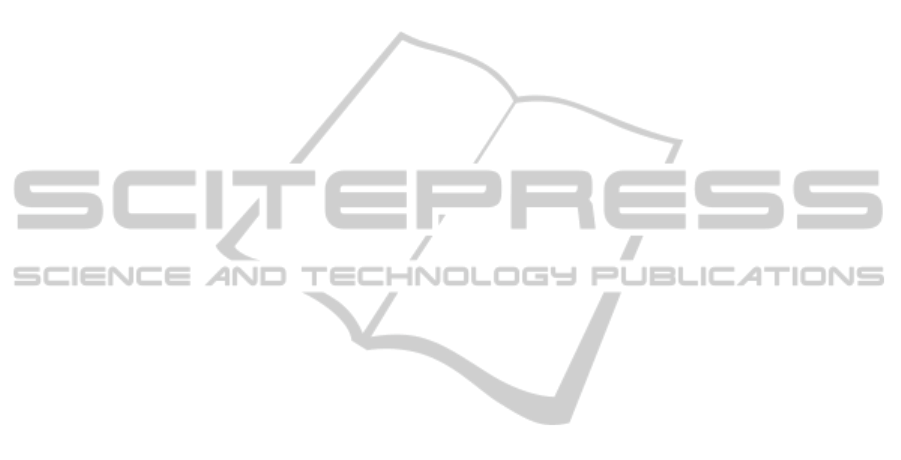
sification analysis is useful because it was possible
to separate agricultural areas from non-agricultural,
such as water, forest and urban areas.
In the second experiment, we performed the clus-
tering analysis of the same datasets. The spatial geo-
graphic visualization of the clustering results is shown
in Figure 6a) and c) and the profile visualization is
shown in Figure 6b) and d). The representative time
series chosen were the centroid of each cluster.
According to the experts, the Albedo variable was
useful to separate water areas from the other targets,
but was not sufficient to distinguish areas with differ-
ent vegetation cover. The clustering of the other areas
was defined mainly by the Surface Temperature vari-
able, being higher for targets with lower canopy, for
example, urban areas and exposed soil, and lower for
forest regions, such as the Atlantic Forest areas. The
cluster configuration varied from year to year because
the weather also varied over the last decade, influenc-
ing the values of Surface Temperature.
5 CONCLUSION
This paper presented the SITSMining framework, an
automated solution to data mining based analysis of
satellite image time series. As the need for knowledge
discovery in large remote sensing databases grows,
the framework is shown as a powerful computational
tool for the experts, as it provides resources such as
data extraction from multitemporal satellite images,
analysis of large datasets through data mining tech-
niques and output formatting in an integrated environ-
ment. Because of its modular architecture, the frame-
work allows the addition of new methods for noise
replacement, classification and clustering based anal-
ysis, output formatting, as well as the incorporation
of new data mining task modules.
The experimental analysis performed shows that
the framework is useful to support researches in agri-
culture domain of application, even considering low
spatial resolution satellite images. In future work, we
aim to fully integrate the SITSMining framework to
the SatImagExplorer tool, to provide for the experts
in agrometeorology, the possibility to perform extrac-
tion of time series from multitemporal satellite im-
ages, data mining analysis and output visualization in
an integrated system under the same platform.
ACKNOWLEDGEMENTS
We thank to CNPq, FAPESP, CAPES, Embrapa-
Campinas for financial support.
REFERENCES
Berndt, D. and Clifford, J. (1994). Using dynamic time
warping to find patterns in time series. In AAAI Work-
shop on Knowledge Discovery in Databases, pages
359–370, Seattle - Washington.
Chino, D. Y. T., Amaral, B. F., Romani, L. A. S., Sousa, E.
P. M., and Traina, A. J. M. (2011). Satimagexplorer:
tornando a minerac¸
˜
ao de dados de sensores orbitais
mais flex
´
ıvel. In VIII SBBD, pages 25–30, Brasil.
Csiszar, I. and Gutman, G. (1999). Mapping global land
surface albedo from noaa avhrr. Journal of Geophysi-
cal Research, 104(d6):6215–6228.
Freitas, R. M., Arai, E., Adami, M., Souza, A. F., Sato, F. Y.,
Shimabukuro, Y. E., Rosa, R. R., Anderson, L. O., and
Rudorff, B. F. T. (2011). Virtual laboratory of remote
sensing time series: visualization of modis evi2 data
set over south america. Journal of Computational In-
terdisciplinary Sciences, 2(1):57–68.
Han, J. and Kamber, M. (2000). Data Mining: Concepts
and Techniques. Morgan Kaufmann, San Francisco.
Julea, A., M
´
eger, N., Bolon, P., Rigotti, C., Doin, M.-P.,
Lasserre, C., Trouv
´
e, E., and Lazarescu, V. N. (2011).
Unsupervised spatiotemporal mining of satellite im-
age time series using grouped frequent sequential pat-
terns. Geoscience and Remote Sensing, IEEE Trans-
actions on, 49(4):1417–1430.
Keogh, E. J. and Pazzani, M. J. (1998). An enhanced repre-
sentation of time series which allows fast and accurate
classification, clustering and relevance feedback. In
KDD 1998, volume 98, pages 239–243.
Kyrgyzov, I. O., Maitre, H., and Campedel, M. (2007). A
method of clustering combination applied to satellite
image analysis. In Image Analysis and Processing,
2007. 14th International Conference on, pages 81–86.
Maimon, O. and Rokach, L. (2005). The Data Mining and
Knowledge Discovery Handbook. Springer.
Mitsa, T. (2010). Temporal Data Mining. Chapman &
Hall/CRC, 1st edition.
Rouse, J. W., Haas, R. H., Schell, J. A., and Deering, D. W.
(1973). Monitoring vegetation systems in the Great
Plains with ERTS, volume 1, pages 309–317. NASA.
Vaduva, C., Costachioiu, T., Patrascu, C., Gavat, I.,
Lazarescu, V., and Datcu, M. (2011). Classification of
dynamic evolutions from satellitar image time series
based on similarity measures. In Analysis of Multi-
temporal Remote Sensing Images (Multi-Temp), 2011
6th International Workshop on the, pages 141–144.
Vlachos, M., Hadjieleftheriou, M., Gunopulos, D., and
Keogh, E. (2003). Indexing multi-dimensional time-
series with support for multiple distance measures. In
KDD 2003, pages 216–225, New York.
ICEIS2014-16thInternationalConferenceonEnterpriseInformationSystems
232