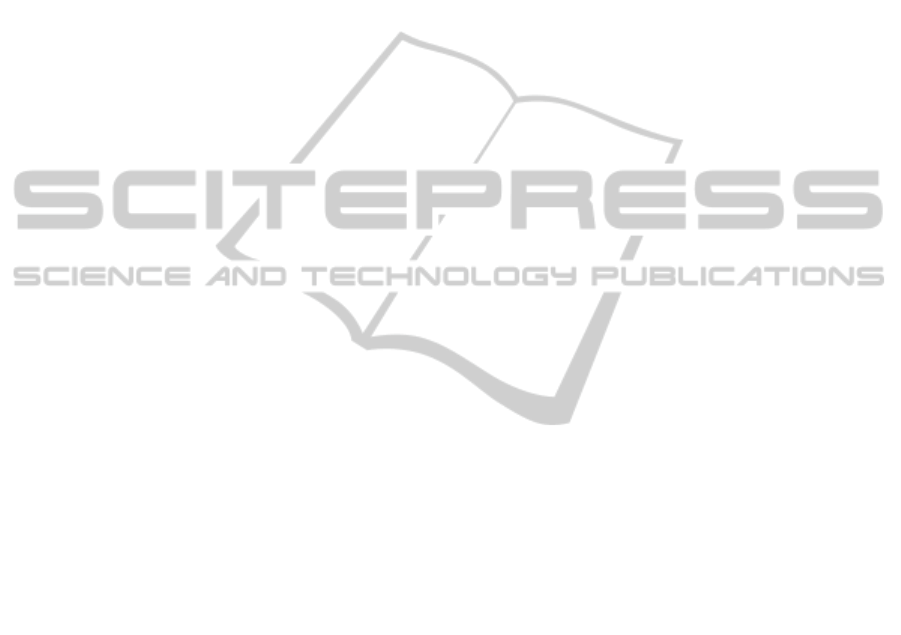
verification rate of the proposed method achieves
95.45% which is superior than that of eigeniris about
81.57% and that of baseline about 61.48%, as show
in Figure 6. Moreover, we discover that the kernel
learning method in the cross-sensor iris matching
problem can not exhibit high recognition
performance.
Figure 7 shows the histogram of HD distribution
for the authentic and impostor comparison, before
(baseline) and after applying the proposed method.
We can see that the authentic score distribution
obviously being moved toward left side, while the
imposter score distribution remains almost the same.
Moreover, the EER of the proposed method achieves
0.8576%, compared to EER=4.7726% in the
baseline experiment. The results show that the two
distributions are moved further away from each
other, demonstrating the effectiveness of the
proposed method.
Figure 8 shows the example iris images
hallucinated by the proposed methods SR
(heterogeneous dictionary learning method using
sparse representation). From these three examples,
given test IOM image whose quality is low, we can
see that hybrid iris dictionary learning method using
sparse representation can synthesize high quality
image that look as if it is captured by the PIER
device.
4 CONCLUSIONS
In this paper, we propose a novel patch-based hybrid
iris dictionary learning method using sparse
representation to approach the issue of cross-sensor
iris matching. The proposed method achieves better
recognition performance for two situations: 1) the
iris images for training and testing are acquired by
different iris image sensors; 2) the training set
images have higher quality while the test images
have lower quality. Furthermore, the experimental
results shows the proposed method successfully
enhance the iris recognition performance in terms of
EER and separability of Hamming distance
distribution, as shown in Figure 6 and 7. Future
work includes using more delicate algorithm (for
example, k-SVD) for dictionary atom update and
collecting more heterogeneous iris images for large-
scale experiment.
ACKNOWLEDGEMENTS
This work was financially supported by the National
Science Council of Taiwan under contract no. NSC
102-2221-E-008 -115.
REFERENCES
K. W. Bowyer, 2008, K. Hollingsworth, and P. J. Flynn,
Image understanding for iris biometrics: A survey,
Computer Vision and Image Understanding, vol.110,
no.2, pp. 281-307.
K. Bowyer, S. Baker, A. Hentz, K. Hollingsworth, T.
Peters, and P. Flynn, 2009, Factors that degrade the
match distribution in iris biometrics, Identity in the
information Society, vol.2, no.3, pp. 327-343.
R. Connaughton, A. Sgroi, K. W. Bowyer, and P. J. Flynn,
2011, A cross-sensor evaluation of three commercial
iris cameras for iris biometrics, IEEE Computer
Society Workshop on Biometrics, pp. 90-97.
Pillai, M. Puertas, and R. Chellappa, 2014, "Cross-sensor
Iris Recognition through Kernel Learning," IEEE
Transactions on Pattern Analysis and Machine
Intelligence, vol.36, no. 1, pp. 73-85.
K. Q. Weinberger, F. Sha, and L. K. Saul, 2004, Learning
a kernel Matrix for nonlinear dimensionality reduction,
International Conference on Machine learning,
pp.839-846.
Y. Li, M. Savvides, and V. Bhagavatula, 2006,
Illumination Tolerant Face Recognition Using a Novel
Face From Sketch Synthesis Approach and Advanced
Correlation Filters, 2006 IEEE International
Conference on Acoustics, Speech and Signal
Processing, 2006. ICASSP 2006 Proceedings, vol.2,
no., pp. II, II, 14-19.
Y. Li and M. Savvides, 2006, Faces from sketches: a
subspace synthesis approach, Defense and Security
Symposium. International Society for Optics and
Photonics.
Securimetrics pier device, securiMetrics Inc., http://
www.securimetrics.com/solutions/pier.html.
J. Matey, O. Naroditsky, K. Hanna, R. Kolczynski, D.
LoIacono, S. Mangru, M. tinker, T.Zappia, and W.
Zhao, 2006, Iris on the move: Acquisition of images
for iris recognition in less constrained environments,
Proceedings of the IEEE, vol. 94, no.11, pp. 1936-
1947.
G. Davis, S. Mallat, and M. Avellaneda, Adaptive Greedy
Approximations, Constructive Approximation, vol. 13,
no. 1, pp.57-98, 1997.
Y. Pati, R. Rezaiifar, and P. Krihnaprassad, Orthogonal
Matching Pursuit: Recursive Function Approximation
with Applications to Wavelet Decomposition,
Conference Record of The Twenty-Seventh Asilomar
Conference on Signals, Systems and Computers, pp.
40-44, 1993.
ICEIS2014-16thInternationalConferenceonEnterpriseInformationSystems
174