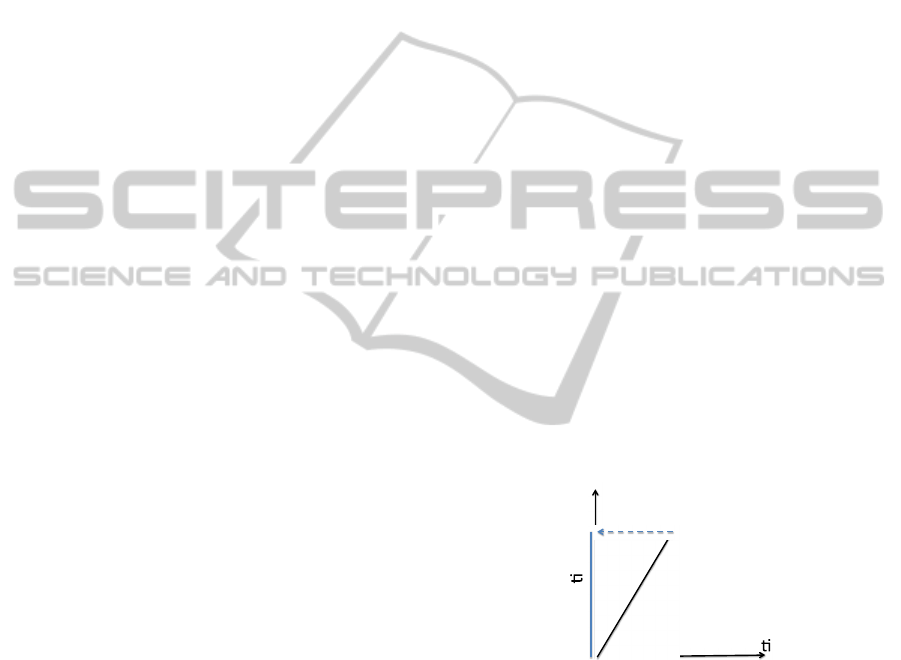
2 BASIS VECTORS AND
BEHAVIORAL STATE
In quantum probability theory a vector space
(technically, a Hilbert space) represents all possible
outcomes for questions we could ask about a system.
A basis is a set of linearly independent vectors that,
in linear combination, can represent every vector in
the vector space. They represent the coordinate
system and correspond to elementary observations.
Put it another way, the intersection of all subspaces
containing the basis vectors, that is, their linear span,
constitutes the vector space. A vector represents the
state of the system, given by the superposition of the
basis vectors according to their coefficients (Hughes,
1989; Isham, 1989). Historically, quantum
probability has been applied to physical systems but
the same analysis can refer to other types of systems,
including animals and software agents. At the end of
the day, animals are behavior systems –sets of
behaviors that are organized around biological
functions and goals, e.g., feeding (Timberlake and
Silva, 1995), defense (Fanselow, 1994), or sex
(Domjan, 1994). Software agents, on the other hand,
are formally defined as systems that (learn to) act in
virtual environments. Not surprisingly,
reinforcement learning in software agents has taken
concepts and methods from operant conditioning
theory. In turn, the former, software learning agents,
can be understood as computational models of the
latter, operant conditioning.
We define two basis vectors according to the
dichotomies reinforcement vs. punishment and
positive vs. negative in Fig. 1. The former, that we
call Frequency, takes values ranging from a
maximum number of responses per unit time
(Reinforcement) to the absence of response
(Punishment); the latter, that we call Applies, takes
values from “the response always applies the
outcome” (Positive) to “the response always
removes the outcome” (Negative). The values in
between indicate various response frequencies, that
is, probabilities that the animal responds, and
various probabilities that the outcome follows the
response, respectively.
The relation of the two bases is undetermined, in
the sense that even in the simplest reinforcement
schedules (fixed/variable ratio/interval schedules)
we cannot observe with certainty how the response
affects the outcome and how the outcome affects the
frequency of responding at the same time. This
uncertainty is aggravated in more complex
compound schedules.
The problem is thus how to determine the
behavioral state of an animal given this uncertainty.
Several models have been proposed to explain
patterns of operant behavior, some of which use
probabilities (see (Staddon and Cerutti, 2003) for a
recent survey). We argue that the inherent
uncertainty in operant conditioning cannot be
represented using classical probability (Kolmogorov,
1933), and that we need quantum probability
instead.
The behavioral state of the animal is represented
using the state vector, a unit length vector, denoted
as |Ψ in bra-ket notation. We need to find out which
linear combination of the basis vectors results in a
given behavioral state and with which probability.
We start with a single question in Fig. 2, about
whether the response applies the outcome. In this
case |Positive and |Negative are the basis states, so
we can write |Ψ = a|Positive + b|Negative
, where
“a” and “b” are amplitudes (coefficients) that reflect
the components of the state vector along the
different basis vectors. The answer to the question is
certain when the state vector |Ψ exactly coincides
with one basis vector. For instance if “the response
always applies the outcome”, then |Ψ = |Positive.
In such case the probability of Positive is 1. Since
the basis vectors are orthogonal, that is, since they
represent mutually exclusive answers, we know that
“the response removes the outcome” with 0
probability, corresponding to a 0 projection to the
subspace for Negative.
Figure 2: State space with the Applies subspace
(corresponding to the question whether response applies
outcome) and Positive-Negative basis vectors. The blue
vertical line represents the projection of |Ψ on |Positive.
To determine the probability of Positive we use a
projector, P
Positive
, which takes the vector |Ψ and
lays it down on the subspace spanned by |Positive,
that is, P
Positive
|Ψ = a|Positive. Then, the probability
that the response applies the outcome is equal to the
squared length of the projection, ||P
Positive
|Ψ||
2
. The
same applies to the probability associated with
b|Negative.
Posi ve
Nega ve
Ψ
(a)
QuantumProbabilityinOperantConditioning-BehavioralUncertaintyinReinforcementLearning
549