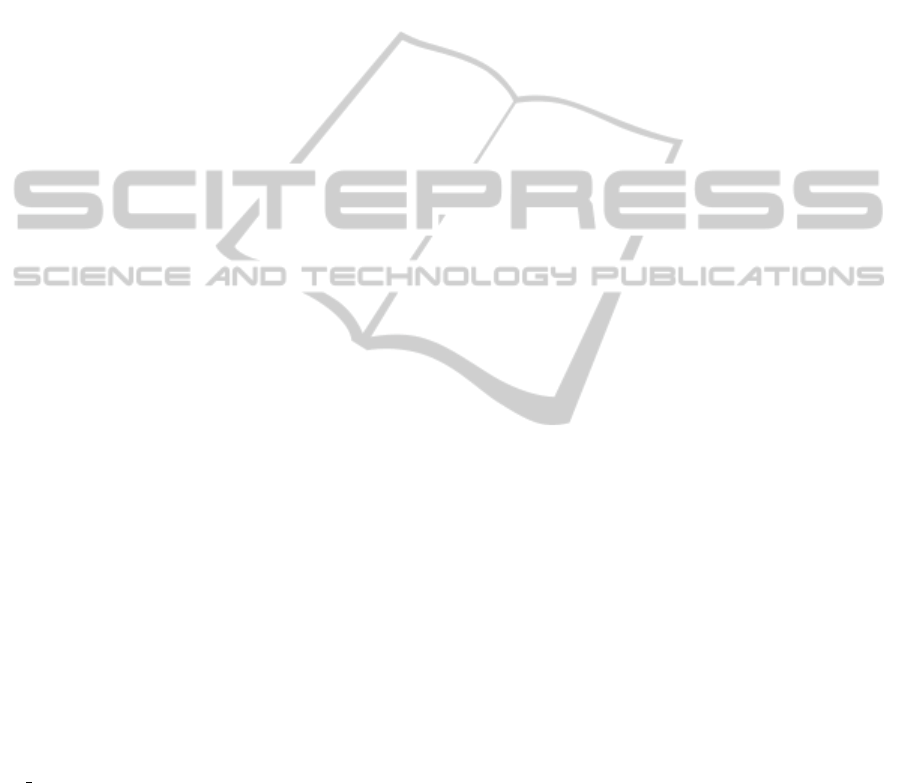
the quality of 3D models reconstructed by the origi-
nal KinFu algorithm in comparison with our distance-
aware DA and DASS methods. The experiments have
revealed that the input from stereo sensors is valid
and sufficient for KinFu-based algorithms, resulting
in an appropriate reconstruction of outdoor scenes.
We have also shown that by replacing the original
KinFu weighting strategy by distance-aware weight-
ing strategies, we obtain 3D models from stereo
data with higher quality and more accurate pose-
estimation values. In our experiments, the new strate-
gies increase the endurance of the reconstruction pro-
cess with a factor of two or more.
Comparing the depth data obtained from the
Kinect and stereo sensors, we have found that the
stereo camera is able to provide more continuous
depth data for scenes with sufficient visual features
that interfere the IR patterns of the Kinect sensor, such
as black or shiny surfaces. Alternatively, the Kinect
can provide more continuous depth data for the sur-
faces with insufficient amount of visual features or
featureless surfaces, where stereo cameras are unable
to extract any depth information.
For future work, we plan experiments on finding
the optimal hybrid system capable of working in dif-
ferent environments in terms of the quantity and qual-
ity of visual and 3D features and intelligently fusing
the resulting data from depth sensor and stereo cam-
era, based on the scene configuration and features.
ACKNOWLEDGEMENTS
This research has been performed within the
PANORAMA project, co-funded by grants from Bel-
gium, Italy, France, the Netherlands, the United King-
dom, and the ENIAC Joint Undertaking.
REFERENCES
Asus. Xtion-PRO. http://www.asus.com/Multimedia/Xtion
PRO/.
Bondarev, E., Heredia, F., Favier, R., Ma, L., and de With, P.
(2013). On photo-realistic 3d reconstruction of large-
scale and arbitrary-shaped environments. In Con-
sumer Communications and Networking Conference
(CCNC), 2013 IEEE, pages 621–624.
Chow, J., Ang, K., Lichti, D., and Teskey, W. (2012). Per-
formance analysis of a low-cost triangulation-based
3D camera: Microsoft kinect system. In Interna-
tional Archives of the Photogrammetry, Remote Sens-
ing and Spatial Information Sciences, Volume XXXIX-
B5, 2012 XXII ISPRS Congress, Melbourne, Aus-
tralia.
Curless, B. and Levoy, M. (1996). A volumetric method for
building complex models from range images. In CM
SIGGRAPH Conf. Proceedings, pp. 303–312 (1996).
Engelhard, N., Endres, F., Hess, J., Strum, J., and Burgard,
W. (2011). Real-time 3d visual slam with a hand-held
camera. In RGB-D Workshop on 3D Perception in
Robotics, European Robotics Forum.
Izadi, S., Kim, D., Hilliges, O., Molyneaux, D., Newcombe,
R., Kohli, P., Shotton, J., Hodges, S., Freeman, D.,
Davison, A., and Fitzgibbon, A. (2011). Kinectfu-
sion: Real-time 3d reconstruction and interaction us-
ing a moving depth camera. In Proceedings of the
24th Annual ACM Symposium on User Interface Soft-
ware and Technology, UIST ’11, pages 559–568, New
York, NY, USA. ACM.
Javan Hemmat, H., Bondarev, E., and de With, P. (2014a).
Exploring distance-aware weighting strategies for ac-
curate reconstruction of voxel-based 3d synthetic
models. In MMM’14 Proceedings of Multi-Media
Modelling 2014 (will be presented) (2014).
Javan Hemmat, H., Bondarev, E., Dubbelman, G., and
de With, P. (2014b). Improved icp-based pose estima-
tion by distance-aware 3d mapping. In VISAPP’14,
Proceedings of 9th International Conference on Com-
puter Vision Theory and Application (will be pre-
sented) 2014.
Khoshelham, K. (2011). Accuracy analysis of kinect depth
data. In ISPRS workshop laser scanning / ed. by D.D.
Lichti and A.F. Habib., IAPRS XXXVIII-5/W12 (2011).
Khoshelham, K. and Elberink, S. O. (2012). Accuracy and
resolution of kinect depth data for indoor mapping ap-
plications. Sensors, 12(2):1437–1454.
Kinect, M. http://www.xbox.com/en-us/kinect/.
Newcombe, R., Lovegrove, S., and Davison, A. (2011a).
Dense tracking and mapping in real-time. In ICCV’11,
IEEE International Conference on Computer Vision,
Spain.
Newcombe, R. A., Davison, A. J., Izadi, S., Kohli, P.,
Hilliges, O., Shotton, J., Molyneaux, D., Hodges, S.,
Kim, D., and Fitzgibbon, A. (2011b). Kinectfusion:
Real-time dense surface mapping and tracking. In
Mixed and Augmented Reality (ISMAR), 2011 10th
IEEE International Symposium on, pages 127–136.
PCL (2011). Kinectfusion implementation in the PCL.
http://svn.pointclouds.org/pcl/trunk/.
PrimeSense. http://www.primesense.com/.
Steinbrucker, F., Sturm, J., and Cremers, D. (2011). Real-
time visual odometry from dense rgb-d images. In
IEEE International Conference on Computer Vision
Workshops (ICCV Workshops), pages 719–722.
Whelan, T., Johannsson, H., Kaess, M., Leonard, J., and
McDonald, J. (2012a). Robust tracking for real-time
dense rgb-d mapping with kintinuous. In MIT techni-
cal report, MIT-CSAIL-TR-2012-031.
Whelan, T., Johannsson, H., Kaess, M., Leonard, J., and
McDonald, J. (2013). Robust real-time visual odome-
try for dense RGB-D mapping. In IEEE Intl. Conf. on
Robotics and Automation, ICRA, Karlsruhe, Germany.
Whelan, T., Kaess, M., Fallon, M., Johannsson, H.,
Leonard, J., and McDonald, J. (2012b). Kintinuous:
Spatially extended kinectfusion. In RSS Workshop on
RGB-D: Advanced Reasoning with Depth Cameras,
Sydney, Australia.
EvaluationofDistance-AwareKinFuAlgorithmforStereoOutdoorData
751