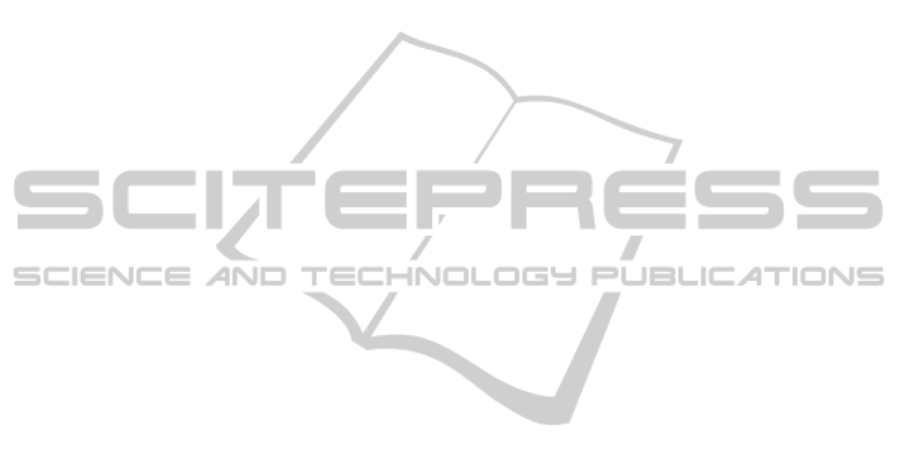
depends only on its parents. A set with all random
variables being instantiated is called an interpretation
of the MEBN theory. Thus, the joint probability that
combines all the local conditional distributions of the
random variables assigns a probability to each inter-
pretation.
From a Bayesian perspective, a MEBN the-
ory (MTheory) is a collection of MEBN fragments
(MFrags). An MFrag is the analogous of a BN node,
since it includes resident nodes, for which a local
conditional distribution is defined. These variables
are conditioned on the input nodes, i.e., their the an-
cestors. Note that every input node in an MFrag is
a resident node in another MFrag, which is called
home MFrag. Thus, each MFrag is a fragment of the
global Bayesian network consisting of all MFrag of
the MTheory. Another component of an MFrag is the
context terms which are used to specify logical con-
straints for the instantiations of the ordinary logical
variables. We must note that, an ordinary variable
can have multiple instantiations for a single MThe-
ory interpretation. Since the random variables have
logic variables (or functions) as arguments, the result-
ing SSBN contains multiple random variables, one for
each argument instantiation. Nevertheless, local dis-
tributions can be designed with the ability to take into
account the volatile number of parents. Note that this
is the core mechanism provided by FOBL for the con-
struction of SSBN.
The ultimate goal of modeling with MEBN is in-
ference, which provides us with the ability to ana-
lyze observations, taken from the real world and draw
conclusions. Inference is performed in SSBN that
result based on evidence. There are two steps in
SSBN construction. In the first step, new evidence
are incorporated in the MTheory by instantiating or-
dinary variables, which enables the construction of
the SSBN that models the specific situation. Other
evidence are observations of a subset of the random
variables. Thus, before inference, these variables are
instantiated to their observations (i.e., they are the ob-
served variables). Then, Bayesian inference provides
the posterior probability distribution of the unknown
random variables we want to estimate. This posterior
distribution can also be used to learn the conditional
distributions the target random variables.
With this position paper, we aim to highlight
MEBN as a knowledge representation and analysis
tool for the ICH domain. Specifically, our goal is to
recognize ICH concepts, such as dance types, singing
styles, etc., in ICH content, based on their formal def-
inition in a knowledge representation schema. This
schema has been developed with consultation from
the domain knowledge experts and it is expected to
boost the analysis performance. The concepts meant
to be detected are manifested in ICH content by per-
formers (singers, dancers, etc.), which content comes
with various modalities, e.g., audio, video, motion.
Thus, we deal with multimodal analysis.
There are two main reasons we propose MEBN
for this task. The first is the uncertainty modeling ca-
pability and the second is the situation specific con-
struction of BN that MEBN provides. For example,
regarding uncertainty, a movement pattern in a tra-
ditional dance is not always deterministically driven,
since people may chose to follow a variant of the stan-
dard dance movement. Another source of uncertainty
arises also from the inaccuracy of the basic concept
detection algorithms, e.g., some steps that comprise
the dance may not be detected. The second reason
is that, usually, it is not a priori known how many
dancers, singers, or even dances and songs are mani-
fested in a particular timespan of ICH content. SSBN
construction using FOBL can be proven very benefi-
cial for dynamic modeling of such situations.
A concise example of MEBN usage for multi-
modal traditional dance modeling is presented in Fig-
ure 1. In this figure, an MTheory is presented consist-
ing of three MFrags. The ”Dance” MFrag contains
one input random variable, DanceType, with its states
being the ”dance type” instances (Tsamiko, Calus,
etc.). The resident random variables of this MFrag
are Steps(d,t1), Steps(d,t2), MusicTempo(d). The first
two denote a sequence of steps and the third the mu-
sic tempo accompanying the dance. Due to the re-
cursive definition of the steps, there can be numer-
ous steps, depending on the number of the time vari-
ables instances. These instances depend on the ev-
idence being found in the ICH content (i.e., the time
instants). Let us suppose a particular variable instanti-
ation in order to demonstrate the mechanism that pro-
duces an SSBN. For simplicity of the demonstration,
we will work only with the Dance MFrag. Suppose
that the following logical entities have been found
as evidence: T 0, T 1, T 2, Dance1. This means that
only one dance is performed. d=Dance, starting at
time T 0 and ending at T 2 (T 0, T 1, T2 is an ordered
sequence of time instants). Regarding random vari-
ables, the observations are three consecutive steps, re-
sulting in the instantiations Step(Dance1,T0)=Right,
Step(Dance1,T1)=Right, Step(Dance1,T2)=Left, and
one tempo MusicTempo(Dance1)=Slow. The partial
SSBN produced by these instantiations is depicted in
Figure 2.
The instantiated random variables in the SSBN
of Figure 2 are the observed variables. The goal is
to estimate the posterior distribution of the Dance-
Type variable given the observations. This is achieved
Multi-entityBayesianNetworksforTreasuringtheIntangibleCulturalHeritage
799