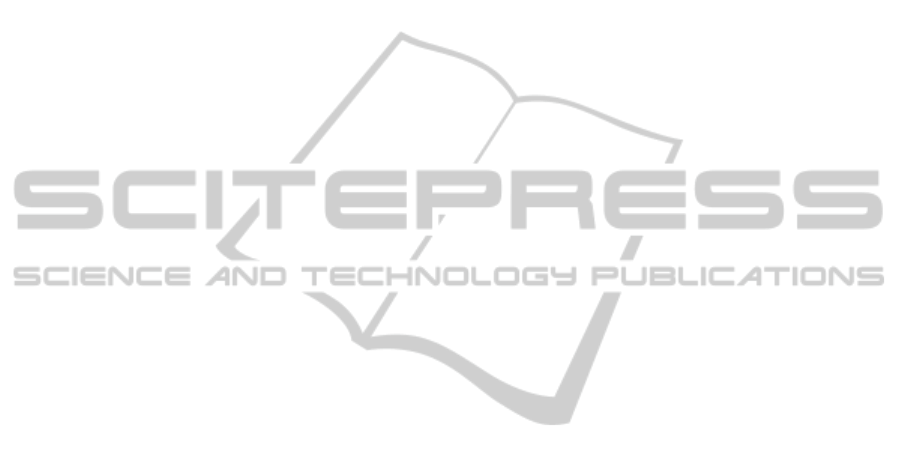
placement, and because of long nights, as is the case
at latitudes of northern continental climates, while,
(b), during winter the indoor to outdoor temperature
difference can reach as much as 60 degrees Celsius
creating unparalleled opportunities for thermoelectric
harvesting. More specifically, we take advantage of
the difference between indoor and outdoor tempera-
ture in buildings in northern climates. To this end, we
use data collected from an actual inhabited apartment
complex in Fort McMurray, Alberta, Canada. The
particular apartment complex has been constructed
using modular construction techniques.
Placing thermoelectric harvesters inside walls
serves the application of powering co-located sensors
that monitor the wall and building behaviour. In cli-
mates like the one considered in our study, extreme
weather conditions can cause events important to the
integrity of a building, e.g., breakage of water pipes,
more frequently occurring than in moderate climates.
The ability to embed sensors in inaccessible locations
that autonomously operate for several decades (i.e., as
long as the building lasts), monitoring for such events,
can currently only be supported using energy harvest-
ing.
In terms of the methodology followed, we use the
difference between indoor air and outdoor air temper-
ature as the basis for the energy harvesting potential
we report in this paper. Nevertheless, the energy har-
vesting specific to an apartment (and, more specifi-
cally, to a location on an exterior wall of an apart-
ment) depends on a number of factors whose com-
bined effect can be captured by the heat flow rate
through specific locations of the exterior walls. We
therefore study whether the difference between indoor
and outdoor temperature at each apartment is a good
proxy for the actual heat flow via the exterior walls.
The heat flow is the real rate of energy transfer via the
wall unit, i.e., the ground truth. Our study indicates
among other things that, even though the difference
between indoor and outdoor air temperature is a good
(scaled) proxy for the average heat flow, there exists
a high degree of variability of heat flow across apart-
ments. Furthermore, the variability is smaller during
certain times of the year. We comment on how these
observations should guide the design of suitable net-
work protocols.
The remaining of the paper is structured as fol-
lows. Section 2 briefly reviews some related work on
the subject at hand, Section 3 has the description of
the data set, and elements of the methodology we fol-
lowed in interpreting them. Section 4 outlines the sys-
tem model that we employ. Section 5 presents numer-
ical performance results. We conclude with Section 6
summarizing our findings.
2 RELATED WORK
Energy harvesting for wireless networks and low
power wireless sensor node architectures are areas of
intense research activity. The use of energy harvesting
for powering WSN nodes, with example applications,
such as smart buildings and predictive maintenance of
structures has been explored in the past (Vullers et al.,
2010).
In this paper we narrow our focus to thermoelec-
tric generators and specifically exploit the energy lost
through walls in cold climates. We use a device model
loosely based on (Mateu et al., 2006), in which energy
harvesting from the human body was used, which is
an idea explored by other researchers as well, (Ra-
madass and Chandrakasan, 2011; Wang et al., 2009).
The devices proposed in previous works assume oper-
ation based on the difference of temperature between
the human body and its environment, thus indirectly
exploiting the temperature homeostasis of the human
body.
In this paper, we are not concerned with the task of
increasing the energy harvesters efficiency, a topic of
intense activity anyway, e.g., (Hudak and Amatucci,
2008; Luber et al., 2013). We use off-the-shelf com-
ponents and we do not even use a sensor platform op-
timized for energy efficiency. In other words, the re-
sults we present here are very close to representing a
“worst case” scenario with respect to the devices em-
ployed.
In the field of energy harvesting for WSNs, a no-
table work is that of Gorlatova et al. (Gorlatova et al.,
2009; Gorlatova et al., 2011) which focuses on how
to use photovoltaic harvesting under diverse use sce-
narios, by proposing suitable optimization models.
Another distinct property of their work is the study
of photovoltaic harvesting in environments under the
control of the users (depending on indoor illumina-
tion, or in the pocket of users), i.e., with idiosyncratic
and sometimes unpredictable behaviour. They also
consider elementary WSN applications, such as ID
beacon transmission.
3 THE DATA SET
We use data collected over a period of a year, from 11
different apartments within a single apartment com-
plex in Fort McMurray, Alberta, Canada. The data
collected is comprehensive, including such aspects as
water flow and temperature for the water used by radi-
ators for heating, water flow and temperature for res-
idential water, CO2 concentration, etc. For the pur-
poses of this study we consider only the heat flow
SMARTGREENS2014-3rdInternationalConferenceonSmartGridsandGreenITSystems
214