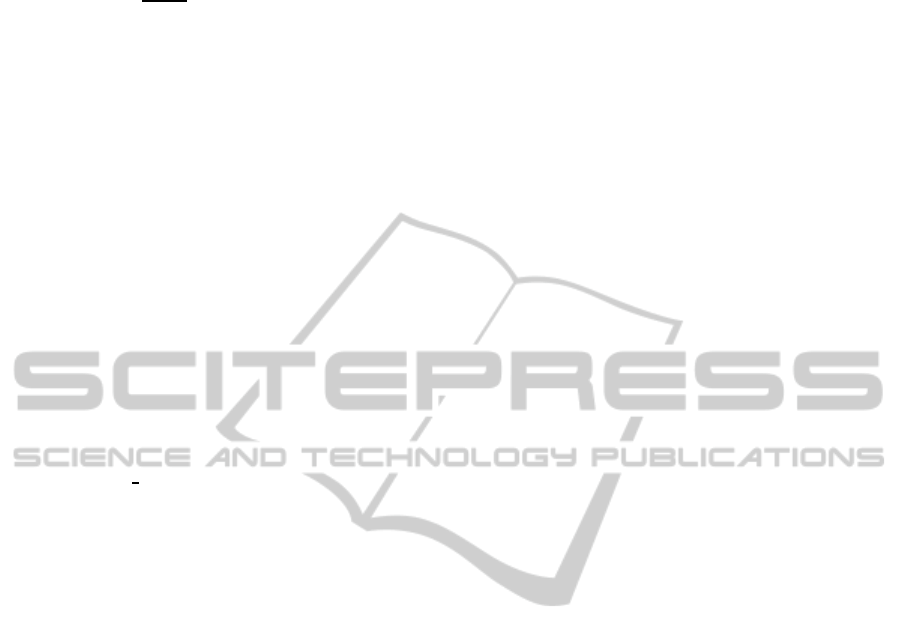
tional performancewith dramatically better character-
istic of the former in terms of hardware implementa-
tion, regardless the
MAD is implemented as a combi-
natorial trellis as suggested by some authors or in a
more efficient folded version. The paper also stresses
the benefit of using hardware-software co-simulations
tools such as Xilinx System Generator for rapid proto-
typing and verification on FPGA, which represents a
value added for the research in rapidly evolving fields
such as neural engineering, overcoming the limits of
the traditional HDL coding.
ACKNOWLEDGEMENTS
The research leading to these results has received
funding by the Region of Sardinia in the ELoRA
project (Fundamental Research Programme, L.R.
7/2007, grant agreement CRP-60544), by the Euro-
pean Commission in the NEBIAS project (FP7, FET
Proactive, grant agreement 611687), by Italian Gov-
ernment in the HANDBOT project (PRIN 2010/11,
prot. 2010YF2RY
003) and by the Italian Ministry of
Health in the NEMESIS project (Young Researchers,
grant agreement 064/GR-2009-1591615).
REFERENCES
Bahoura, M. and Ezzaidi, H. (2012). FPGA-
implementation of discrete wavelet transform with
application to signal denoising. Circuits, Systems,
and Signal Processing, 31(3):987–1015.
Baig, M. M., Gholamhosseini, H., and Connolly, M. J.
(2013). A comprehensive survey of wearable and
wireless ECG monitoring systems for older adults.
Medical & Biological Engineering & Computing,
51(5):485–495.
Casson, A., Yates, D., Smith, S., Duncan, J., and Rodriguez-
Villegas, E. (2010). Wearable electroencephalogra-
phy. Engineering in Medicine and Biology Magazine,
IEEE, 29(3):44–56.
Citi, L., Carpaneto, J., Yoshida, K., Hoffmann, K.-P., Koch,
K. P., Dario, P., and Micera, S. (2008). On the use of
wavelet denoising and spike sorting techniques to pro-
cess electroneurographic signals recorded using intra-
neural electrodes. Journal of Neuroscience Methods,
172:294–302.
Cohen, A. and Kovacevic, J. (1996). Wavelets: the
mathematical background. Proceedings of the IEEE,
84(4):514–522.
Diedrich, A., Charoensuk, W., Brychta, R., Ertl, A., and
Shiavi, R. (2003). Analysis of raw microneurographic
recordings based on wavelet de-noising technique
and classification algorithm: wavelet analysis in mi-
croneurography. IEEE Trans Biomed Eng, 50(1):41–
50.
Holschneider, M., Kronland-Martinet, R., Morlet, J., and
Tchamitchian, P. (1990). A real-time algorithm for
signal analysis with the help of the wavelet transform.
In Wavelets, pages 286–297. Springer Berlin Heidel-
berg.
Kuzume, K., Niijima, K., and Takano, S. (2004). FPGA-
based lifting wavelet processor for real-time signal de-
tection. Signal Processing, 84(10):1931–1940.
Mahmoud, M. I., Dessouky, M. I. M., Deyab, S., and
Elfouly, F. H. (2008). Signal denoising by wavelet
packet transform on FPGA technology. Special Issue
of Ubiquitous Computing and Communication Jour-
nal Bioinformatics and Image.
Martinez, J., Cumplido, R., and Feregrino, C. (2005). An
FPGA-based parallel sorting architecture for the Bur-
rows Wheeler transform. In International Conference
on Reconfigurable Computing and FPGAs, ReConFig
2005.
Medina, C., Alcaim, A., and Jr., J. A. (2003). Wavelet de-
noising of speech using neural networks for threshold
selection. Electronics Letters, 39(25):1869–1871.
Montani, M., Marchi, L. D., Marcianesi, A., and Speciale,
N. (2003). Comparison of a programmable DSP and
FPGA implementation for a wavelet-based denoising
algorithm. In Proc. IEEE 46th Midwest Symposium
on Circuits and Systems, volume 2, pages 602–605.
Nezan, J., Siret, N., Wipliez, M., Palumbo, F., and Raffo,
L. (2012). Multi-purpose systems: A novel dataflow-
based generation and mapping strategy. In Proc. IEEE
International Symposium on Circuits and Systems (IS-
CAS), pages 3073–3076.
Palumbo, F., Carta, N., Pani, D., Meloni, P., and Raffo, L.
(2012). The multi-dataflow composer tool: genera-
tion of on-the-fly reconfigurable platforms. Journal of
Real-Time Image Processing, pages 1–17.
Pani, D., Usai, F., Citi, L., and Raffo, L. (2011). Impact of
the approximated on-line centering and whitening in
OL-JADE on the quality of the estimated fetal ecg. In
Proc. of the 5th International IEEE/EMBS Conference
on Neural Engineering (NER), pages 44–47.
Quiroga, R. Q., Nadasdy, Z., and Ben-Shaul, Y. (2004). Un-
supervised spike detection and sorting with wavelets
and superparamagnetic clustering. Neural Comput.,
16(8):1661–1687.
Radovan, S., Saˇsa, K., Dejan, K., and Goran, D. (2013). Op-
timization and implementation of the wavelet based
algorithms for embedded biomedical signal process-
ing. Computer Science and Information Systems,
10:502–523.
Zhang, M., Deng, R., Ma, Z., and Zhang, M. (2011). A
FPGA-based low-cost real-time wavelet packet de-
noising system. In Proc. of 2011 Int. Conf. on
Electronics and Optoelectronics (ICEOE), volume 2,
pages V2–350–V2–353.
BIODEVICES2014-InternationalConferenceonBiomedicalElectronicsandDevices
52