligent Virtual Agents. pp. 146–158.
Deterding, S., Dixon, D., Khaled, R., Nacke, L., 2011.
From game design elements to gamefulness: defining
gamification, in: Proceedings of the 15th International
Academic MindTrek Conference: Envisioning Future
Media Environments. pp. 9–15.
Eglesz, D., Fekete, I., Kiss, O. E., Izso, L., 2005.
Computer games are fun? On professional games and
players’ motivations. Educational Media International
42, 117–124 (in Hainey, T., Connolly, T., Stansfield,
M., Boyle, E., 2011. The differences in motivations of
online game players and offline game players: A
combined analysis of three studies at higher education
level. Computers & Education 57, 2197–2211.).
Ferro, L. S., Walz, S. P., Greuter, S., 2013. Towards
personalised, gamified systems: an investigation into
game design, personality and player typologies, in:
Proceedings of The 9th Australasian Conference on
Interactive Entertainment: Matters of Life and Death.
p. 7.
Gamification Co, 2013, A New Perspective on the Bartle
Player Types for Gamification - GCo [WWW
Document], n.d. URL http://www.gamification.co/
2013/08/12/a-new-perspective-on-the-bartle-player-
types-for-gamification/ (accessed 10.1.13).
Giroux, S., Pachet, F., Paquette, G., Girard, J., 1995. Des
systèmes conseillers épiphytes. Revue d’intelligence
artificielle 9, 165–190.
Hocine, N., Gouaïche, A., Di Loreto, I., Abrouk, L., 2011.
Techniques d´adaptation dans les jeux ludiques et
sérieux. Revue d’intelligence artificielle 25, 253–280.
KhanAcademy, 2006. www.khanacademy.org.
Kobsa, A., Koenemann, J., Pohl, W., 2001. Personalised
hypermedia presentation techniques for improving
CSEDU2014-6thInternationalConferenceonComputerSupportedEducation
124