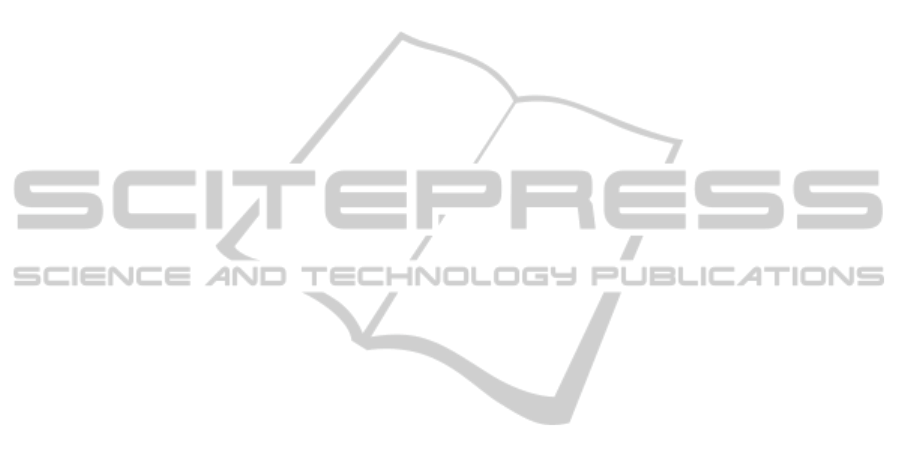
6 CONCLUSIONS
In this study, we have tested the use of typicality de-
grees measure the relevance of physiological signals
to model users’ affective states. We considered typ-
icality as per cognitive and psychology principles of
categorization to discover pertinent psychophysiolog-
ical relations. We showed how this framework is a
powerful characterization tool.
Regarding characterization task, we were able to
extract key psychophysiological characteristics for
modeling real-life affective systems. Our experimen-
tal results revealed that Electrodermal activity (EDA)
measure is very powerful in characterizing all the
users’ states. When considering a player’s affective
states, we found that heart rate is less relevant than
EDA, but is critical to distinguish a state of comfort
from a state of frustration. On the contrary, the char-
acterization power of respiration recordings (RR) was
low. Thus, in relation to affective gaming, our results
show that it is possible to gain information from phys-
iological signals considering the optimal state of sat-
isfaction of a player.
However, still much is to be done before getting
access to the structure of the player’s emotional pro-
cesses. In particular, to consider multi-modal fusion
of measures such as audio-visual and various physio-
logical measures.
REFERENCES
Bailenson, J. N., Pontikakis, E. D., Mauss, I. B., Gross, J. J.,
Jabon, M. E., Hutcherson, C. A., Nass, C., and John,
O. (2008). Real-time classification of evoked emo-
tions using facial feature tracking and physiological
responses. International Journal of Human-Computer
Interaction, 6:303–317.
Boiten, F. A. (1998). The effects of emotional behaviour
on components of the respiratory cycle. Biological
Psychology, 49:29–51.
Boiten, F. A., Frijda, N. H., and Wientjes, C. J. (1994).
Emotions and respiratory patterns: review and critical
analysis. International Journal of Psychophysiology,
17:103–128.
Bouchon-Meunier, B., Rifqi, M., and Bothorel, S. (1996).
Towards general measures of comparison of objects.
Fuzzy Sets and Systems, 84:143–153.
Bradley, M., Greenwald, M. K., and Hamm, A. (1993).
Affective picture processing. In Birbaumer, N. and
Ohman, A., editors, The Structure of Emotion, pages
48–65. Hogrefe and Huber Publishers, Toronto.
Cacioppo, J., Bush, L., and Tassinary, L. (1992). Microex-
pressive facial actions as a function of affective stim-
uli: replication and extension. Personality and Social
Psychology Bulletin, 18:515–526.
Cacioppo, J. T., Tassinary, L. G., and Berntson, G. G.
(2007). Psychophysiological science: interdisci-
plinary approaches to classic questions about the
mind. In Cacioppo, J. T., Tassinary, L. G., and
Berntson, G. G., editors, Handbook of psychphysiol-
ogy, pages 1–18. New York: Cambridge University
Press.
Calvo, R. A. and D’Mello, S. (2010). Affect detection: an
interdisciplinary review of models, methods, and their
applications. IEEE Transactions on Affective Comput-
ing, 1:18 – 37.
Chanel, G., Kierkels, J., Soleymani, M., and Pun, T. (2009).
Short-term emotion assessment in a recall paradigm.
International Journal of Human-Computer Studies,
67:607–627.
Detenber, B., Simons, R., and Bennett, G. (1998). Roll’
em! the effects of picture motion on emotional re-
sponses. Journal of Broadcasting and Electronic Me-
dia., 21:112–126.
Detyniecki, M. (2001). Mathematical aggregation opera-
tors and their application to video querying. PhD the-
sis, Universit
´
e Pierre et Marie Curie, France.
Ekman, P., Levenson, R., and Friesen, W. (1983). Auto-
nomic nervous system activity distinguishes among
emotions. Science, 221:1208–1210.
Fairclough, S. (2009). Fundamentals of physiological com-
puting. Interacting With Computers, 21:133–145.
Haag, A., Goronzy, S., Schaich, P., and Williams, J. (2004).
Emotion recognition using bio-sensors: First stepts to-
wards an automatic system. Lecture Notes in Com-
puter Science, Affective Dialogue Systems, 3068:36–
48.
Kim, J. and Andr
´
e, E. (2008). Emotion recognition based on
physiological changes in music listening. IEEE Trans-
actions on Pattern Analysis And Machine Intelligence,
30(12):2067–2083.
Lang, P. (1995). The emotion probe studies of motivation
and attention. American Psychologist, 50(5):372–385.
Lesot, M.-J., Mouillet, L., and Bouchon-Meunier, B.
(2006). Fuzzy prototypes based on typicality degrees.
In Fuzzy Days04, Springer, Advances on Soft Comput-
ing.
Lesot, M.-J., Rifqi, M., and Bouchon-Meunier, B. (2008).
Fuzzy prototypes: from a cognitive view to a ma-
chine learning principle. Fuzzy sets and their exten-
sions: representation, aggregation and models. Stud-
ies in fuzziness and soft computing edition.
Levillain, F., Orero, J. O., Rifqi, M., and Bouchon-Meunier,
B. (2010). Characterizing player’s experience from
physiological signals using fuzzy decision trees. In
IEEE Conference on Computational Intelligence and
Games.
Novak, D., Mihelj, M., and Munih, M. (2012). A survey of
methods for data fusion and system adaptation using
autonomic nervous system responses in physiological
computing. Interacting with Computers, 24:154–172.
Picard, R., Vyzas, E., and Healey, J. (2001). Toward ma-
chine emotional intelligence: Analysis of affective
physiological state. IEEE Transactions Pattern Anal-
ysis and Machine Intelligence, 23:1175–1191.
PhyCS2014-InternationalConferenceonPhysiologicalComputingSystems
356