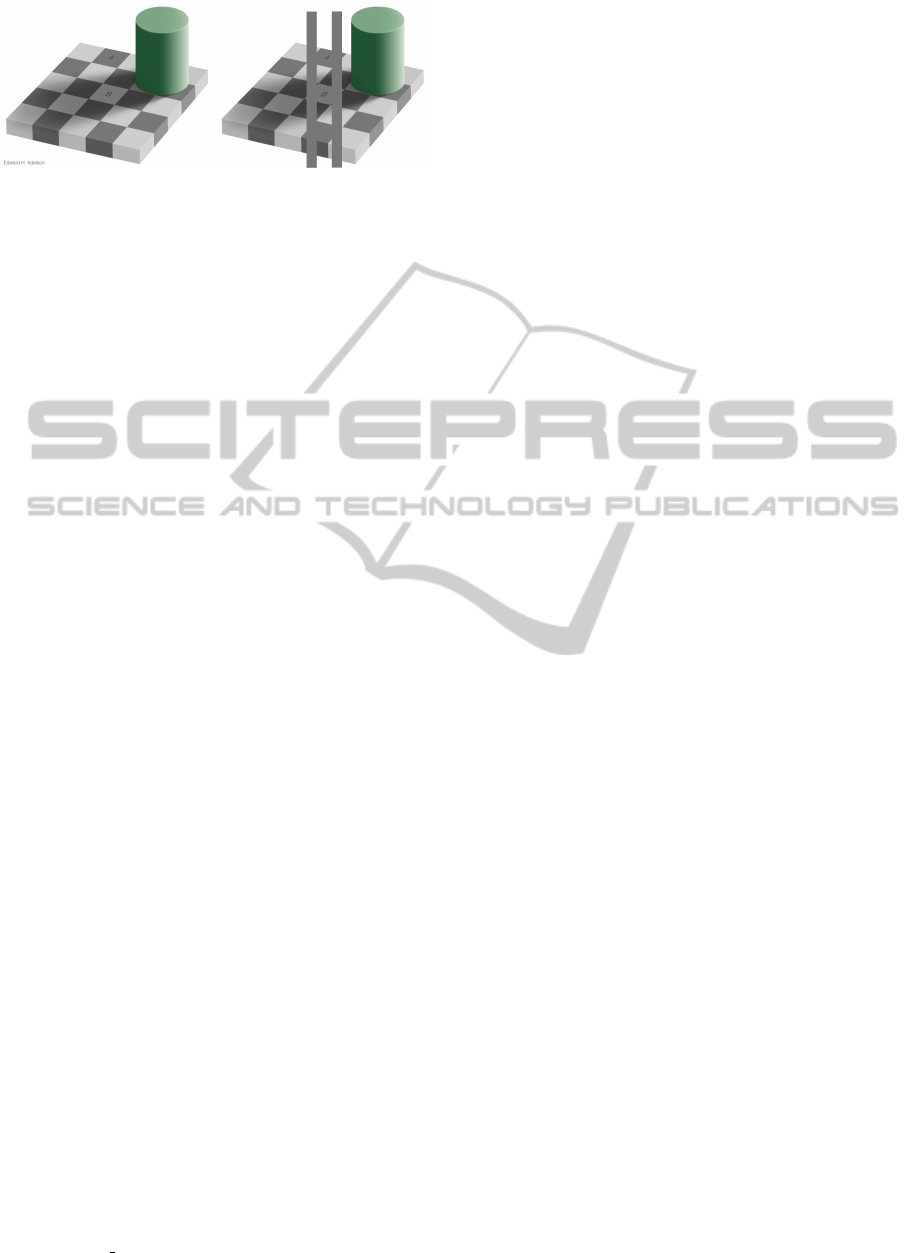
Figure 4: Demonstration of the checkerboard illusion.
gests wrong or implausible inferences. As a compo-
nent of a theory of visualization, an evaluation method
will ensure all these issues are in some way addressed.
5 SUMMARY
There is still much to say about how a multi-layer
theory of visualization should be structured, and how
the properties at one level are selected and preserved
when mapped to the subsequent layer. Indeed, dy-
namic visual analytics is about how direct manipula-
tion of a picture can be constrained by the next lower
level so that users exploring the properties of a picture
are constrained to make only plausible adjustments to
that picture (cf. (Cooper et al., 2010)).
But the primary value of such a theory is to
articulate principles, which are typically domain-
dependent, for the multi-layer mappings from base
data to picture. This ensures that anomalies are not
created in the mappings, and that the resulting pic-
tures can be evaluated with respect to their inferential
value. In that regard, evaluation must focus on how
alternative mappings to pictures make accurate con-
strained inference easy or difficult.
ACKNOWLEDGEMENTS
This research is supported in part by AICML, iCORE,
NSERC, AITF, and the Meme Lab of Hokkaido Uni-
versity. I am grateful for continued discussions on all
aspects of visualization with Wei Shi, Yuzuru Tanaka,
Walter Bischof, Christopher Culy, Jonas Sjobergh,
and Bob Spence. All misconceptions and misrepre-
sentations remain my own.
REFERENCES
Adelson, E. H. (1995). The checker shadow illu-
sion. see /web.mit.edu/persci/people/adelson/ check-
ershadow illusion.html.
Bertschi, S., Bresciani, S., Crawford, T., Goebel, R., Kien-
reich, W., Lindner, M., Sabol, V., and Moere, A. V.
(2011). What is knowledge visualization? perspec-
tives on an emerging discipline. 2010 14th Interna-
tional Conference Information Visualisation, 0:329–
336.
Card, S. K. (2012). Information visualization. In Jacko,
J. A., editor, Human-Computer Interaction Hand-
book: Fundamentals, Evolving Technologies, and
Emerging Applications, 3rd Edition, pages 515–545.
CRC Press.
Card, S. K., Mackinlay, J. D., and Shneiderman, B. (1999).
Readings in information visualization - using vision to
think. Academic Press.
Cooper, S., Treullie, A., Barbero, J., Leaver-Fay, A., Tuite,
K., Khatib, F., Snyder, A. C., Beenen, M., Salesin, D.,
Baker, D., and Popovi
´
c, Z. (2010). Predicting pro-
tein structures with a multiplayer online game. Na-
ture, 466:756–760.
Fagnan, J., Za
¨
ıane, O. R., and Goebel, R. (2012). Visualiz-
ing community centric network layouts. In Banissi, E.,
Bertschi, S., Burkhard, R. A., Cvek, U., Eppler, M. J.,
Forsell, C., Grinstein, G. G., Johansson, J., Kender-
dine, S., Marchese, F. T., Maple, C., Trutschl, M., Sar-
fraz, M., Stuart, L. J., Ursyn, A., and Wyeld, T. G., ed-
itors, Information Visualization 2012, pages 321–330.
IEEE Computer Society.
Goebel, R., Shi, W., and Tanaka, Y. (2013). The role of di-
rect manipulation of visualizations in the development
and use of multi-level knowledge models. In 17th In-
ternational Conference on Information Visualization,
pages 325–332. IEEE Computer Society.
May, R., Hanrahan, P., Keim, D., Shneiderman, B., and
Card, S. (2010). The state of visual analytics: Views
on what visual analytics is and where it is going. In Vi-
sual Analytics Science and Technology (VAST), 2010
IEEE Symposium on, pages 257 –259.
Patterson, R., Liggett, K., Blaha, L., Grinstein, G., Havig,
P., Kaveney, D., Moore, J., and Sheldon, K. (2013).
A theory and model of visualization. International
Journal of Computer Graphics and Visualization.
(preprint).
Tufte, E. R. (1983). The visual display of quantitative in-
formation. Graphics Press.
ASketchofaTheoryofVisualization
221