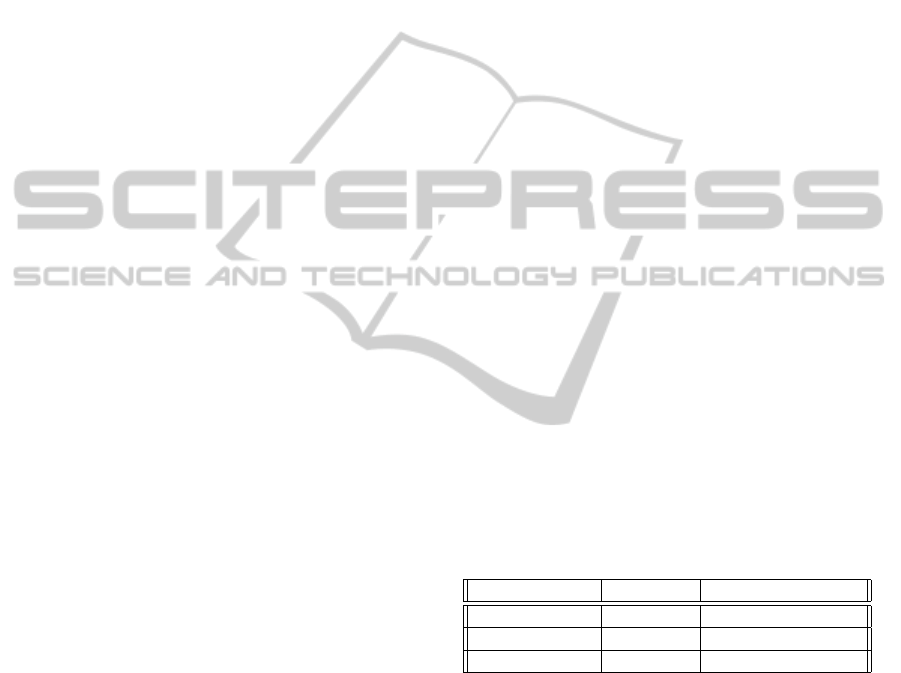
A vocal system has also been implemented and
can be activated to additionally assist the driver during
the flight. It uses the result from the image processing
system to determine the appropriate message. The vo-
cal assistance system is used to warn the driver about
the quadromotor position (on/off track) and to an-
nounce the next marker when a new targeted marker
became available. It also helps the driver to keep track
of the timing.
For the retrieval of heart rate data and skin con-
ductance data of the driver we used the same system
as described in Section 2.1.
3.2 Subjects
The participants are recruited through local commu-
nity. Seven subjects participated (1 female and 6
males), their age varies between 24 to 33, with a mean
age of 27. They all have technical background. One
of them did not participate in the previous experiment
with the simulated driving system.
3.3 Procedure
Before starting the experiment, the participants were
asked to fill-up a pre-study questionnaire about their
demographic information. After that, a short intro-
duction about the experiment context and the setting
was made. The physiological sensors are then at-
tached to the body of the participant for the real-time
measurements of the heart rate and the skin conduc-
tance signals. The participants are given about 20
minutes to learn to fly the quadromotor with the Log-
itech’s G27 Racing Wheel and to get used to the driv-
ing setting.
The main experiment consisted of 5 minutes of
music relaxation and four times of 5-minutes flight.
We designed four different conditions: in terms of dif-
ficulty level (easy trajectory vs. difficult trajectory)
and vocal assistance (vocal assistance activated vs.
vocal assistance not-activated). Trajectories are pre-
defined and announced to the participant before the
beginning of each flight. The participant is asked to
finish each trajectory in 5 minutes or less. If he/she
finishes the trajectory before 5 minutes he/she can
pass to the next condition. The order of the four con-
ditions are changed from one participant to another.
After each condition, the participant is asked to an-
swer a questionnaire about his/her emotional impres-
sion about the flight before beginning the next flight.
At the end of the experiment, the participants are
asked to fill-up a last questionnaire about his/her over-
all impression about the system.
3.4 Hypothesis
With the experiment of driving in a real environment,
we want to test the following hypotheses:
• Hypothesis 1. Participants’ heart rate should cor-
relate with the difficulty level of the driving task.
The more difficult the task is, the higher the heart
rate level is.
• Hypothesis 2. The participants’ skin conductiv-
ity should correlate with the repetitiveness of the
driving task. The more repetitive the driving tra-
jectory is, the lower the level of the skin conduc-
tance is.
• Hypothesis 3. The variability of the participants’
physiological signals between task conditions in
the real world environment experiment should be
greater than the variability of these signals in the
simulated environment experiment.
3.5 Data Analysis and Discussion
As stated in the previous section, for the purpose of
this paper, we will present only the results of the phys-
iological signals collected from the experiment. The
average skin conductance level and the average heart
rate of each participant in the drone driving condition
are depicted in Fig. 6 and Fig. 7. The overall aver-
age of these physiological signal data is presented in
Table 2.
Table 2: Overall average (and standard deviation) of the
physiological signals (heart rate in Beat Per Minute and skin
conductance in MicroSiemens) from the experiment of driv-
ing the quadromotor drone in a real environment.
Physio. Signal Heart rate Skin Conductance
Baseline 79 (5) 3.66 (3.1)
Easy level 82 (8) 5.08 (3.2)
Difficult level 84 (9) 4.69 (3.3)
Interestingly, the heart rate and the skin conduc-
tance level of the participants collected during this ex-
periment show the same phenomenon as in the previ-
ous experiment with the simulated driving environ-
ment. This also supports our two hypotheses (i.e.
Hypothesis 1 and Hypothesis 2) about the activity of
these physiological signals for this experiment. It is
observable from Figure 6, Figure 7, and from Table
2) that heart rate correlates positively with the diffi-
culty level of the flight and the skin conductance sig-
nal correlates negatively with the difficulty level of the
flight. Moreover, the variation of physiological sig-
nals in this experiment is larger than in the previous
experiment. This can show that human subjects have
higher internal reactions in real situations rather than
PhyCS2014-InternationalConferenceonPhysiologicalComputingSystems
382