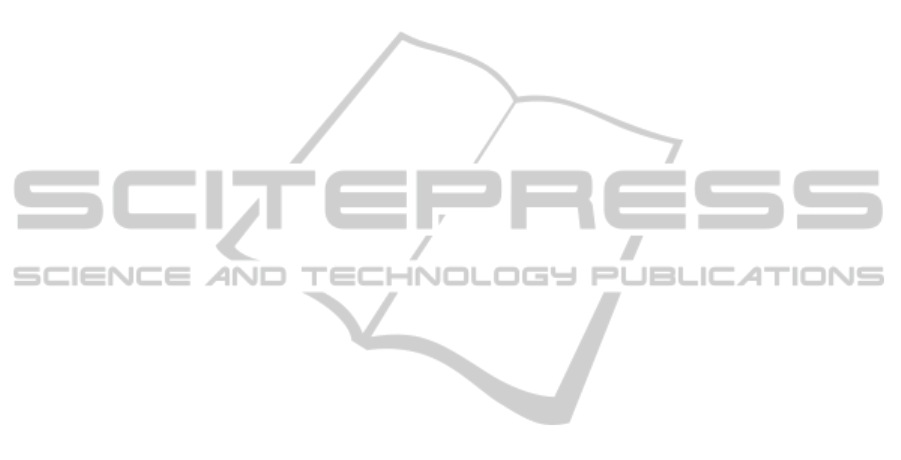
OE/EEI/LA0021/2013, by the project EMOTE from
the EU 7
th
Framework Program (FP7/2007-2013) un-
der grant agreement n.317923, and under the PhD
grant SFRH/BD/75924/2011.
REFERENCES
Andreassi, J. (2007). Psychophysiology: Human Behavior
And Physiological Response. Lawrence Erlbaum.
Berthold, M. and Hand, D. J., editors (1999). Intelligent
Data Analysis: An Introduction. Springer-Verlag New
York, Inc., Secaucus, NJ, USA, 1st edition.
Bos, D. O. (2006). Eeg-based emotion recognition the
influence of visual and auditory stimuli. Emotion,
57(7):1798–806.
Brand, M. (1999). Structure learning in conditional proba-
bility models via an entropic prior and parameter ex-
tinction. Neural Comput., 11(5):1155–1182.
Brown, M., Hughey, R., Krogh, A., Mian, I. S., Sj
¨
olander,
K., and Haussler, D. (1993). Using dirichlet mix-
ture priors to derive hidden markov models for protein
families. In Int. Sys. for Molecular Bio., pages 47–55.
AAAI Press.
Burges, C. J. C. (1998). A tutorial on support vector
machines for pattern recognition. Data Mining and
Knowledge Discovery, 2:121–167.
Cacioppo, J., Tassinary, L., and Berntson, G. (2007). Hand-
book of psychophysiology. Cambridge University
Press.
Ekman, P. and W., F. (1988). Universals and cultural differ-
ences in the judgments of facial expressions of emo-
tion. Personality and Social Psychology, 53:712–717.
Exarchos, T., Tsipouras, M., Papaloukas, C., and Fotiadis,
D. (2008). A two-stage methodology for sequence
classification based on sequential pattern mining and
optimization. Data Knowl. Eng., 66(3):467–487.
Geurts, P. (2001). Pattern extraction for time series clas-
sification. In PKDD, pages 115–127, London, UK.
Springer-Verlag.
Guimar
˜
aes, G. (2000). The induction of temporal grammat-
ical rules from multivariate time series. In Colloquium
on Grammatical Inference: Alg. and App., pages 127–
140, London, UK. Springer-Verlag.
Haag, A., Goronzy, S., Schaich, P., and Williams, J. (2004).
Emotion recognition using bio-sensors: First steps to-
wards an automatic system. In Affective D.Sys., vol-
ume 3068 of LNCS, pages 36–48. Springer.
Han, J., Cheng, H., Xin, D., and Yan, X. (2007). Frequent
pattern mining: current status and future directions.
Data Min. Knowl. Discov., 15(1):55–86.
Henriques, R. and Antunes, C. (2014). Learning predictive
models from integrated healthcare data: Capturing
temporal and cross-attribute dependencies. In HICSS.
IEEE.
Henriques, R. and Paiva, A. (2014). Seven principles to
mine flexible behavior from physiological signals for
effective emotion recognition and description in affec-
tive interactions. In PhyCS.
Henriques, R., Paiva, A., and Antunes, C. (2012). On the
need of new methods to mine electrodermal activity
in emotion-centered studies. In AAMAS’12, 8th IW on
ADMI. Springer-Verlag LNAI series.
Henriques, R., Paiva, A., and Antunes, C. (2013). Access-
ing emotion patterns from affective interactions using
electrodermal activity. In Affective Comp. and Intel.
Interaction (ACII). IEEE Computer Society.
Jerritta, S., Murugappan, M., Nagarajan, R., and Wan, K.
(2011). Physiological signals based human emotion
recognition: a review. In CSPA, 2011 IEEE 7th Inter-
national Colloquium on, pages 410 –415.
Kulic, D. and Croft, E. A. (2007). Affective state estimation
for human-robot interaction. Trans. Rob., 23(5):991–
1000.
Lang, P. (1995). The emotion probe: Studies of motivation
and attention. American psychologist, 50:372–372.
Leite, I., Henriques, R., Martinho, C., and Paiva, A. (2013).
Sensors in the wild: Exploring electrodermal activ-
ity in child-robot interaction. In HRI, pages 41–48.
ACM/IEEE.
Lessard, C. S. (2006). Signal Processing of Random Physi-
ological Signals. S.Lectures on Biomedical Eng. Mor-
gan and Claypool Publishers.
Li, W., Han, J., and Pei, J. (2001). Cmar: Accurate and effi-
cient classification based on multiple class-association
rules. In ICDM, pages 369–376. IEEE CS.
Liu, H. and Motoda, H. (1998). Feature Selection for
Knowledge Discovery and Data Mining. Kluwer Aca-
demic Pub.
Maaoui, C., Pruski, A., and Abdat, F. (2008). Emotion
recognition for human-machine communication. In
IROS, pages 1210 –1215. IEEE/RSJ.
Madeira, S., Teixeira, M. N. P. C., S
´
a-Correia, I., and
Oliveira, A. (2010). Identification of regulatory mod-
ules in time series gene expression data using a lin-
ear time biclustering algorithm. IEEE/ACM TCBB,
1:153–165.
Madeira, S. C. and Oliveira, A. L. (2004). Bicluster-
ing algorithms for biological data analysis: A sur-
vey. IEEE/ACM Trans. Comput. Biol. Bioinformatics,
1(1):24–45.
Mitsa, T. (2009). Temporal Data Mining. DMKD. Chapman
& Hall/CRC.
M
¨
orchen, F. (2006). Time series knowledge mining. Wis-
senschaft in Dissertationen. G
¨
orich & Weiersh
¨
auser.
Mueen, A., Keogh, E. J., Zhu, Q., Cash, S., and Westover,
M. B. (2009). Exact discovery of time series motifs.
In SDM, pages 473–484.
Murphy, K. (2002). Dynamic Bayesian Networks: Repre-
sentation, Inference and Learning. PhD thesis, UC
Berkeley, CS Division.
Nanopoulos, A., Alcock, R., and Manolopoulos, Y. (2001).
Information processing and technology. chapter
Feature-based classification of time-series data, pages
49–61. Nova Science Pub.
Oatley, K., Keltner, and Jenkins (2006). Understanding
Emotions. Blackwell P.
Picard, R. W. (2003). Affective computing: challenges.
International Journal of Human-Computer Studies,
59(1-2):55–64.
DescriptiveModelsofEmotion-LearningUsefulAbstractionsfromPhysiologicalResponsesduringAffectiveInteractions
399