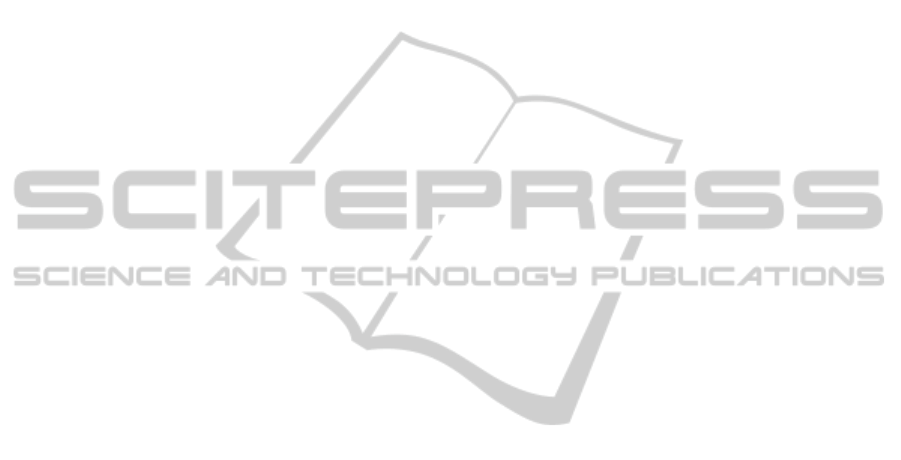
2006 IEEE International Conference on, pages 2381–
2384. IEEE.
De Smedt, F., Van Beeck, K., Tuytelaars, T., and Goedem
´
e,
T. (2013). Pedestrian detection at warp speed: Ex-
ceeding 500 detections per second. In Proceedings
of IEEE Conference on Computer Vision and Pattern
Recognition.
Doll
´
ar, P., Belongie, S., and Perona, P. (2010). The fastest
pedestrian detector in the west. In BMVC, volume 2-3,
page 7.
Doll
´
ar, P., Tu, Z., Perona, P., and Belongie, S. (2009). Inte-
gral channel features. In BMVC, volume 2-4, page 5.
Felzenszwalb, P., Girshick, R., and McAllester, D. (2010).
Cascade object detection with deformable part mod-
els. In CVPR, pages 2241–2248.
Freund, Y., Schapire, R., and Abe, N. (1999). A short in-
troduction to boosting. Journal-Japanese Society For
Artificial Intelligence, 14(771-780):1612.
Gall, J. and Lempitsky, V. (2013). Class-specific hough
forests for object detection. In Decision Forests for
Computer Vision and Medical Image Analysis, pages
143–157. Springer.
Hammami, M., Jarraya, S. K., and Ben-Abdallah, H.
(2013). On line background modeling for moving
object segmentation in dynamic scenes. Multimedia
Tools and Applications, pages 1–28.
Hsieh, J., Liao, H., Fan, K., Ko, M., and Hung, Y. (1997).
Image registration using a new edge-based approach.
CVIU, pages 112–130.
Huang, C., Ai, H., Li, Y., and Lao, S. (2005). Vector boost-
ing for rotation invariant multi-view face detection. In
Computer Vision, 2005. ICCV 2005. Tenth IEEE In-
ternational Conference on, volume 1, pages 446–453.
IEEE.
Kapoor, A., Grauman, K., Urtasun, R., and Darrell, T.
(2007). Active learning with gaussian processes for
object categorization. In Computer Vision, 2007.
ICCV 2007. IEEE 11th International Conference on,
pages 1–8. IEEE.
Kurz, G., Gilitschenski, I., Julier, S., and Hanebeck,
U. D. (2013). Recursive estimation of orientation
based on the bingham distribution. arXiv preprint
arXiv:1304.8019.
Leiva-Valenzuela, G. A. and Aguilera, J. M. (2013). Auto-
matic detection of orientation and diseases in blueber-
ries using image analysis to improve their postharvest
storage quality. Food Control.
Lewis, J. (1995). Fast normalized cross-correlation. In Vi-
sion interface, volume 10, pages 120–123.
Li, X. and Guo, Y. (2013). Adaptive active learning for im-
age classification. In IEEE Conf. on Computer Vision
and Pattern Recognition (CVPR).
Mathias, M., Timofte, R., Benenson, R., and Gool, L.
(2013). Traffic sign recognition-how far are we from
the solution? In Proceedings of IEEE International
Joint Conference on Neural Networks.
Mindru, F., Tuytelaars, T., Gool, L., and Moons, T. (2004).
Moment invariants for recognition under changing
viewpoint and illumination. CVIU, 94:3–27.
Mittal, A., Zisserman, A., and Torr, P. (2011). Hand detec-
tion using multiple proposals. BMVC 2011.
Puttemans, S. and Goedem
´
e, T. (2013). How to exploit
scene constraints to improve object categorization al-
gorithms for industrial applications? In Proceedings
of the international conference on computer vision
theory and applications (VISAPP 2013), volume 1,
pages 827–830.
Riaz, F., Hassan, A., Rehman, S., and Qamar, U.
(2013). Texture classification using rotation-and
scale-invariant gabor texture features. IEEE Signal
Processing Letters.
Shackelford, A. K. and Davis, C. H. (2003). A combined
fuzzy pixel-based and object-based approach for clas-
sification of high-resolution multispectral data over
urban areas. Geoscience and Remote Sensing, IEEE
Transactions on, 41(10):2354–2363.
Van Beeck, K., Goedem
´
e, T., and Tuytelaars, T. (2012). A
warping window approach to real-time vision-based
pedestrian detection in a truck’s blind spot zone. In
ICINCO, volume 2, pages 561–568.
Villamizar, M., Moreno-Noguer, F., Andrade-Cetto, J., and
Sanfeliu, A. (2010). Efficient rotation invariant object
detection using boosted random ferns. In Computer
Vision and Pattern Recognition (CVPR), 2010 IEEE
Conference on, pages 1038–1045. IEEE.
Viola, P. and Jones, M. (2001). Rapid object detection using
a boosted cascade of simple features. In CVPR, pages
I–511.
Yeh, C.-H., Lin, C.-Y., Muchtar, K., and Kang, L.-W.
(2013). Real-time background modeling based on a
multi-level texture description. Information Sciences.
Yu, Q., Gong, P., Clinton, N., Biging, G., Kelly, M., and
Schirokauer, D. (2006). Object-based detailed vegeta-
tion classification with airborne high spatial resolution
remote sensing imagery. Photogrammetric Engineer-
ing and Remote Sensing, 72(7):799.
VISIGRAPP2014-DoctoralConsortium
34