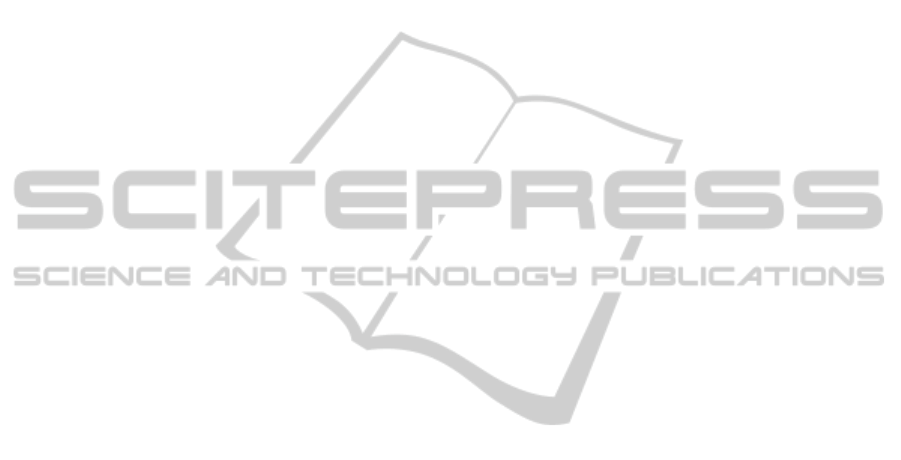
tation phase of CRISP-DM by testing the obtained
data-driven model in a real-environment (e.g., by de-
signing a friendly interface to query the RF model).
After some time, this would allow us to obtain addi-
tional feedback from the hospital managers and also
enrich the datasets by gathering more examples.
ACKNOWLEDGEMENTS
We wish to thank the physicians that participated
in this study for their valuable feedback. Also, we
would like to thank the anonymous reviewers for
their helpful suggestions. The work of P. Cortez
has been supported by FCT – Fundac¸
˜
ao para a
Ci
ˆ
encia e Tecnologia within the Project Scope: PEst-
OE/EEI/UI0319/2014.
REFERENCES
Abelha, F., Maia, P., Landeiro, N., Neves, A., and Barros,
H. (2007). Determinants of outcome in patients ad-
mitted to a surgical intensive care unit. Arquivos de
Medicina, 21(5-6):135–43.
Azari, A., Janeja, V. P., and Mohseni, A. (2012). Pre-
dicting hospital length of stay (phlos): A multi-tiered
data mining approach. In Data Mining Workshops
(ICDMW), 2012 IEEE 12th International Conference
on, pages 17–24. IEEE.
Bi, J. and Bennett, K. (2003). Regression Error Character-
istic curves. In Fawcett, T. and Mishra, N., editors,
Proceedings of 20th Int. Conf. on Machine Learning
(ICML), Washington DC, USA, AAAI Press.
Brown, M. and Kros, J. (2003). Data mining and the im-
pact of missing data. Industrial Management & Data
Systems, 103(8):611–621.
Cios, K. and Moore, G. (2002). Uniqueness of Medical
Data Mining. Artificial Intelligence in Medicine, 26(1-
2):1–24.
Clifton, C. and Thuraisingham, B. (2001). Emerging stan-
dards for data mining. Computer Standards & Inter-
faces, 23(3):187–193.
Cortez, P. (2010). Data Mining with Neural Networks and
Support Vector Machines using the R/rminer Tool. In
Perner, P., editor, Advances in Data Mining – Appli-
cations and Theoretical Aspects, 10th Industrial Con-
ference on Data Mining, pages 572–583, Berlin, Ger-
many. LNAI 6171, Springer.
Cortez, P. and Embrechts, M. J. (2013). Using sensi-
tivity analysis and visualization techniques to open
black box data mining models. Information Sciences,
225:1–17.
Fayyad, U., Piatetsky-Shapiro, G., and Smyth, P. (1996).
Advances in Knowledge Discovery and Data Mining.
MIT Press.
Freitas, A., Silva-Costa, T., Lopes, F., Garcia-Lema, I.,
Teixeira-Pinto, A., Brazdil, P., and Costa-Pereira,
A. (2012). Factors influencing hospital high length
of stay outliers. BMC Health Services Research,
12(1):265.
Freund, Y. and Schapire, R. E. (1995). A desicion-theoretic
generalization of on-line learning and an application
to boosting. In Computational learning theory, pages
23–37. Springer.
Guzman Castillo, M. (2012). Modelling patient length of
stay in public hospitals in Mexico. PhD thesis, Uni-
versity of Southampton.
Hastie, T., Tibshirani, R., and Friedman, J. (2008). The
Elements of Statistical Learning: Data Mining, Infer-
ence, and Prediction. Springer-Verlag, NY, USA, 2nd
edition.
Kalra, A. D., Fisher, R. S., and Axelrod, P. (2010). De-
creased length of stay and cumulative hospitalized
days despite increased patient admissions and read-
missions in an area of urban poverty. Journal of gen-
eral internal medicine, 25(9):930–935.
Menard, S. (2002). Applied logistic regression analysis.
Number 106. Sage.
Oliveira, A., Dias, O., Mello, M., Arajo, S., Dragosavac, D.,
Nucci, A., and Falc
˜
ao, A. (2010). Fatores associados
`
a
maior mortalidade e tempo de internac¸
˜
ao prolongado
em uma unidade de terapia intensiva de adultos. Re-
vista Brasileira de Terapia Intensiva, 22(3):250–256.
Pena, F., Soares, J., Peixoto, R., Jnior, H., Paiva, B.,
Moraes, F., Engel, P., Gomes, N., and Pena, G. (2010).
An
´
alise de um modelo de risco pr
´
e-operatrio espec-
fico para cirurgia valvar e a relac¸
˜
ao com o tempo de
internac¸
˜
ao em unidade de terapia intensiva. Revista
Brasileira de Terapia Intensiva, 22(4):339–345.
Sheikh-Nia, S. (2012). An Investigation of Standard and
Ensemble Based Classification Techniques for the
Prediction of Hospitalization Duration. Thesis for
Master Science Degree, University of Guelph, On-
tario, Canada.
Silva, A., Cortez, P., Santos, M. F., Gomes, L., and Neves,
J. (2006). Mortality assessment in intensive care units
via adverse events using artificial neural networks. Ar-
tificial Intelligence in Medicine, 36(3):223–234.
Silva, A., Cortez, P., Santos, M. F., Gomes, L., and Neves,
J. (2008). Rating organ failure via adverse events us-
ing data mining in the intensive care unit. Artificial
Intelligence in Medicine, 43(3):179–193.
Witten, I., Frank, E., and Hall, M. (2011). Data Mining:
Practical Machine Learning Tools and Techniques.
Morgan Kaufmann, San Franscico, USA, San Fran-
cisco, CA, 3rd edition.
ICEIS2014-16thInternationalConferenceonEnterpriseInformationSystems
414