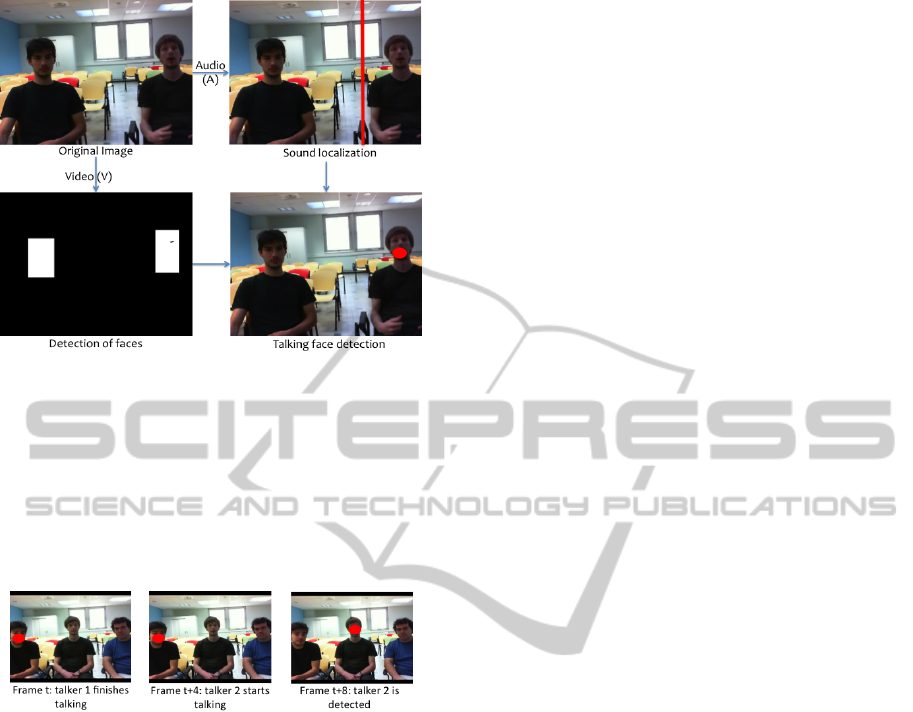
Figure 7: Results of audiovisual data fusion for a conversa-
tion involving 2 speakers.
• The time of response when the speaker change is
no more than a few frames (around 4) before the
detection of the new speaker (figure 8).
• In the different videos that were tested the speaker
is always detected after some time
Figure 8: Detection of a new speaker among 3 persons after
a small delay.
6 CONCLUSIONS
This work focuses on three main issues: produce de-
tection methods on low-quality visual and audio data
from low-cost sensors, elaborate a robust audiovisual
data fusion method adapted to the situation, and make
the method follow a real-time constraint.
A full processing chain has been elaborated: it re-
lies on the independent processing of the audio stream
(sound source localization) and the video stream (face
detection), fused in a late fusion process to create the
decision. One of the main advantages of this process-
ing chain is that each of its links can be modified and
upgraded in future works. There are perspectives to
improve this processing chain: the robot should be
able to differentiate two speakers speaking at the same
time at this stage, and the detection process can be
improved by dealing with the bias introduced in the
coordinate system. A lot of improvement could come
from the inclusion of new sensors such as depth sen-
sors or laser sensors to discriminate between region of
interests to be explored directly instead of exploring
the whole frame at each time. Improvement can also
be made from using motion in the video, instead of
frame-by-frame processing.
This work has been partially supported by the
LabEx PERSYVAL-Lab (ANR-11-LABX-0025).
REFERENCES
Brandstein, M. S. and Silverman, H. F. (1997). A practi-
cal methodology for speech source localization with
microphone arrays. Computer Speech & Language.
Chai, D. and Ngan, K. (1998). Locating facial region of a
head-and-shoulders color image. In Automatic Face
and Gesture Recognition. Proc.
Farmer, M. E., Hsu, R., and Jain, A. K. (2002). Interacting
multiple model kalman filters for robust high speed
human motion tracking. ICPR’02.
Gustafsson, F. and Gunnarsson, F. (2003). Positioning
using time-difference of arrival measurements. In
ICASSP’03.
Hospedales, T. M. and Vijayakumar, S. (2008). Structure
inference for bayesian multisensory scene understand-
ing. IEEE Transactions on Pattern Analysis and Ma-
chine Intelligence.
Nguyen, Q. and Choi, J. (2010). Audio-visual data fusion
for tracking the direction of multiple speakers. In Con-
trol Automation and Systems.
Osuna, E., Freund, R., and Girosi, F. (1997). Training sup-
port vector machines: an application to face detection.
In CVPR’97.
Rao, B. D. and Trivedi, M. M. (2008). Multimodal infor-
mation fusion using the iterative decoding algorithm
and its application to audio-visual speech recognition.
In Acoustics, Speech, and Signal Processing, 2008.
Schneiderman, H. and Kanade, T. (1998). Probabilistic
modeling of local appearance and spatial relationships
for object recognition. In CVPR’98.
Snoek, C. G. M. (2005). Early versus late fusion in semantic
video analysis. ACM Multimedia.
Thrun, S., Burgard, W., and Fox, D. (2005). Probabilis-
tic Robotics (Intelligent Robotics and Autonomous
Agents). The MIT Press.
Vaillant, R., Monrocq, C., and Le Cun, Y. (1994). Origi-
nal approach for the localisation of objects in images.
Vision, Image and Signal Processing, IEEE Proc.
Valin, J.-M., Michaud, F., Hadjou, B., and Rouat, J. (2004).
Localization of simultaneous moving sound sources
for mobile robot using a frequency- domain steered
beamformer approach. In ICRA’04.
Viola, P. and Jones, M. (2004). Robust real-time face detec-
tion. IJCV’04.
Zhang, C. and Zhang, Z. (2010). A survey of recent ad-
vances in face detection.
AudiovisualDataFusionforSuccessiveSpeakersTracking
701