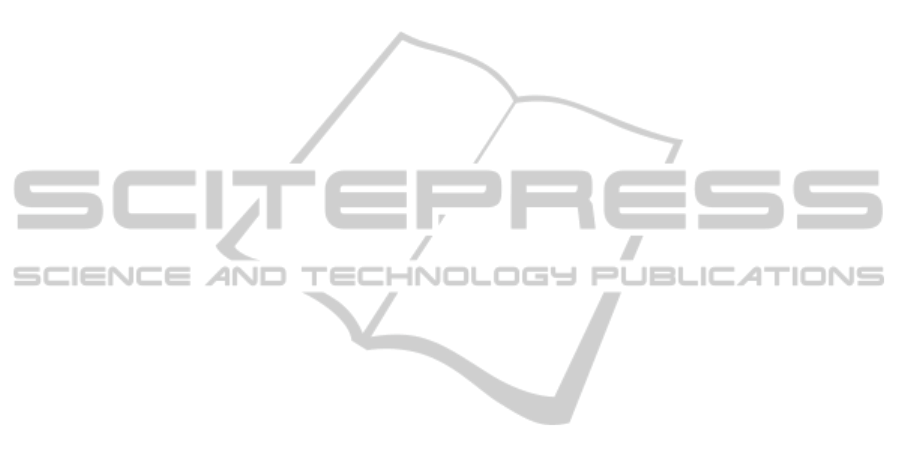
Albatineh, A. N., 2010. Means and variances for a family
of similarity indices used in cluster analysis. Journal
of Statistical Planning and Inference, 140, 2828-2838.
Albatineh, A. N. & Niewiadmska-Bugaj, M., 2011.
Correcting Jaccard and other similarity indices for
chance agreement in cluster analysis. Advances in
Data Analysis and Classification, 5, 179-200.
Amorim, M. J. &Cardoso, M. G. M. S., 2010. Limiares De
Concordância Entre Duas Partições. Livro de Resumos
do XVIII Congresso Anual da Sociedade Portuguesa
de Estatística, 47-49.
Amorim, M. J. P. C. & Cardoso, M. G. M. S., 2012.
Clustering cross-validation and mutual information
indices. In: Ana Colubi, K. F., Gil Gonzalez-
Rodriguesand Erricos John Kontoghiorghes, ed. 20th
International Con-ference on Computational Statistics
(COMPSTAT 2012), 2012 Limassol, Cyprus. The
International Statistical Institute/International
Association for Statistical Computing, 39-52.
Chumwatana, T., Wong, K. W. & Xie, H., 2010. A SOM-
Based Document Clustering Using Frequent Max
Substrings for Non-Segmented Texts. J. Intelligent
Learning Systems & Applications,, 2, 117-125.
Czekanowski, J., 1932. "Coefficient of racial likeness" and
"durchschnittliche Differenz". Anthropologischer
Anzeiger, 14, 227-249.
Dempster, A. P., Laird, N. M. & Rubin, D. B., 1977.
Maximum likelihood from incomplete data via the EM
algorithm.Journal of the Royal Statistical Society.
Series B (Methodological), 1-38.
Everit, B., Landau, S. & Leese, M. 2001. Cluster Analysis,
London, Arnold.
Fowlkes, E. B. &mallows, C. L., 1983. A method for
comparing two hierarchical clusterings.Journal of the
American Statistical Association, 78, 553-569.
Goodman, L. A. & Kruskal, W. H., 1954. Measures of
Association for Cross Classifications. Journal of the
American Statistical Associations, 49.
Gower, J. C. & Legendre, P., 1986. Metric and Euclidean
Properties of Dissimilarity Coefficients. Journal of
Classification, 3.
Halton, J. H., 1969. A rigorous derivation of the exact
contingency formula. In:Proceedings of the
Cambridge Philosophical Society. Cambridge Univ
Press, 527-530.
Hennig, C., 2006. Cluster-wise assessment of cluster
stability. Research report nº 271, Department of
Statistical Science, University College London.
Hubert, L. and Arabie, P. 1985. Comparing partitions.
Journal of classification,
2, 193-218.
Jaccard, 1908. Nouvelles Recerches sur la Distribuition
Florale. Bulletin de la Societé Vaudoise de Sciences
Naturells, 44, 223-370.
Jain, A. K., 2010. Data clustering: 50 years beyond K-
means. Pattern Recognition Letters, 31, 651-666.
Krzanowski, W. J. & Marriott, F. H. C., 1994.
Multivariate analysis, Edward Arnold London.
Lebret, R., S., L., Langrognet, F., Biernacki, C., Celeux,
G. & Govaert, G., 2012. Rmixmod: The r package of
the model-based unsupervised, supervised and semi-
supervised classification mixmod library.http://cran.r-
project.org/web/ packages/Rmixmod/index.html.
Maitra, R. & Melnykov, V., 2010. Simulating data to
study performance of finite mixture modeling and
clustering algorithms. Computational and Graphical
Statistics, 19, 354-376.
Meyeri, A. D. S., Garcia, A. A. F., Souza, A. P. & JR., C.
L. D. S., 2005. Comparison of similarity coefficients
used for cluster analysis with dominant markers in
maize (Zea mays L). Genetics and Molecular Biology,
27, 83-91.
Milligan, G. W. & Cooper, M. C., 1986. A Study of
Comparability of External Criteria for Hierarchical
Cluster Analysis. Multivariate Behavioral Reserch,
21, 441-458.
O’Hagan, A., Murphy, T. B. & Gormley, I. C., 2012.
Computational aspects of fitting mixture models via
the expectation–maximization algorithm. Compu-
tational Statistics and Data Analysis, 56, 3843-3864.
Rand, W. M., 1971. Objective Criteria for the Evaluation
of Clustering Methods. Journal of the American
Statistical Association, 66, 846-850.
RusseL, P. F. & Rao, T. R. 1940. On Habitat and
Association of Species of Anophelinae Larvae in
South-Eastern Madras. J. Malar. Inst. India, 3, 153-
178.
Shamir, O. and tishby, N., 2010. Stability and model
selection in k-means clustering. Mach Learn, 80, 213-
244.
Sokal, R. R. and Sneath, P. H., 1963. Principles of
Numerical Taxonomy, San Francisco CA: Freeman.
ICEIS2014-16thInternationalConferenceonEnterpriseInformationSystems
170