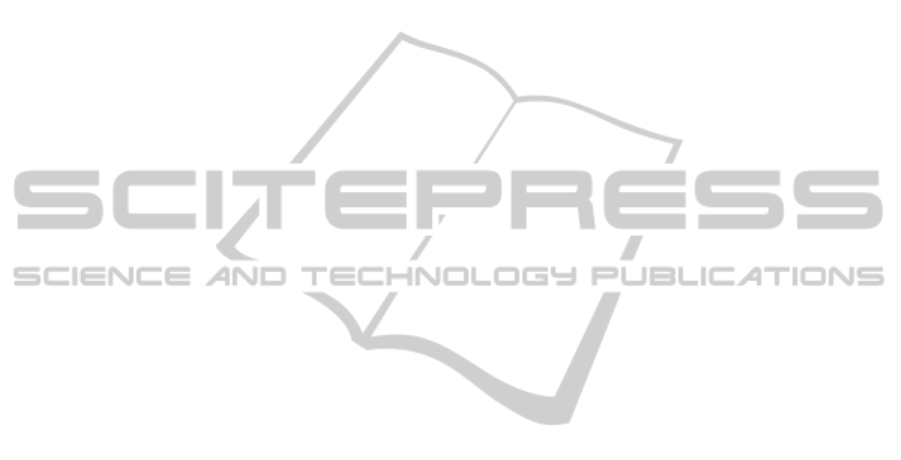
questionnaire and comparing with other reference
methods of risk tolerance estimation allows
achieving preliminary solutions based on stochastic
portfolio optimization for each risk tolerance.
However, a multi-criteria final assessment should be
done, using the Monte Carlo simulation results, in
order to ascertain how decision-makers valuate the
underneath multiple consequences from each
hedging option. This multi-criteria final risk
tolerance evaluation can in fact help the company in
the always difficult decision “to hedge or not to
hedge” and, if yes, which amount to hedge.
It is important to note that these results were
obtained with data and preference judgements
concerning a specific moment in time. Few months
before or later, with different crude and refined
products prices, would lead to different decisions
under this approach. On the other side, each year,
the company has different goals, the market value
can grow or shrink along with the earnings and gross
margins. Further research should be done to evaluate
the results of the model in different price conditions
and involving other decision makers, preferably also
including board directors.
REFERENCES
Artzner, P., Delbaen, F., Eber, J.-M. & Heath, D. 1999.
Coherent measures of risk. Mathematical Finance, 9,
203–228.
Asche, F., Al, E., Gjølberg, O. & Völker, T. 2003. Price
relationships in the petroleum market: An analysis of
crude oil and refined product prices. Energy
Economics, 25, 289-301.
Bana e Costa, C. A., De Corte, J. M. & Vansnick, J. C.
2012. MACBETH. International Journal of
Information Technology & Decision Making, 11, 359-
387.
Bana e Costa, C. A. & Vansnick, J. C. 1999. The
MACBETH approach: Basic ideas, software, and an
application. In: Meskens, N. & Roubens M. R. (eds.)
Advances in Decision Analysis. Kluwer Academic
Publishers, Dordrecht, pp. 131-157
Belton, V. & Stewart, T. J. 2002. Multiple Criteria
Decision Analysis: An Integrated Approach, Kluwer
Academic Publishers, Boston, MA.
Bernoulli, D. 1738. Exposition of a new theory on the
measurement of risk. Econometrica, 22, 23-39.
Bollerslev, T. 1986. Generalized autoregressive
conditional heteroskedasticity. Journal of Economics,
31, 307-327.
Chang, C.-L., Mcaleer, M. & Tansuchat, R. 2011. Crude
oil hedging strategies using dynamic multivariate
GARCH. Energy Economics, 33, 912-923.
Chollete, L. 2008. Economic implications of copulas and
extremes. Penger og Kreditt - Norges Bank, 2, 56-58.
Clemen, R. T. 1996. Making Hard Decisions: An
Introduction to Decision Analysis. Duxbury Press,
Belmont, CA, 2nd edition.
Delquie, P. 2008. Interpretation of the risk tolerance
coefficient in terms of maximum acceptable loss.
Decision Analysis, 5, 5-9.
Energy Information Administration 2002. Derivatives and
risk management in the petroleum, natural gas, and
electricity industries. U.S. Department of Energy.
Fermanian, J.-D. 2005. Goodness-of-fit tests for copulas.
Journal of Multivariate Analysis, 95, 119-152.
Haushalter, D. 2000. Finance policy, basis risk and
corporate hedging: Evidence from oil and gas
producers. The Journal of Finance, 55, 107-152.
Howard, R. A. 1988. Decision analysis: Practice and
promise. Management Science, 34, 679-695.
Huang, J.-J., Lee, K.-J., Liang, H. & Lin, W.-F. 2009.
Estimating value at risk of portfolio by conditional
copula-GARCH method. Insurance: Mathematics and
Economics, 45, 315-324.
J.P.Morgan 1992. RiskMetricsTM—Technical Document.
In: J.P.Morgan/Reuters (ed.) Fourth Edition, 1996
Ji, Q. & Fan, Y. 2011. A dynamic hedging approach for
refineries in multiproduct oil markets. Energy, 36,
881-887.
Jin, Y. & Jorion, P. 2006. Firm value and hedging:
Evidence from U.S. oil and gas producers. The
Journal of Finance, 61, 893-919.
Jobst, N. J., Mitra, G. & Zenios, S. A. 2006. Integrating
market and credit risk: A simulation and optimisation
perspective. Journal of Banking & Finance, 30, 717-
742.
Kaplan, R. S. & Mikes, A. 2012. Managing risks: A new
framework. Harvard Business Review, 90, 48-60.
Kirkwood, C. W. 2004. Approximating risk aversion in
decision analysis applications. Decision Analysis, 1,
51-67.
Kretzschmar, G. L., Kirchner, A. & Reusch, H. 2008. Risk
and return in oilfield asset holdings. Energy
Economics, 30, 3141-3155.
Levy, H. & Markowitz, H. M. 1979. Approximating
expected utility by a function of mean and variance.
American Economic Review, 69, 308-17.
Lu, X. F., Lai, K. K. & Liang, L. 2011. Portfolio value-at-
risk estimation in energy futures markets with time-
varying copula-GARCH model. Annals of Operations
Research, doi: 10.1007/s10479-011-0900-9
Modelrisk 2012. Vose Software. http://
www.vosesoftware.com/
Nomikos, N. & Andriosopoulos, K. 2012. Modelling
energy spot prices: Empirical evidence from NYMEX.
Energy Economics, 34, 1153–1169.
Optquest 2012. OptTek Systems. http:// www.opttek.com/
OptQuest.
Platts 2012. Market Data – Oil, www.platts.com
Pratt, J. W. 1964. Risk aversion in the small and in the
large. Econometrica, 32, 122-136.
Quintino, A., Lourenço, J. C. & Catalão-Lopes, M. 2013.
An integrated risk management model for an oil and
gas company. Proceedings of EBA 2013, 16-19 July,
ManagingPriceRiskforanOilandGasCompany
137