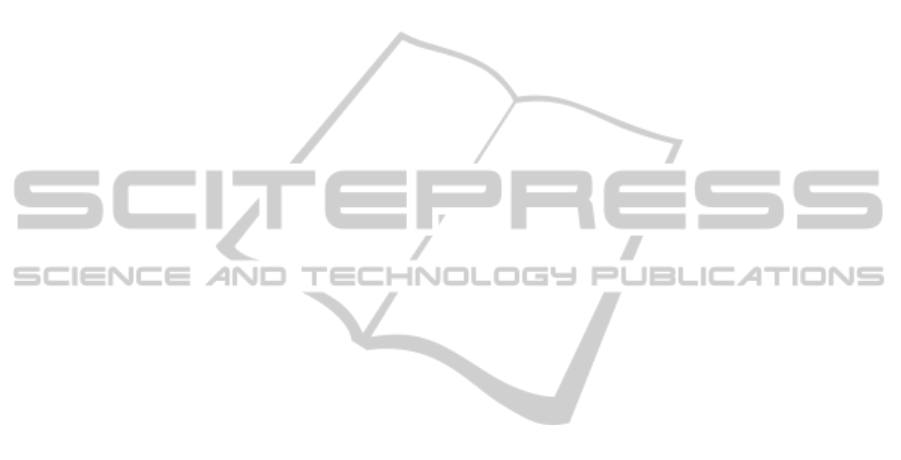
consensual clinical definitions of HAIs. They are
connected to an automated inference engine based
on fuzzified Arden Syntax, which is adopted as an
industry standard by HL7. They serve as intelligent
tools that can on the one hand be adapted to varying
or newly emerging inputs, and on the other hand to
changing output demands. In this way, they are
“living” intelligent ICT systems, responsive to
environmental changes.
REFERENCES
Adlassnig, K.-P., 1982. A survey on medical diagnosis
and fuzzy subsets. In Gupta, M.M. and Sanchez, E.
(eds.), Approximate Reasoning in Decision Analysis.
Amsterdam: North-Holland Publishing Company,
203-217.
Adlassnig, K.-P., Blacky, A., and Koller, W., 2008.
Fuzzy-based nosocomial infection control. In
Nikravesh, M., Kacprzyk, J., and Zadeh, L. (eds.),
Forging New Frontiers: Fuzzy Pioneers II. Berlin:
Springer, 343-349.
Adlassnig, K.-P. and Rappelsberger, A., 2008. Medical
knowledge packages and their integration into health-
care information systems and the World Wide Web. In
Andersen, S. K., Klein, G. O., Schulz, S., Aarts, J.,
and Mazzoleni, M. C. (eds.) eHealth Beyond the
Horizon–Get IT There. Proceedings of the 21st
International Congress of the European Federation
for Medical Informatics (MIE 2008). Studies in Health
Technology and Informatics, 136, Amsterdam: IOS
Press, 121-126.
Adlassnig, K.-P., Blacky, A., and Koller, W., 2009.
Artificial-intelligence-based hospital-acquired
infection control. In Bushko, R. (ed.) Strategy for the
Future of Health. Studies in Health Technology and
Informatics, 149, Amsterdam: IOS Press, 103-110.
ANISS Surveillance. http://www.meduniwien.ac.at/
hp/krankenhaushygiene/forschung-lehre/aniss-
surveillance/, Nov 3, 2013.
AUQIP http://www.ipg.uni-linz.ac.at/fr_leiste_proj.htm,
Nov. 3, 2013.
Blacky, A., Mandl, H., Adlassnig, K.-P., and Koller, W.,
2011. Fully automated surveillance of healthcare-
associated infections with MONI-ICU – A
breakthrough in clinical infection surveillance. Applied
Clinical Informatics, 2(3), 365-372.
Centers for Disease Control and Prevention, 2013a.
National Healthcare Safety Network (NHSN).
http://www.cdc.gov/nhsn/, Nov 4, 2013.
Centers for Disease Control and Prevention, 2013b.
National Healthcare Safety Network NHSN,
Surveillance for Ventilator-associated events.
www.cdc.gov/nhsn/acute-care-hospital/vae/, Nov 4,
2013.
Centers for Medicare & Medicaid Services, 2013.
Medicare.gov, The Official U.S. Government Site for
Medicare. http://www.medicare.gov/hospitalcompare/
?AspxAutoDetectCookieSupport=1, Nov 3, 2013.
Chizzali-Bonfadin, C., Adlassnig, K.-P., and Koller, W.
MONI: An intelligent database and monitoring system
for surveillance of nosocomial infections. In: Greenes,
R. A., Peterson, H. E., and Protti D. J. (eds), 1995.
MEDINFO 95. Proceedings of the Eighth World
Congress on Medical Informatics; Jul 23-27;
Vancouver, Canada. Canada: Healthcare Computing &
Communications Canada, Inc., 1684.
de Bruin, J., Adlassnig, K.-P., Blacky, A., Mandl, H.,
Fehre, K., and Koller, W., 2013. Effectiveness of an
automated surveillance system for intensive care unit-
acquired infections. Journal of the American Medical
Informatics Association, 20(2), 369-372.
European Centre for Disease Prevention and Control,
2013. http://www.ecdc.europa.eu/en/Pages/home.aspx,
Nov 4, 2013.
Freeman R., Moore, L. S. P., Garcia Alvarez, L., Charlett,
A., Holmes, A., 2013. Advances in electronic
surveillance for healthcare-associated infections in the
21st Century: A systematic review. Journal of
Hospital Infection
, 84(2), 106-119.
German National Center for Surveillance of Nosocomial
Infections, 2013a. KISS (Krankenhaus-Infektions-
Surveillance-System). http://www.nrz-hygiene.de/
surveillance/kiss/, Nov 3, 2013.
German National Center for Surveillance of Nosocomial
Infections, 2013b. NEO-KISS. http://www.nrz-
hygiene.de/surveillance/kiss/neo-kiss/, Nov 3, 2013.
Health Level Seven, Inc., 2013. The Arden Syntax for
Medical Logic Systems Version 2.9, Ann Arbor, MI:
Health Level Seven Inc. http://www.hl7.org/
implement/standards/product_brief.cfm?product_id=2
90, Nov 3, 2013.
Hripcsak, G., 1994. Writing Arden Syntax Medical Logic
Modules. Computers in Biology and Medicine, 24(5),
331-363.
IQIP International Quality Indicator Project.
http://www.internationalqip.com/, Nov 3, 2013.
Samwald, M., Fehre, K., De Bruin, J., and Adlassnig, K.-
P., 2012. The Arden Syntax standard for clinical
decision support: Experiences and directions. Journal
of Biomedical Informatics, 45(4), 711-718.
Vermont-Oxford Network, 2013. http://
www.vtoxford.org/home.aspx, Nov 3, 2013.
Zadeh, L. A., 1965. Fuzzy sets. Information and Control,
8(3), 338-353.
HEALTHINF2014-InternationalConferenceonHealthInformatics
404