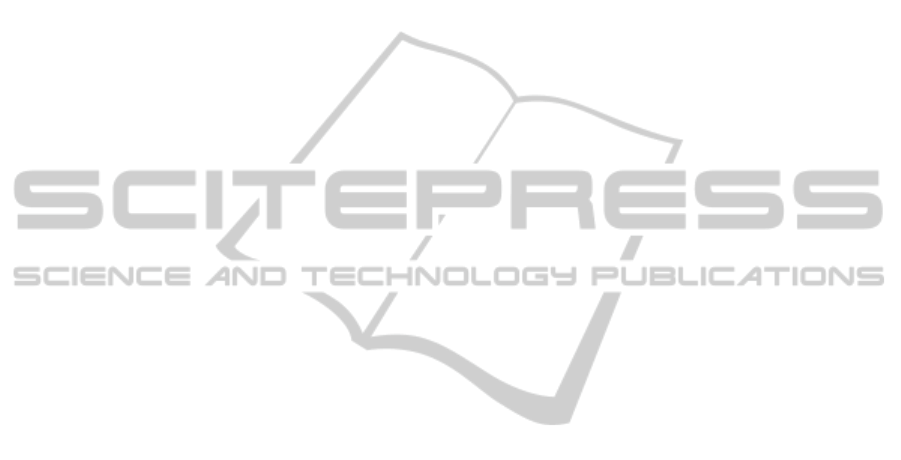
still missing is the capability to produce and exploit
real-time knowledge at a fine grain - in geographical
terms at the neighbourhoodscale; from a people point
of view, at the single dweller level. This is partially
justifiable when considering that such economic data
are usually not openly accessible, even if its digital
trace could be recorded easily. A straightforward ex-
ample could be gathering credit card transactions, for
instance both at shops and on e-commerce sites: as
they belong to either banks or other private financial
entities, the likelihood to access to such gold mine
of information is inversely proportional to the inter-
estingness of its content. On the other hand, even in
presence of open data, urban policies suffers from the
lack of data-driven modelling and practice, thus limit-
ing its effectiveness and comprehension of urban dy-
namics.
Nevertheless, very recent works show that, by us-
ing such non-traditional sources of data, it is possible
to catch fascinating and not-trivial facets of the urban
landscape. For instance, in (Krumme et al., 2013),
the authors could access electronic records of indi-
vidual economic transactions from both North Amer-
ican and European financial institutions to predict pat-
terns of customers’ habits in their shopping activi-
ties in the course of time. By using similar data, an
interactive visualization manages to inspect the eco-
nomic impact of the http://mwcimpact.com/Mobile
World Congress 2012 on the city of Barcelona. This
objective was achieved by visually comparing his-
torical data from the week before and during the
MWC 2012 so that differences in the purchase dy-
namics of the city along that week could emerge.
On the other hand, another visual project, namely
http://senseable.mit.edu/bbva/Spring Spree, was con-
ceived to examine spatio-temporal transactions cate-
gorized by the type of sale (e.g. fashion, restaurants)
during the week of Easter 2011 in order to detect dis-
tinctive patterns emerging across Spain.
1.2 The Role of Visualization
The last aforementioned examples introduce a couple
of important elements to reflect on. First of all, the
Big Data opportunities could turn into the Big Data
nightmare if policies to handle them are not imple-
mented conveniently. Indeed, current approachesusu-
ally do not scale up to face such volume and variety
of information (at a reasonable velocity), thus deter-
mining underused and wasted opportunities.
Then, another awkward point coming up when
dealing with economic studies is its (perceived?) in-
trinsic abstraction in the formulation of problems and
methodologies to work them out. Indeed, models and
formulas usually used in this field are lacking in per-
ceptible concreteness and immediacy. In particular,
this means that their outcomes are neither easily com-
municable nor understandable outside the (few) mem-
bers of the economic ’clique’. Moreover, while talk-
ing about urban economic facts, the risk is somehow
to miss the context in which the economic analysis
is performed and therefore, to reveal the impact on
the real urban environment. We are plenty aware that
classical charts (e.g. bar, pie and scatter-plot charts)
have been extensively used in this field, especially to
portray temporal trends of indicators and indexes. But
they usually do not associate that evidence to the geo-
graphical context they refer to. For instance, in (Mul-
brandon, 2013), a whole dissection of American in-
comes is presented through both classical and more
sophisticated visualizations. Despite that, there is a
lack of visual feedback at the geographical level (but
for some choropleth maps at US scale).
In this context, a smart approach to solve both
problems could rely on information visualization and
visual analysis disciplines. Indeed, they can provide a
strong support to economists and stakeholders in gen-
eral to highlight efficiently the development of urban
economies. The strength of such approaches is to ex-
ploit perceptual mechanisms of the human sight to en-
force cognitive process of learning, as well as provide
powerfultools in dynamically revealingpatterns, rela-
tionships, clusters, unknownfacts and outliers (Shnei-
derman, 1996). In recent years, the same paradigm
has been extensively and successfully applied to re-
veal cities facts and dynamics under different points
of view. So, for instance, different kinds of mobil-
ity (e.g. people and transport systems (Girardin et al.,
2008; Roth et al., 2011)) have been studied accord-
ing to a number of digital traces they leave (e.g. cell
phones, social networks activities and photographies
(Reades et al., 2009; Jankowski et al., 2010)). In this
sense, visual economies are the next facet of the urban
environment gemstone to look at.
1.3 Our Objectives
Within this context, the main contribution of this work
could be summarized with the following points: i)
to provide an introduction to both opportunities and
problems when dealing with urban data and planning
processes (mainly discussed in Sections 1.1 and 1.2);
ii) to suggest engagingabstract, economic studies also
from the perspective of the geography of the cities, in
order to visually relate economic evidences and facts
to their urban context (we will talk about it in Sections
2 and 3); iii) to show some practical examples about
our work on visualizing urban economies applied to
APerceptiveInsightintoCitiesPatternsbyVisualizingUrbanEconomies
223