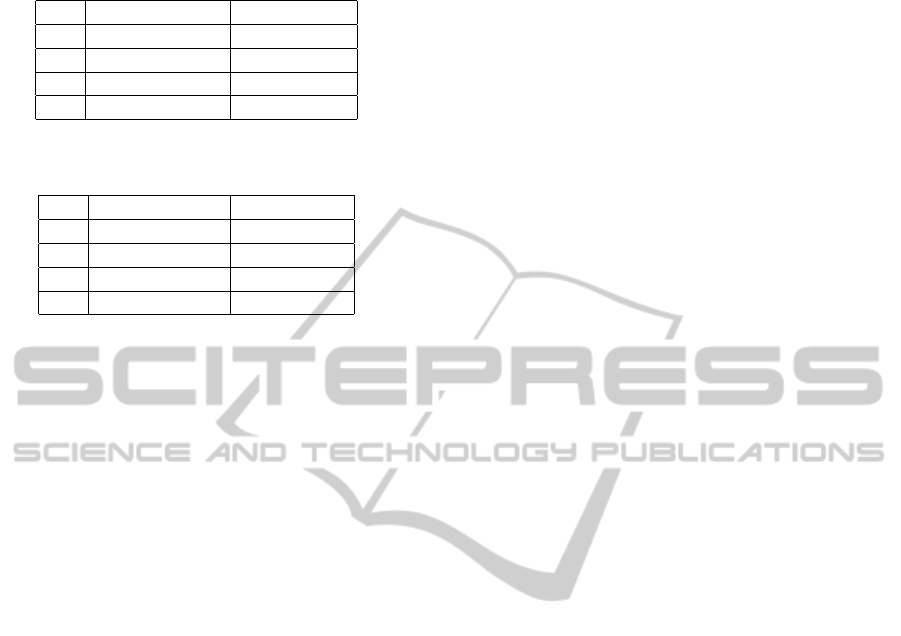
Table 7: L
∞
Error on the image with L–model and ON–
model related to the vase Test.
σ L
∞
Error Lamb L
∞
Error ON
0 0.808202 0.808202
0.2 0.808202 0.766265
0.4 0.808202 0.678274
0.5 0.808202 0.634672
Table 8: L
1
Error on the image with L–model and ON–
model related to the vase Test.
σ L
1
Error Lamb L
1
Error ON
0 0.028919 0.028919
0.2 0.028919 0.027292
0.4 0.028919 0.023764
0.5 0.028919 0.022190
the differences in terms of computational complexity
and accuracy. We also plan to compare the results for
the orthographic projection and the perspective pro-
jection model introduced in (Ju et al., 2013).
5 CONCLUSIONS
The non-Lambertian models lead to rather complex
nonlinear PDEs of the first order which can be treated
in the framework of weak (viscosity) solutions. The
analysis of this models shows that they are not able
to resolve the well known convex/concave ambigu-
ity despite the fact that they can deal with more gen-
eral surfaces. From the numerical point of view, these
equations can be approximated via semi-Lagrangian
techniques in a rather effective way. The role of the
roughness parameter σ is crucial to obtain accurate
results, playing with this parameter can in fact im-
prove the approximation with respect to the classical
L–model. In this respect, the non-Lambertian frame-
work is more flexible and effective.
REFERENCES
Breuß, M., Cristiani, E., Durou, J.-D., Falcone, M., and Vo-
gel, O. (2012). Perspective shape from shading: Am-
biguity analysis and numerical approximations. SIAM
J. Imaging Sciences, 5(1):311–342.
Courteille, F., Crouzil, A., Durou, J. D., and Gurdjos, P.
(2004). Towards shape from shading under realistic
photographic conditions. 17th International Confer-
ence on Parttern Recognition, pages 277–280.
Durou, J., Falcone, M., and Sagona, M. (2008). Numerical
methods for shape from shading: a new survey with
benchmarks computer vision and image understand-
ing. Elsevier, 109(1):22–43.
Falcone, M. and Ferretti, R. (2013). Semi-Lagrangian Ap-
proximation Schemes for Linear and Hamilton-Jacobi
Equations. SIAM.
Falcone, M., Sagona, M., and Seghini, A. (2003). A scheme
for the shape-from-shading model with ”black shad-
ows”. In Numerical Mathematics and Advanced Ap-
plications. Springer-Verlag.
Falcone, M. and Tozza, S. (2014). Analysis and approxi-
mation of some Shape–from-Shading models for non-
lambertian surfaces. Forthcoming.
Horn, B. and Brooks, M. (1986). The variational approach
to shape from shading. Computer Vision, Graphics,
and Image Processing, 33(2):174–208.
Horn, B. and Brooks, M. (1989). Shape from Shading. The
MIT Press.
Ju, Y. C., Tozza, S., Breuß, M., Bruhn, A., and Kleefeld,
A. (2013). Generalised Perspective Shape from Shad-
ing with Oren-Nayar Reflectance. In Proceedings of
the 24th British Machine Vision Conference (BMVC
2013), Bristol, UK. BMVA, BMVA Press.
Lions, P. L., Rouy, E., and Tourin, A. (1993). Shape-from-
shading, viscosity solutions and edges. Numerische
Mathematik, 64(3):323–353.
Mecca, R. and Falcone, M. (2013). Uniqueness and approx-
imation of a photometric shape-from-shading model.
SIAM J., 6(1):616–659.
Onn, R. and Bruckstein, A. M. (1990). Integrability dis-
ambiguates surface recovery in two-image photomet-
ric stereo. International Journal of Computer Vision,
5(1):105–113.
Oren, M. and Nayar, S. (1994). Generalization of Lambert’s
reflectance model. In Proc. International Conference
and Exhibition on Computer Graphics and Interactive
Techniques (SIGGRAPH), pages 239–246.
Oren, M. and Nayar, S. (1995). Generalization of the Lam-
bertian model and implications for machine vision. In-
ternational Journal of Computer Vision, 14(3):227–
251.
Phong, B. (1975). Illumination for computer generated pic-
tures. Communications of the ACM, 18(6):311–317.
Prados, E., Camilli, F., and Faugeras, O. (2006). A viscosity
solution method for shape-from-shading without im-
age boundary data. ESAIM: Mathematical Modelling
and Numerical Analysis, 40(2):393–412.
Zhang, R., Tsai, P.-S., Cryer, J., and Shah, M. (1999). Shape
from shading: a survey. IEEE Transactions on Pattern
Analysis and Machine Intelligence, 21(8):690–706.
VISAPP2014-InternationalConferenceonComputerVisionTheoryandApplications
716