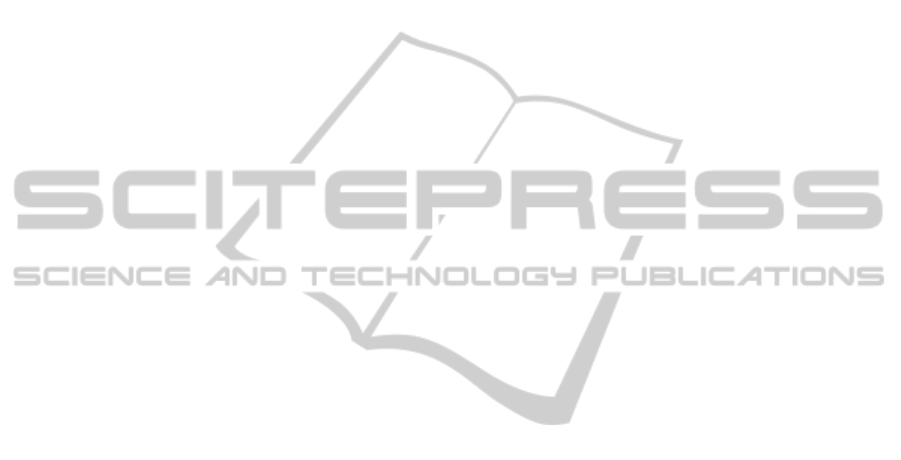
The semantic portal search module supports the
input of inaccurate user requests. Figures 3 and 4
show the search function result at the portal.
6 CONCLUSION AND
PROSPECTS FOR FUTURE
DEVELOPMENTS
The pilot project of the university’s scientific
activity semantic portal has allowed us to generate a
fragment of the university’s scientific knowledge
base and to explore the functionality of the
developed models and methods of text document
processing.
"Solid State Physics" vocabularies have been
formed by the developed methods of automatic
extraction and key terms list selection from the body
of scientific papers.
The topical classification of documents has
allowed the researchers to create the university
researchers’ profiles and to implement a
personalized search engine at the university.
The next stage of the research is the development
of the semantic portal functionality and its
implementation as a part of the university’s
scientific knowledge management system.
ACKNOWLEDGEMENTS
The work was performed under grant "The
development of an e-university's ontological
knowledge base”, state registration number
0213RK00305.
REFERENCES
Allemang, D., Hendler, J., 2011. Semantic Web for the
Working Ontologist. Morgan Kaufmann Publisher,
Burlington, USA.
Altınçay, H., Erenel, Z., 2010. Analytical evaluation of
term weighting schemes for text categorization. In
Proceedings of the Pattern Recognition Letters, 1, pp.
1310–1323.
Bolshakov, E., Klyshinsky, E., Lande D., Noskov, A.,
Peskov, O., Yagunova, E., 2011. Automatic processing
of natural language text and computational linguistics.
MIEM Publishing House, Russia, 272 p.
Braslavsky, P., Sokolov, Е., 2008. Comparison of five
methods for extraction of terms of arbitrary length. In
Proceedings of International Conference "Dialogue" -
Computational Linguistics and Intelligent
Technologies, vol. 7(14). Russia, pp. 67-74.
Ceci, F., Pietrobon, R., Gonçalves, A., 2012. Turning Text
into Research Networks: Information Retrieval and
Computational Ontologies in the Creation of Scientific
Databases. PLoS ONE, vol. 7(1), pp. 1-9.
Cherman, E. A., Monard, M.C., Metz, J., 2011. Multi-
label Problem Transformation Methods: a Case Study.
Electronic Journal CLEI, vol. 14(1), pp. 4-13.
Du, M., Chen, X., 2013. Accelerated k-nearest neighbours
algorithm based on principal component analysis for
text categorization. Journal of Zhejiang University-
Science C-Computers & Electronics, vol. 14(6), pp.
407-416.
Guarino, N., 2009. The Ontological Level: Revisiting 30
Years of Knowledge Representation. Conceptual
Modeling: Foundations and Applications, pp. 52-67.
Jiang, J., Tsai, Sh., Lee, Sh., 2012. FSKNN: Multi-label
text categorization based on fuzzy similarity and k
nearest neighbors. In Proceedings of the Expert
Systems with Applications 39, pp. 2813–2821.
Kryukov, K.V., Kuznetsov, O., Suhoverov, V., 2013. On
the notion of a formal competency researchers. In
Proceedings of III International Scientific and
Technical Conference – OSTIS-2013, pp. 143-146.
Liu, Y., Loh Han, T., Sun, A., 2009. Imbalanced text
classification: A term weighting approach. In
Proceedings of the Expert Systems with Applications,
vol. 36, pp. 690–701.
Lukashevich, N.V., 2011. Thesauri in information
retrieval tasks. Moscow University Publishing House,
Russia, 415 p.
Ma, J., Xu, W., Sun, Y., Turban, E., Wang, Sh., Liu, O.,
2012. An Ontology-Based Text-Mining Method to
Cluster Proposals for Research Project Selection.
IEEE Transactions on Systems, Man, and Cybernetics
— Part A: Systems and Humans, vol. 42(3), pp.784-
790.
Maier, R., 2007. Knowledge Management Systems:
Information and Communication Technologies for
Knowledge Management, Springer, 3rd edition.
Malarvizhi, P., Ramachandra, V.P., 2013. Multilabel
classification of documents with MAPREDUCE.
International Journal of Engineering and Technology
(IJET), pp.1260-1267.
Manning, Ch.D., Raghavan, P., Schütze, H., 2009.
Introduction to Information Retrieval. Cambridge
University Press.
Min, J., Josh, C.D., Buzhou, T., Hongxin, C., Hua, X.,
2012. Extracting semantic lexicons from discharge
summaries using machine learning and the C-Value
method. Proceeding of the AMIA Symposium, pp. 409-
416.
Pivovarova, L.M.,Yagunova, E.V., 2010. Extraction and
classification of terminological collocations on the
material of linguistic scientific texts (preliminary
observations). In Proceedings of Symposium:
"Terminology and knowledge" Russia, Moscow,
[http://webground.su/data/lit/pivovarova_yagunova/Iz
vlechenie_i_klassifikatsiya_terminoligicheskih_kollok
atsyi.pdf].
University'sScientificResourcesProcessinginKnowledgeManagementSystems
231