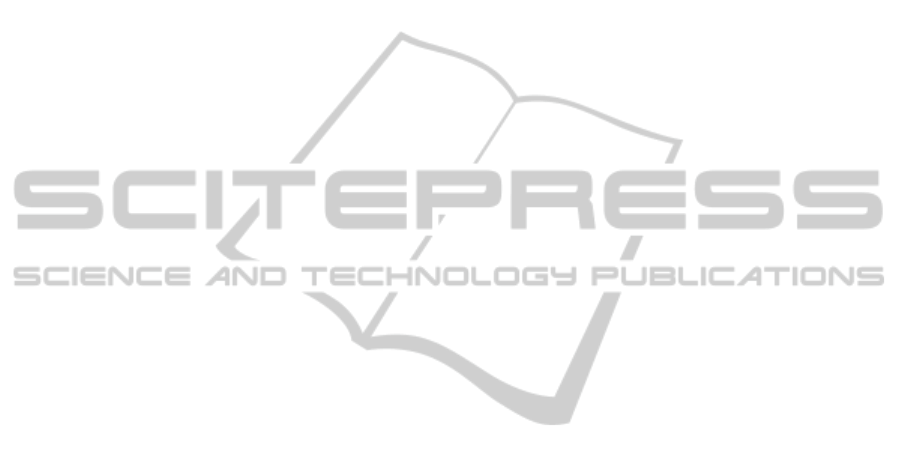
highly sarcastic, with many puns and popular culture
references.
In Table 2 are reported the most frequently ex-
tracted and inferred KPs. All the documents in the set
were associated with the Inferred KP “Music Genre”
and the 97% of them with “Record Label”, which
clearly associates the texts with the music domain.
Evaluation and development are still ongoing and new
knowledge sources, such as domain-specific wikis
and Urban Dictionary, are being considered.
7 CONCLUSIONS AND FUTURE
WORK
In this paper we proposed a truly domain independent
approach to both KP extraction and inference, able
to generate significant semantic metadata with two
different layers of abstraction (phrase extraction and
phrase inference) for any given text without need for
training. The KP extraction part of the system pro-
vides a very fine level of detail, producing KPs that
may not be found in a controlled dictionary (such as
Wikipedia), but characterize the text. Such KPs are
extremely valuable for the purpose of summarization
and provide great accuracy when used as search keys.
However, they are not widely shared, meaning, from
an information retrieval point of view, a very poor re-
call. On the other hand, the KP inference part gener-
ates only KPs taken from a controlled dictionary (the
union of the considered EKSs) that are more likely to
be general, widely known and used, and, therefore,
shared among a significant number of texts.
As shown in the previous section, our approach
can annotate a set of documents with meaningful KPs,
however, a few unrelated KPs may be inferred, mostly
due to ambiguities of the text and to the general-
ist nature of the exploited online external knowledge
sources. This unrelated terms, fortunately, tend to ap-
pear in a limited number of cases and to be clearly
unrelated not only to the majority of the generated
KPs, but also to each other. In fact, our next step in
this research will be precisely to identify such false
positives by means of an estimate of the Semantic Re-
latedness (Strube and Ponzetto, 2006), (Ferrara and
Tasso, 2012) between terms in order to identify, for
each generated KP, a list of related concepts and de-
tect concept clusters in the document.
The proposed KP generation technique can be ap-
plied both in the Information Retrieval domain and in
the Adaptive Personalization one. The previous ver-
sion of the DIKPE system has already been integrated
with good results in RES (De Nart et al., 2013), a per-
sonalized content-based recommender system for sci-
entific papers that suggests papers accordingly to their
similarity with one or more documents marked as in-
teresting by the user, and in the PIRATES framework
(Pudota et al., 2010) for tag recommendation and au-
tomatic document annotation. We expect this ex-
tended version of the system to provide an even more
accurate and complete KP generation and, therefore,
to improve the performance of these existing systems,
in this way supporting the creation of new Semantic
Web Intelligence tools.
REFERENCES
Barker, K. and Cornacchia, N. (2000). Using noun phrase
heads to extract document keyphrases. In Advances in
Artificial Intelligence, pages 40–52. Springer.
Bracewell, D. B., Ren, F., and Kuriowa, S. (2005). Mul-
tilingual single document keyword extraction for in-
formation retrieval. In Natural Language Process-
ing and Knowledge Engineering, 2005. IEEE NLP-
KE’05. Proceedings of 2005 IEEE International Con-
ference on, pages 517–522. IEEE.
Danilevsky, M., Wang, C., Desai, N., Guo, J., and Han, J.
(2013). Kert: Automatic extraction and ranking of
topical keyphrases from content-representative docu-
ment titles. arXiv preprint arXiv:1306.0271.
DAvanzo, E., Magnini, B., and Vallin, A. (2004).
Keyphrase extraction for summarization purposes:
The lake system at duc-2004. In Proceedings of the
2004 document understanding conference.
De Nart, D., Ferrara, F., and Tasso, C. (2013). Personalized
access to scientific publications: from recommenda-
tion to explanation. In User Modeling, Adaptation,
and Personalization, pages 296–301. Springer.
Dumais, S., Platt, J., Heckerman, D., and Sahami, M.
(1998). Inductive learning algorithms and represen-
tations for text categorization. In Proceedings of the
seventh international conference on Information and
knowledge management, pages 148–155. ACM.
Ferrara, F. and Tasso, C. (2012). Integrating semantic relat-
edness in a collaborative filtering system. In Mensch
& Computer Workshopband, pages 75–82.
Ferrara, F. and Tasso, C. (2013). Extracting keyphrases
from web pages. In Digital Libraries and Archives,
pages 93–104. Springer.
Litvak, M. and Last, M. (2008). Graph-based keyword ex-
traction for single-document summarization. In Pro-
ceedings of the workshop on multi-source multilingual
information extraction and summarization, pages 17–
24. Association for Computational Linguistics.
Marujo, L., Gershman, A., Carbonell, J., Frederking, R.,
and Neto, J. P. (2013). Supervised topical key
phrase extraction of news stories using crowdsourc-
ing, light filtering and co-reference normalization.
arXiv preprint arXiv:1306.4886.
Medelyan, O. and Witten, I. H. (2006). Thesaurus based au-
tomatic keyphrase indexing. In Proceedings of the 6th
ADomainIndependentDoubleLayeredApproachtoKeyphraseGeneration
311