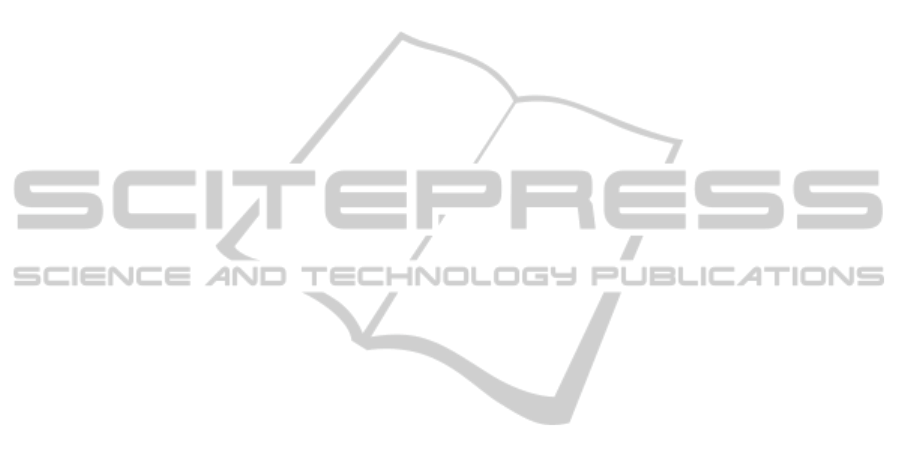
First, Fig. 8 shows ApEn images that were computed
for m = 2, M = 9 (i.e. N = 81) and different values of
r.
It can be noticed in Fig. 8 that again, it exists a
value of r leading to visual interesting results in terms
of the emphasis of cell boundaries: more precisely,
for r = 0.2, a good compromise is obtained between
noise removal and emphasis of cell boundaries (mem-
brane). This result, illustrated in Fig. 8.(d), can be
compared with Fig. 8.(f) showing the classic gradient
image.
About segmentation results using ApEn in GAC
framework, we only present here some first results
obtained on some crops of an original actin-tagged
microscopy image. Initialization strategy is still the
same as the previous one, a square-curve surrounding
the cell to segment (Fig. 9).
These first results are, from our point of view,
quite encouraging when considering the challenging
task. Fig. 9 shows that it is possible to properly seg-
ment cells even in case where visually the boundary
information is not present within the original image
(illustrations (d) and (e) for instance). Same Figure
also shows some limitations of the proposed criterion
that is ApEn when considering subfigure (f). In this
case, the size (M = 9) of the kernel used for the com-
putation of the ApEn image introduced some uncer-
tainty on the precise location of the cell boundary and
lead to an approximative result of segmentation.
5 CONCLUSIONS AND
PERSPECTIVE
In this article, we introduced an original approach for
image segmentation using GAC framework and Ap-
proximate Entropy (ApEn) estimation used as an edge
detector function. Results are presented on both syn-
thetic and real image, focusing for this latter part on
a particular application that is segmentation of cell
boundaries in actin-tagged microconfocal images.
As a proof a feasibility, this contribution is a first
step towards a better understanding of ApEn for a
possible use in image processing and more precisely
as a possible criterion for active contour segmenta-
tion. Compared to the former work of Parker et al.
(Parker et al., 1999) this preliminary study investi-
gates the influence of the different parameters used for
computation of ApEn in an image processing context,
which was not proposed before.
Main forthcoming perspective will consist in
quantitatively estimate the performance of ApEn with
respect to the different parameters r, m, and N (via the
choice made for the size M of the square-window) in
order to possibly go towards an automatic optimiza-
tion of them (r above all). From the application per-
spective, if the first results are quite encouraging, we
must now proposed a more adapted strategy in terms
of initialization in order to be able to segment all the
cells in paralell. Moreover, a clinical validation will
be also necessary to validate the segmentation pro-
cess.
REFERENCES
(2011). Image filtering using anisotropic structure tensor
for cell membrane enhancement in 3d microscopy.
In Proceedings of International Conference on Image
Processing ICIP 2011, pages 2085–2088.
Goldenberg, R., Kimmel, R., Rivlin, E., and Rudzsky,
M. (2001). Fast Geodesic Active Contours.
IEEE TRANSACTIONS ON IMAGE PROCESSING,
10(10):1467–75.
Hall, A. (2009). The cytoskeleton and cancer, volume 28.
Springer Netherlands, Philadelphia, PA, USA.
Histace, A., Meziou, L., Matuszewski, B., Precioso, F.,
Murphy, M., and Carreiras, F. (2013). Statistical re-
gion based active contour using a fractional entropy
descriptor: Application to nuclei cell segmentation in
confocal microscopy images. Annals of British Ma-
chine Vision Association, 2013(5):1–15.
Matuszewski, B., Murphy, M., Burton, D., Marchant, T.,
Moore, C., Histace, A., and Precioso, F. (2011). Seg-
mentation of Cellular Structures in Actin Tagged Flu-
orescence Confocal Microscopy Images. In IEEE
ICIP 2011, pages pp. 3081–3084, Bruxelles, Belgium.
McKinley, R. A., McIntire, L. K., Schmidt, R., Repperger,
D. W., and Caldwell, J. A. (2011). Evaluation of eye
metrics as a detector of fatigue. Human Factors: The
Journal of the Human Factors and Ergonomics Soci-
ety, 53(4):403–414.
Meziou, L., Histace, A., Precioso, F., Matuszewski, B.,
and Carreiras, F. (2012). 3D Confocal Microscopy
data analysis using level-set segmentation with alpha-
divergence similarity measure. In International Con-
ference on Computer Vision Theory and Applications,
pages 861–864, Rome, Italy.
Meziou, L., Histace, A., Precioso, F., Matuszewski, B.,
and Murphy, M. (2011). Confocal Microscopy Seg-
mentation Using Active Contour Based on Alpha-
Divergence. In Proceedings of ICIP 2011, pages
3138–3141.
Mosaliganti, K., Gelas, A., Gouaillard, A., Noche, R., Ob-
holzer, N., and Megason, S. (2009). Detection of spa-
tially correlated objects in 3d images using appear-
ance models and coupled active contours. In Proceed-
ings of MICCAI’09, pages 641–648, Berlin, Heidel-
berg. Springer-Verlag.
Ortiz De Solorzano, C., Garcia Rodriguez, E., Jones, A.,
Pinkel, D., Gray, J. W., Sudar, D., and Lockett, S. J.
(1999). Segmentation of confocal microscope images
of cell nuclei in thick tissue sections. Journal of Mi-
croscopy, 193(3):212–226.
BIOSIGNALS2014-InternationalConferenceonBio-inspiredSystemsandSignalProcessing
276